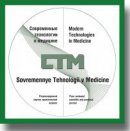
Identification of Diagnostic Schizophrenia Biomarkers Based on the Assessment of Immune and Systemic Inflammation Parameters Using Machine Learning Modeling
Disorders of systemic immunity and immune processes in the brain have now been shown to play an essential role in the development and progression of schizophrenia. Nevertheless, only a few works were devoted to the study of some immune parameters to objectify the diagnosis by means of machine learning. At the same time, machine learning methods have not yet been applied to a set of data fully reflecting systemic characteristics of the immune status (parameters of adaptive immunity, the level of inflammatory markers, the content of major cytokines). Considering a complex nature of immune system disorders in schizophrenia, incorporation of a broad panel of immunological data into machine learning models is promising for improving classification accuracy and identifying the parameters reflecting the immune disorders typical for the majority of patients.
The aim of the study is to assess the possibility of using immunological parameters to objectify the diagnosis of schizophrenia applying machine learning models.
Materials and Methods. We have analyzed 17 immunological parameters in 63 schizophrenia patients and 36 healthy volunteers. The parameters of humoral immunity, systemic level of the key cytokines of adaptive immunity, anti-inflammatory and pro-inflammatory cytokines, and other inflammatory markers were determined by enzyme immunoassay. Applied methods of machine learning covered the main group of approaches to supervised learning such as linear models (logistic regression), quadratic discriminant analysis (QDA), support vector machine (linear SVM, RBF SVM), k-nearest neighbors algorithm, Gaussian processes, naive Bayes classifier, decision trees, and ensemble models (AdaBoost, random forest, XGBoost). The importance of features for prediction from the best fold has been analyzed for the machine learning methods, which demonstrated the best quality. The most significant features were selected using 70% quantile threshold.
Results. The AdaBoost ensemble model with ROC AUC of 0.71±0.15 and average accuracy (ACC) of 0.78±0.11 has demonstrated the best quality on a 10-fold cross validation test sample. Within the frameworks of the present investigation, the AdaBoost model has shown a good quality of classification between the patients with schizophrenia and healthy volunteers (ROC AUC over 0.70) at a high stability of the results (σ less than 0.2). The most important immunological parameters have been established for differentiation between the patients and healthy volunteers: the level of some systemic inflammatory markers, activation of humoral immunity, pro-inflammatory cytokines, immunoregulatory cytokines and proteins, Th1 and Th2 immunity cytokines. It was for the first time that the possibility of differentiating schizophrenia patients from healthy volunteers was shown with the accuracy of more than 70% with the help of machine learning using only immune parameters.
The results of this investigation confirm a high importance of the immune system in the pathogenesis of schizophrenia.
- Jääskeläinen E., Juola P., Hirvonen N., McGrath J.J., Saha S., Isohanni M., Veijola J., Miettunen J. A systematic review and meta-analysis of recovery in schizophrenia. Schizophr Bull 2013; 39(6): 1296–1306, https://doi.org/10.1093/schbul/sbs130.
- Nordentoft M., Wahlbeck K., Hällgren J., Westman J., Osby U., Alinaghizadeh H., Gissler M., Laursen T.M. Excess mortality, causes of death and life expectancy in 270,770 patients with recent onset of mental disorders in Denmark, Finland and Sweden. PLoS One 2013; 8(1): e55176, https://doi.org/10.1371/journal.pone.0055176.
- Correll C.U., Galling B., Pawar A., Krivko A., Bonetto C., Ruggeri M., Craig T.J., Nordentoft M., Srihari V.H., Guloksuz S., Hui C.L.M., Chen E.Y.H., Valencia M., Juarez F., Robinson D.G., Schooler N.R., Brunette M.F., Mueser K.T., Rosenheck R.A., Marcy P., Addington J., Estroff S.E., Robinson J., Penn D., Severe J.B., Kane J.M. Comparison of early intervention services vs treatment as usual for early-phase psychosis: a systematic review, meta-analysis, and meta-regression. JAMA Psychiatry 2018; 75(6): 555–565, https://doi.org/10.1001/jamapsychiatry.2018.0623.
- Ferrara M., Guloksuz S., Mathis W.S., Li F., Lin I.H., Syed S., Gallagher K., Shah J., Kline E., Tek C., Keshavan M., Srihari V.H. First help-seeking attempt before and after psychosis onset: measures of delay and aversive pathways to care. Soc Psychiatry Psychiatr Epidemiol 2021; 56(8): 1359–1369, https://doi.org/10.1007/s00127-021-02090-0.
