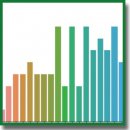
Prediction of Postoperative Speech Dysfunctions in Neurosurgery Based on Cortico-Cortical Evoked Potentials and Machine Learning Technology
Intraoperative recording of cortico-cortical evoked potentials (CCEPs) enables studying effective connections between various functional areas of the cerebral cortex. The fundamental possibility of postoperative speech dysfunction prediction in neurosurgery based on CCEP signal variations could serve as a basis to develop the criteria for the physiological permissibility of intracerebral tumors removal for maximum preservation of the patients’ quality of life.
The aim of the study was to test the possibility of predicting postoperative speech disorders in patients with glial brain tumors by using the CCEP data recorded intraoperatively before the stage of tumor resection.
Materials and Methods. CCEP data were reported for 26 patients. To predict the deterioration of speech functions in the postoperative period, we used four options for presenting CCEP data and several machine learning models: a random forest of decision trees, logistic regression, and support vector machine method with different types of kernels: linear, radial, and polynomial. Twenty variants of models were trained: each in 300 experiments with resampling. A total of 6000 tests were performed in the study.
Results. The prediction quality metrics for each model trained in 300 tests with resampling were averaged to eliminate the influence of “successful” and “unsuccessful” data grouping. The best result with F1-score = 0.638 was obtained by the support vector machine with a polynomial kernel. In most tests, a high sensitivity score was observed, and in the best model, it reached a value of 0.993; the specificity of the best model was 0.370.
Conclusion. This pilot study demonstrated the possibility of predicting speech dysfunctions based on CCEP data taken before the main stage of glial tumors resection; the data were processed using traditional machine learning methods. The best model with high sensitivity turned out to be insufficiently specific. Further studies will be aimed at assessing the changes in CCEP during the operation and their relationship with the development of postoperative speech deficit.
- Sporns O. The human connectome: a complex network. Ann N Y Acad Sci 2011; 1224: 109–125, https://doi.org/10.1111/j.1749-6632.2010.05888.x.
- Leisman G., Moustafa A.A., Shafir T. Thinking, walking, talking: integratory motor and cognitive brain function. Front Public Health 2016; 4: 94, https://doi.org/10.3389/fpubh.2016.00094.
- Kunieda T., Yamao Y., Kikuchi T., Matsumoto R. New approach for exploring cerebral functional connectivity: review of cortico-cortical evoked potential. Neurol Med Chir (Tokyo) 2015; 55(5): 374–382, https://doi.org/10.2176/nmc.ra.2014-0388.
- Matsumoto R., Nair D.R., LaPresto E., Bingaman W., Shibasaki H., Lüders H.O. Functional connectivity in human cortical motor system: a cortico-cortical evoked potential study. Brain 2007; 130(Pt 1): 181–197, https://doi.org/10.1093/brain/awl257.
- Bykanov A.E., Pitskhelauri D.I., Titov O.Y., Lin M.C., Gulaev E.V., Ogurtsova A.A., Maryashev S.A., Zhukov V.Y., Buklina S.B., Lubnin A.Y., Beshplav S.T., Konakova T.A., Pronin I.N. Broca’s area intraoperative mapping with cortico-cortical evoked potentials. Voprosy neirokhirurgii imeni N.N. Burdenko 2020; 84(6): 49–58, https://doi.org/10.17116/neiro20208406149.
- Yamao Y., Matsumoto R., Kikuchi T., Yoshida K., Kunieda T., Miyamoto S. Intraoperative brain mapping by cortico-cortical evoked potential. Front Hum Neurosci 2021; 15: 635453, https://doi.org/10.3389/fnhum.2021.635453.
- Tamura Y., Ogawa H., Kapeller C., Prueckl R., Takeuchi F., Anei R., Ritaccio A., Guger C., Kamada K. Passive language mapping combining real-time oscillation analysis with cortico-cortical evoked potentials for awake craniotomy. J Neurosurg 2016; 125(6): 1580–1588, https://doi.org/10.3171/2015.4.jns15193.
- Saito T., Tamura M., Muragaki Y., Maruyama T., Kubota Y., Fukuchi S., Nitta M., Chernov M., Okamoto S., Sugiyama K., Kurisu K., Sakai K.L., Okada Y., Iseki H. Intraoperative cortico-cortical evoked potentials for the evaluation of language function during brain tumor resection: initial experience with 13 cases. J Neurosurg 2014; 121(4): 827–838, https://doi.org/10.3171/2014.4.jns131195.
- Kubota Y., Enatsu R., Gonzalez-Martinez J., Bulacio J., Mosher J., Burgess R.C., Nair D.R. In vivo human hippocampal cingulate connectivity: a corticocortical evoked potentials (CCEPs) study. Clin Neurophysiol 2013; 124(8): 1547–1556, https://doi.org/10.1016/j.clinph.2013.01.024.
