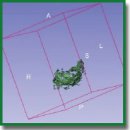
Radiomic Phenotype of Periatrial Adipose Tissue in the Prognosis of Late Postablation Recurrence of Idiopathic Atrial Fibrillation
The aim of the study is to find new predictors of postablation atrial fibrillation (AF) recurrence in patients with lone AF using a texture analysis of the periatrial adipose tissue (PAAT) of the left atrium.
Materials and Methods. Forty-three patients admitted for lone AF catheter ablation, who had undergone multispiral coronary angiography, were enrolled in the study. PAAT segmentation was performed using 3D Slicer application followed by extraction of 93 radiomic features. At the end of the follow-up period, patients were divided into 2 groups depending on the presence or absence of AF recurrence.
Results. 12 months of follow-up after catheter ablation, postablation AF recurrence was reported in 19 out of 43 patients. Of 93 extracted radiomic features of PAAT, statistically significant differences were observed for 3 features of the Gray Level Size Zone matrix. At the same time, only one radiomic feature of PAAT, Size Zone Non Uniformity Normalized, was an independent predictor of postablative recurrence of AF after catheter ablation and 12 months of follow-up (McFadden’s R2=0.451, OR — 0.506, 95% CI: 0.331‒0.776, p<0.001).
Conclusion. The radiomic analysis of periatrial adipose tissue may be considered as a promising non-invasive method for predicting adverse outcomes of the catheter treatment, which opens the possibilities for planning and correction of patient management tactics after intervention.
- Kirchhof P., Benussi S., Kotecha D., Ahlsson A., Atar D., Casadei B., Castella M., Diener H.C., Heidbuchel H., Hendriks J., Hindricks G., Manolis A.S., Oldgren J., Popescu B.A., Schotten U., Van Putte B., Vardas P.; ESC Scientific Document Group. 2016 ESC Guidelines for the management of atrial fibrillation developed in collaboration with EACTS. Eur Heart J 2016; 37(38): 2893–2962, https://doi.org/10.1093/eurheartj/ehw210.
- Sazonova S.I., Ilyushenkova Yu.N., Lishmanov Yu.B., Batalov R.E., Sazonov A.E., Larionova L.A., Nesterov E.A., Varlamova N.V., Rogovskaya Yu.V., Shelkovnikova T.A., Popov S.V. Assessment of radiological techniques application possibility for non-invasive diagnostics of latent inflammatory processes in myocardium in patients with atrial fibrillation. Ann Nucl Med 2016; 30(10): 738–748, https://doi.org/10.1007/s12149-016-1120-9.
- Hindricks G., Potpara T., Dagres N., Arbelo E., Bax J.J., Blomström-Lundqvist C., Boriani G., Castella M., Dan G.A., Dilaveris P.E., Fauchier L., Filippatos G., Kalman J.M., La Meir M., Lane D.A., Lebeau J.P., Lettino M., Lip G.Y.H., Pinto F.J., Thomas G.N., Valgimigli M., Van Gelder I.C., Van Putte B.P., Watkins C.L.; ESC Scientific Document Group. 2020 ESC Guidelines for the diagnosis and management of atrial fibrillation developed in collaboration with the European Association for Cardio-Thoracic Surgery (EACTS): The Task Force for the diagnosis and management of atrial fibrillation of the European Society of Cardiology (ESC). Developed with the special contribution of the European Heart Rhythm Association (EHRA) of the ESC. Eur Heart J 2021; 42(5): 373–498, https://doi.org/10.1093/eurheartj/ehaa612.
- Deng H., Bai Y., Shantsila A., Fauchier L., Potpara T.S., Lip G.Y.H. Clinical scores for outcomes of rhythm control or arrhythmia progression in patients with atrial fibrillation: a systematic review. Clin Res Cardiol 2017; 106(10): 813–823, https://doi.org/10.1007/s00392-017-1123-0.
- Gaborit B., Sengenes C., Ancel P., Jucquier A., Dutour A. Role of epicardial adipose tissue in health and disease: a matter of fat? Compr Physiol 2017; 7(3): 1051–1082, https://doi.org/10.1002/cphy.c160034.