- Mirzakhanian H., Singh F., Cadenhead K.S. Biomarkers in psychosis: an approach to early identification and individualized treatment. Biomark Med 2014; 8(1): 51–57, https://doi.org/10.2217/bmm.13.134.
- Ellis J.K., Walker E.F., Goldsmith D.R. Selective review of neuroimaging findings in youth at clinical high risk for psychosis: on the path to biomarkers for conversion. Front Psychiatry 2020; 11: 567534, https://doi.org/10.3389/fpsyt.2020.567534.
- van Mierlo H.C., Schot A., Boks M.P.M., de Witte L.D. The association between schizophrenia and the immune system: review of the evidence from unbiased ‘omic-studies’. Schizophr Res 2020; 217: 114–123, https://doi.org/10.1016/j.schres.2019.05.028.
- Birnbaum R., Weinberger D.R. A genetics perspective on the role of the (neuro)immune system in schizophrenia. Schizophr Res 2020; 217: 105–113, https://doi.org/10.1016/j.schres.2019.02.005.
- Sun H.L., Bai W., Li X.H., Huang H., Cui X.L., Cheung T., Su Z.H., Yuan Z., Ng C.H., Xiang Y.T. Schizophrenia and inflammation research: a bibliometric analysis. Front Immunol 2022; 13: 907851, https://doi.org/10.3389/fimmu.2022.907851.
- Fernandes B.S., Karmakar C., Tamouza R., Tran T., Yearwood J., Hamdani N., Laouamri H., Richard J.R., Yolken R., Berk M., Venkatesh S., Leboyer M. Precision psychiatry with immunological and cognitive biomarkers: a multi-domain prediction for the diagnosis of bipolar disorder or schizophrenia using machine learning. Transl Psychiatry 2020; 10(1): 162, https://doi.org/10.1038/s41398-020-0836-4.
- Ferrara M., Franchini G., Funaro M., Cutroni M., Valier B., Toffanin T., Palagini L., Zerbinati L., Folesani F., Murri M.B., Caruso R., Grassi L. Machine learning and non-affective psychosis: identification, differential diagnosis, and treatment. Curr Psychiatry Rep 2022; 24(12): 925–936, https://doi.org/10.1007/s11920-022-01399-0.
- Sharaev M.G., Malashenkova I.K., Maslennikova A.V., Zakharova N.V., Bernstein A.V., Burnaev E.V., Mamedova G.S., Krynskiy S.A., Ogurtsov D.P., Kondrateva E.A., Druzhinina P.V., Zubrikhina M.O., Arkhipov A.Yu., Strelets V.B., Ushakov V.L. Diagnosis of schizophrenia based on the data of various modalities: biomarkers and machine learning techniques (review). Sovremennye tehnologii v medicine 2022; 14(5): 53, https://doi.org/10.17691/stm2022.14.5.06.
- Malashenkova I.K., Krynskiy S.A., Ogurtsov D.P., Mamoshina M.V., Zakharova N.V., Ushakov V.L., Velichkovsky B.M., Didkovsky N.A. A role of the immune system in the pathogenesis of schizophrenia. Zhurnal nevrologii i psihiatrii im. S.S. Korsakova 2018; 118(12): 72–80, https://doi.org/10.17116/jnevro201811812172.
- Corponi F., Zorkina Y., Stahl D., Murru A., Vieta E., Serretti A., Morozova A., Reznik A., Kostyuk G., Chekhonin V.P. Frontal lobes dysfunction across clinical clusters of acute schizophrenia. Rev Psiquiatr Salud Ment 2021, https://doi.org/10.1016/j.rpsm.2021.12.002.
- Morozova A., Zorkina Y., Abramova O., Pavlova O., Pavlov K., Soloveva K., Volkova M., Alekseeva P., Andryshchenko A., Kostyuk G., Gurina O., Chekhonin V. Neurobiological highlights of cognitive impairment in psychiatric disorders. Int J Mol Sci 2022; 23(3): 1217, https://doi.org/10.3390/ijms23031217.
- Kartashov S.I., Vartanov A.V., Zakharova N.V., Arkhipov A.Yu., Strelets V.B., Maslennikova A.V., Ushakov V.L. Tractographic studies in the human brain at the norm and the paranoid schizophrenia. Procedia Comput Sci 2021; 190: 434–438, https://doi.org/10.1016/j.procs.2021.06.051.
- Kay S.R., Fiszbein A., Opler L.A. The positive and negative syndrome scale (PANSS) for schizophrenia. Schizophr Bull 1987; 13(2): 261–276, https://doi.org/10.1093/schbul/13.2.261.