- Tielen A.M., Lopes da Silva F.H., Mollevanger W.J. Differential conduction velocities in perforant path fibres in guinea pig. Exp Brain Res 1981; 42(2): 231–233, https://doi.org/10.1007/bf00236913.
- Andersen P., Holmqvist B., Voorhoeve P.E. Excitatory synapses on hippocampal apical dendrites activated by entorhinal stimulation. Acta Physiol Scand 1966; 66(4): 461–472, https://doi.org/10.1111/j.1748-1716.1966.tb03224.x.
- Gloor P., Vera C.L., Sperti L. Electrophysiological studies of hippocampal neurons. III. Responses of hippocampal neurons to repetitive perforant path volleys. Electroencephalogr Clin Neurophysiol 1964; 17: 353–370, https://doi.org/10.1016/0013-4694(64)90158-0.
- Wilson C.L., Isokawa M., Babb T.L., Crandall P.H. Functional connections in the human temporal lobe. I. Analysis of limbic system pathways using neuronal responses evoked by electrical stimulation. Exp Brain Res 1990; 82(2): 279–292, https://doi.org/10.1007/bf00231248.
- Rutecki P.A., Grossman R.G., Armstrong D., Irish-Loewen S. Electrophysiological connections between the hippocampus and entorhinal cortex in patients with complex partial seizures. J Neurosurg 1989; 70(5): 667–675, https://doi.org/10.3171/jns.1989.70.5.0667.
- Matsumoto R., Nair D.R., LaPresto E., Najm I., Bingaman W., Shibasaki H., Lüders H.O. Functional connectivity in the human language system: a cortico-cortical evoked potential study. Brain 2004; 127(Pt 10): 2316–2330, https://doi.org/10.1093/brain/awh246.
- Howard M.A., Volkov I.O., Mirsky R., Garell P.C., Noh M.D., Granner M., Damasio H., Steinschneider M., Reale R.A., Hind J.E., Brugge J.F. Auditory cortex on the human posterior superior temporal gyrus. J Comp Neurol 2000; 416(1): 79–92, https://doi.org/10.1002/(sici)1096-9861(20000103)416:179::aid-cne63.0.co;2-2.
- Valentín A., Anderson M., Alarcón G., Seoane J.J., Selway R., Binnie C.D., Polkey C.E. Responses to single pulse electrical stimulation identify epileptogenesis in the human brain in vivo. Brain 2002; 125(Pt 8): 1709–1718, https://doi.org/10.1093/brain/awf187.
- Conner C.R., Ellmore T.M., DiSano M.A., Pieters T.A., Potter A.W., Tandon N. Anatomic and electro-physiologic connectivity of the language system: a combined DTI-CCEP study. Comput Biol Med 2011; 41(12): 1100–1109, https://doi.org/10.1016/j.compbiomed.2011.07.008.
- Silverstein B.H., Asano E., Sugiura A., Sonoda M., Lee M.H., Jeong J.W. Dynamic tractography: integrating cortico-cortical evoked potentials and diffusion imaging. Neuroimage 2020; 215: 116763, https://doi.org/10.1016/j.neuroimage.2020.116763.
- Mercier M., Bickel S., Megevand P., Groppe D., Mehta A. Intracranial recording: a glimpse on white-grey matter differences. Epilepsy Curr 2015; 15: 469.
- Golyandina N. Particularities and commonalities of singular spectrum analysis as a method of time series analysis and signal processing. Wiley Interdiscip Rev Comput Stat 2020; 12(4): e1487, https://doi.org/10.1002/wics.1487.
- Torrence C., Compo G.P. A practical guide to wavelet analysis. Bull Am Meteorol Soc 1998; 79(1): 61–78, https://doi.org/10.1175/1520-0477(1998)0790061:apgtwa2.0.co;2.
- Huang C.C., Chang C.S., Hsin Y.L. Time–frequency spectral analysis of cortico-cortical evoked potentials by means of Hilbert–Huang transform. Brain Stimul 2015; 8(2): 388, https://doi.org/10.1016/j.brs.2015.01.242.
- Prime D., Woolfe M., Rowlands D., O’Keefe S., Dionisio S. Comparing connectivity metrics in cortico-cortical evoked potentials using synthetic cortical response patterns. J Neurosci Methods 2020; 334: 108559, https://doi.org/10.1016/j.jneumeth.2019.108559.
- Christ M., Braun N., Neuffer J., Kempa-Liehr A.W. Time Series FeatuRe Extraction on basis of Scalable Hypothesis tests (tsfresh — a Python package). Neurocomputing 2018; 307: 72–77, https://doi.org/10.1016/j.neucom.2018.03.067.