- El Mahdiui M., Simon J., Smit J.M., Kuneman J.H., van Rosendael A.R., Steyerberg E.W., van der Geest R.J., Száraz L., Herczeg S., Szegedi N., Gellér L., Delgado V., Merkely B., Bax J.J., Maurovich-Horvat P. Posterior left atrial adipose tissue attenuation assessed by computed tomography and recurrence of atrial fibrillation after catheter ablation. Circ Arrhythm Electrophysiol 2021; 14(4): e009135, https://doi.org/10.1161/circep.120.009135.
- Sepehri Shamloo A., Schoene K., Stauber A., Darma A., Dagres N., Dinov B., Bertagnolli L., Hilbert S., Müssigbrodt A., Husser D., Bollmann A., Hindricks G., Arya A. Epicardial adipose tissue thickness as an independent predictor of ventricular tachycardia recurrence following ablation. Heart Rhythm 2019; 16(10): 1492–1498, https://doi.org/10.1016/j.hrthm.2019.06.009.
- Kocyigit D., Gurses K.M., Yalcin M.U., Turk G., Evranos B., Yorgun H., Sahiner M.L., Kaya E.B., Hazirolan T., Tokgozoglu L., Oto M.A., Ozer N., Aytemir K. Periatrial epicardial adipose tissue thickness is an independent predictor of atrial fibrillation recurrence after cryoballoon-based pulmonary vein isolation. J Cardiovasc Comput Tomogr 2015; 9(4): 295–302, https://doi.org/10.1016/j.jcct.2015.03.011.
- Ciuffo L., Nguyen H., Marques M.D., Aronis K.N., Sivasambu B., de Vasconcelos H.D., Tao S., Spragg D.D., Marine J.E., Berger R.D., Lima J.A.C., Calkins H., Ashikaga H. Periatrial fat quality predicts atrial fibrillation ablation outcome. Circ Cardiovasc Imaging 2019; 12(6): e008764, https://doi.org/10.1161/circimaging.118.008764.
- Avanzo M., Stancanello J., El Naqa I. Beyond imaging: the promise of radiomics. Phys Med 2017; 38: 122–139, https://doi.org/10.1016/j.ejmp.2017.05.071.
- Avery E., Sanelli P.C., Aboian M., Payabvash S. Radiomics: a primer on processing workflow and analysis. Semin Ultrasound CT MR 2022; 43(2): 142–146, https://doi.org/10.1053/j.sult.2022.02.003.
- Slart R.H.J.A., Williams M.C., Juarez-Orozco L.E., Rischpler C., Dweck M.R., Glaudemans A.W.J.M., Gimelli A., Georgoulias P., Gheysens O., Gaemperli O., Habib G., Hustinx R., Cosyns B., Verberne H.J., Hyafil F., Erba P.A., Lubberink M., Slomka P., Išgum I., Visvikis D., Kolossváry M., Saraste A. Position paper of the EACVI and EANM on artificial intelligence applications in multimodality cardiovascular imaging using SPECT/CT, PET/CT, and cardiac CT. Eur J Nucl Med Mol Imaging 2021; 48(5): 1399–1413, https://doi.org/10.1007/s00259-021-05341-z.
- Mahabadi A.A., Rassaf T. Radiomic assessment of pericoronary adipose tissue: detecting the vulnerable patient. JACC Cardiovasc Imaging 2020; 13(11): 2384–2385, https://doi.org/10.1016/j.jcmg.2020.07.006.
- Lin A., Kolossváry M., Išgum I., Maurovich-Horvat P., Slomka P.J., Dey D. Artificial intelligence: improving the efficiency of cardiovascular imaging. Expert Rev Med Devices 2020; 17(6): 565–577, https://doi.org/10.1080/17434440.2020.1777855.
- Ma Q., Ma Y., Wang X., Li S., Yu T., Duan W., Wu J., Wen Z., Jiao Y., Sun Z., Hou Y. A radiomic nomogram for prediction of major adverse cardiac events in ST-segment elevation myocardial infarction. Eur Radiol 2021; 31(2): 1140–1150, https://doi.org/10.1007/s00330-020-07176-y.