- Goldsmith D.R., Rapaport M.H., Miller B.J. A meta-analysis of blood cytokine network alterations in psychiatric patients: comparisons between schizophrenia, bipolar disorder and depression. Mol Psychiatry 2016; 21(12): 1696–1709, https://doi.org/10.1038/mp.2016.3.
- Malashenkova I.K., Krynskiy S.A., Ogurtsov D.P., Hailov N.A., Zakharova N.V., Bravve L.V., Kaydan M.A., Chekulaeva E.I., Andreyuk D.S., Ushakov V.L., Didkovsky N.A., Kostyuk G.P. Immunoinflammatory profile in patients with episodic and continuous paranoid schizophrenia. Consortium Psychiatricum 2021; 2(1): 19–31, https://doi.org/10.17816/cp66.
- Ushakov V.L., Malashenkova I.K., Kostyuk G.P., Zakharova N.V., Krynskiy S.A., Kartashov S.I., Ogurtsov D.P., Bravve L.V., Kaydan M.A., Hailov N.A., Chekulaeva E.I., Didkovsky N.A. The relationship between inflammation, cognitive impairments, and neuroimaging data in schizophrenia. Neurosci Behav Physiol 2021; 51(7): 873–881.
- Johnsen E., Fathian F., Kroken R.A., Steen V.M., Jørgensen H.A., Gjestad R., Løberg E.M. The serum level of C-reactive protein (CRP) is associated with cognitive performance in acute phase psychosis. BMC Psychiatry 2016; 16: 60, https://doi.org/10.1186/s12888-016-0769-x.
- Zhang Q., He H., Cao B., Gao R., Jiang L., Zhang X., Dai J. Analysis of cognitive impairment in schizophrenia based on machine learning: Interaction between psychological stress and immune system. Neurosci Lett 2021; 760: 136084, https://doi.org/10.1016/j.neulet.2021.136084.
- Al-Hakeim H.K., Almulla A.F., Al-Dujaili A.H., Maes M. Construction of a neuro-immune-cognitive pathway-phenotype underpinning the phenome of deficit schizophrenia. Curr Top Med Chem 2020; 20(9): 747–758, https://doi.org/10.2174/1568026620666200128143948.
- Tanaka T., Narazaki M., Kishimoto T. IL-6 in inflammation, immunity, and disease. Cold Spring Harb Perspect Biol 2014; 6(10): a016295, https://doi.org/10.1101/cshperspect.a016295.
- Malashenkova I.K., Krynskiy S.A., Khailov N.A., Kazanova G.V., Velichkovsky B.B., Didkovsky N.A. The role of cytokines in memory consolidation. Uspekhi sovremennoi biologii 2015; 135(5): 419–436.
- Malashenkova I.K., Ushakov V.L., Zakharova N.V., Krynskiy S.A., Ogurtsov D.P., Hailov N.A., Chekulaeva E.I., Ratushnyy A.Y., Kartashov S.I., Kostyuk G.P., Didkovsky N.A. Neuro-immune aspects of schizophrenia with severe negative symptoms: new diagnostic markers of disease phenotype. Sovremennye tehnologii v medicine 2021; 13(6): 24, https://doi.org/10.17691/stm2021.13.6.03.
- Momtazmanesh S., Zare-Shahabadi A., Rezaei N. Cytokine alterations in schizophrenia: an updated review. Front Psychiatry 2019; 10: 892, https://doi.org/10.3389/fpsyt.2019.00892.
- Chiang S.S.W., Riedel M., Schwarz M., Mueller N. Is T-helper type 2 shift schizophrenia-specific? Primary results from a comparison of related psychiatric disorders and healthy controls. Psychiatry Clin Neurosci 2013; 67(4): 228–236, https://doi.org/10.1111/pcn.12040.
- de Campos-Carli S.M., Miranda A.S., Dias I.C., de Oliveira A., Cruz B.F., Vieira É.L., Rocha N.P., Barbosa I.G., Salgado J.V., Teixeira A.L. Serum levels of interleukin-33 and its soluble form receptor (sST2) are associated with cognitive performance in patients with schizophrenia. Compr Psychiatry 2017; 74: 96–101, https://doi.org/10.1016/j.comppsych.2017.01.008.
- Musso T., Gusella G.L., Brooks A., Longo D.L., Varesio L. Interleukin-4 inhibits indoleamine 2,3-dioxygenase expression in human monocytes. Blood 1994; 83(5): 1408–1411, https://doi.org/10.1182/blood.V83.5.1408.1408.