- Oikonomou E.K., Siddique M., Antoniades C. Artificial intelligence in medical imaging: a radiomic guide to precision phenotyping of cardiovascular disease. Cardiovasc Res 2020; 116(13): 2040–2054, https://doi.org/10.1093/cvr/cvaa021.
- Zhang L., Xu Z., Jiang B., Zhang Y., Wang L., de Bock G.H., Vliegenthart R., Xie X. Machine-learning-based radiomics identifies atrial fibrillation on the epicardial fat in contrast-enhanced and non-enhanced chest CT. Br J Radiol 2022; 95(1135): 20211274, https://doi.org/10.1259/bjr.20211274.
- Knuuti J., Wijns W., Saraste A., Capodanno D., Barbato E., Funck-Brentano C., Prescott E., Storey R.F., Deaton C., Cuisset T., Agewall S., Dickstein K., Edvardsen T., Escaned J., Gersh B.J., Svitil P., Gilard M., Hasdai D., Hatala R., Mahfoud F., Masip J., Muneretto C., Valgimigli M., Achenbach S., Bax J.J.; ESC Scientific Document Group. 2019 ESC Guidelines for the diagnosis and management of chronic coronary syndromes. Eur Heart J 2020; 41(3): 407–477, https://doi.org/10.1093/eurheartj/ehz425.
- Donal E., Lip G.Y., Galderisi M., Goette A., Shah D., Marwan M., Lederlin M., Mondillo S., Edvardsen T., Sitges M., Grapsa J., Garbi M., Senior R., Gimelli A., Potpara T.S., Van Gelder I.C., Gorenek B., Mabo P., Lancellotti P., Kuck K.H., Popescu B.A., Hindricks G., Habib G., Cardim N.M., Cosyns B., Delgado V., Haugaa K.H., Muraru D., Nieman K., Boriani G., Cohen A. EACVI/EHRA Expert Consensus Document on the role of multi-modality imaging for the evaluation of patients with atrial fibrillation. Eur Heart J Cardiovasc Imaging 2016; 17(4): 355–383, https://doi.org/10.1093/ehjci/jev354.
- Abbara S., Blanke P., Maroules C.D., Cheezum M., Choi A.D., Han B.K., Marwan M., Naoum C., Norgaard B.L., Rubinshtein R., Schoenhagen P., Villines T., Leipsic J. SCCT guidelines for the performance and acquisition of coronary computed tomographic angiography: a report of the society of Cardiovascular Computed Tomography Guidelines Committee: endorsed by the North American Society for Cardiovascular Imaging (NASCI). J Cardiovasc Comput Tomogr 2016; 10(6): 435–449, https://doi.org/10.1016/j.jcct.2016.10.002.
- Fedorov A., Beichel R., Kalpathy-Cramer J., Finet J., Fillion-Robin J.C., Pujol S., Bauer C., Jennings D., Fennessy F., Sonka M., Buatti J., Aylward S., Miller J.V., Pieper S., Kikinis R. 3D Slicer as an image computing platform for the Quantitative Imaging Network. Magn Reson Imaging 2012; 30(9): 1323–1341, https://doi.org/10.1016/j.mri.2012.05.001.
- Shelemehov A.E., Batalov R.E., Rogovskaya Y.V., Usenkov S.Y., Archakov E.A., Gusakova A.M., Rebenkova M.S. Clinical effectiveness of catheter treatment for atrial fibrillation depending on the dynamics of histological changes in the myocardium. Patologiya krovoobrashcheniya i kardiokhirurgiya 2020; 24(3): 90–106, https://doi.org/10.21688/1681-3472-2020-3-90-106.
- Koo T.K., Li M.Y. A guideline of selecting and reporting intraclass correlation coefficients for reliability research. J Chiropr Med 2016; 15(2): 155–163, https://doi.org/10.1016/j.jcm.2016.02.012.
- Yorgun H., Canpolat U., Aytemir K., Hazırolan T., Şahiner L., Kaya E.B., Kabakci G., Tokgözoğlu L., Özer N., Oto A. Association of epicardial and peri-atrial adiposity with the presence and severity of non-valvular atrial fibrillation. Int J Cardiovasc Imaging 2015; 31(3): 649–657, https://doi.org/10.1007/s10554-014-0579-5.
- van Rosendael A.R., Dimitriu-Leen A.C., van Rosendael P.J., Leung M., Smit J.M., Saraste A., Knuuti J., van der Geest R.J., van der Arend B.W., van Zwet E.W., Scholte A.J., Delgado V., Bax J.J. Association between posterior left atrial adipose tissue mass and atrial fibrillation. Circ Arrhythm Electrophysiol 2017; 10(2): e004614, https://doi.org/10.1161/circep.116.004614.
- Gaibazzi N., Martini C., Benatti G., Palumbo A.A., Cacciola G., Tuttolomondo D. Atrial fibrillation and peri-atrial inflammation measured through adipose tissue attenuation on cardiac computed tomography. Diagnostics (Basel) 2021; 11(11): 2087, https://doi.org/10.3390/diagnostics11112087.
- Liu Z., Wang S., Wang Y., Zhou N., Shu J., Stamm C., Jiang M., Luo F. Association of epicardial adipose tissue attenuation with coronary atherosclerosis in patients with a high risk of coronary artery disease. Atherosclerosis 2019; 284: 230–236, https://doi.org/10.1016/j.atherosclerosis.2019.01.033.
- Patel V.B., Mori J., McLean B.A., Basu R., Das S.K., Ramprasath T., Parajuli N., Penninger J.M., Grant M.B., Lopaschuk G.D., Oudit G.Y. ACE2 deficiency worsens epicardial adipose tissue inflammation and cardiac dysfunction in response to diet-induced obesity. Diabetes 2016; 65(1): 85–95, https://doi.org/10.2337/db15-0399.
- Gullaksen S., Funck K.L., Laugesen E., Hansen T.K., Dey D., Poulsen P.L. Volumes of coronary plaque disease in relation to body mass index, waist circumference, truncal fat mass and epicardial adipose tissue in patients with type 2 diabetes mellitus and controls. Diab Vasc Dis Res 2019; 16(4): 328–336, https://doi.org/10.1177/1479164119825761.
- Berenguer R., Pastor-Juan M.D.R., Canales-Vázquez J., Castro-García M., Villas M.V., Mansilla Legorburo F., Sabater S. Radiomics of CT features may be nonreproducible and redundant: influence of CT acquisition parameters. Radiology 2018; 288(2): 407–415, https://doi.org/10.1148/radiol.2018172361.
- Meyer M., Ronald J., Vernuccio F., Nelson R.C., Ramirez-Giraldo J.C., Solomon J., Patel B.N., Samei E., Marin D. Reproducibility of CT radiomic features within the same patient: influence of radiation dose and CT reconstruction settings. Radiology 2019; 293(3): 583–591, https://doi.org/10.1148/radiol.2019190928.
- Agatston A.S., Janowitz W.R. Ultrafast computed tomography in coronary screening. Circulation 1994; 89(4): 1908–1909, https://doi.org/10.1161/01.cir.89.4.1908.
- van Griethuysen J.J.M., Fedorov A., Parmar C., Hosny A., Aucoin N., Narayan V., Beets-Tan R.G.H., Fillion-Robin J.C., Pieper S., Aerts H.J.W.L. Computational radiomics system to decode the radiographic phenotype. Cancer Res 2017; 77(21): e104–e107, https://doi.org/10.1158/0008-5472.can-17-0339.
- Umesh Pai M., Ardakani A.A., Kamath A., Raghavendra U., Gudigar A., Venkatesh N., Samanth J., Devasia T., Prabhu M.A., Sampathila N., Bairy G.M. Novel radiomics features for automated detection of cardiac abnormality in patients with pacemaker. Comput Math Methods Med 2022; 2022: 1279749, https://doi.org/10.1155/2022/1279749.