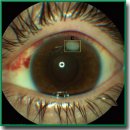
Application of Deep Learning Algorithms Based on the Multilayer YOLOv8 Neural Network to Identify Fungal Keratitis
The aim of the study is to develop a method for diagnosing fungal keratitis based on the analysis of photographs of the anterior segment of the eye using deep learning algorithms with subsequent evaluation of sensitivity and specificity of the method on a test data set in comparison with the results of practicing ophthalmologists.
Materials and Methods. The study has included the stages of data acquisition, image pre-training and markup, selection of training approach and neural network architecture, training with input data augmentation, validation with hyperparameter correction, evaluation of algorithm performance on a test sample, and determination of sensitivity and specificity of fungal keratitis detection by practicing doctors. A total of 274 anterior segment images were used, including 130 photographs of the eyes affected by fungal keratitis and 144 photographs illustrating normal eyes, keratitis of other etiologies, and various anterior segment pathologies. Photographs taken after the treatment onset, illustrations of keratitis of mixed etiology and corneal perforation were excluded from the study. Images of the training sample were marked up using the VGG Image Annotator web application and then used to train the YOLOv8 convolutional neural network. Images from the test data set were also offered to practicing ophthalmologists to determine the diagnostic accuracy of fungal keratitis.
Results. The sensitivity of the model was 56.0%, the specificity level reached 96.1%, and the proportion of correct answers of the algorithm was 76.5%. The accuracy of image recognition by practicing ophthalmologists was 50.0%, specificity — 41.7%, sensitivity — 57.7%.
Conclusion. The study showed the high potential of deep learning algorithms in the diagnosis of fungal keratitis and its advantages in accuracy compared to expert judgment in the absence of metadata. The use of computer vision technologies may find application as a complementary diagnostic method in decision making in complex cases and in telemedicine care settings. Further research is required to compare the developed model with alternative approaches, to expand and standardize databases.
- Aprelev A.E., Mankibaeva R.I., Mankibaev B.S., Apreleva E.V. The use of systems with artificial intelligence in the diagnosis of ophthalmic diseases. Vestnik Bashkirskogo gosudarstvennogo medicinskogo universiteta 2019; 3: 10–14.
- Sharma M., Jain N., Ranganathan S., Sharma N., Honavar S.G., Sharma N., Sachdev M.S. Tele-ophthalmology: need of the hour. Indian J Ophthalmol 2020; 68(7): 1328–1338, https://doi.org/10.4103/ijo.IJO_1784_20.
- Gliznitsa P.V., Takhchidi Kh.P., Svetozarskiy S.N., Bursov A.I., Shusterzon K.A. Machine learning in the diagnosis and treatment of ophthalmic diseases. Head and neck. Russian Journal 2022; 10(1): 83–90, https://doi.org/10.25792/HN.2022.10.1.83-90.
- Litjens G., Kooi T., Bejnordi B.E., Setio A.A.A., Ciompi F., Ghafoorian M., van der Laak J.A.W.M., van Ginneken B., Sánchez C.I. A survey on deep learning in medical image analysis. Med Image Anal 2017; 42: 60–88, https://doi.org/10.1016/j.media.2017.07.005.
- LeCun Y., Bengio Y., Hinton G. Deep learning. Nature 2015; 521(7553): 436–444, https://doi.org/10.1038/nature14539.
- Garri D.D., Saakyan S.V., Khoroshilova-Maslova I.P., Tsygankov A.Yu., Nikitin O.I., Tarasov G.Yu. Methods of machine learning in ophthalmology: review. Oftal’mologia 2020; 17(1): 20–31, https://doi.org/10.18008/1816-5095-2020-1-20-31.
- Ursuleanu T.F., Luca A.R., Gheorghe L., Grigorovici R., Iancu S., Hlusneac M., Preda C., Grigorovici A. Deep learning application for analyzing of constituents and their correlations in the interpretations of medical images. Diagnostics (Basel) 2021; 11(8): 1373, https://doi.org/10.3390/diagnostics11081373.
- Wang E.Y., Kong X., Wolle M., Gasquet N., Ssekasanvu J., Mariotti S.P., Bourne R., Taylor H., Resnikoff S., West S. Global trends in blindness and vision impairment resulting from corneal opacity 1984–2020: a meta-analysis. Ophthalmology 2023; 130(8): 863–871, https://doi.org/10.1016/j.ophtha.2023.03.012.
- Cabrera-Aguas M., Khoo P., Watson S.L. Infectious keratitis: a review. Clin Exp Ophthalmol 2022; 50(5): 543–562, https://doi.org/10.1111/ceo.14113.
- Sitnova A.V., Svetozarskiy S.N. Modern technologies in diagnosis of fungal keratitis (review). Sovremennye tehnologii v medicine 2023; 15(2): 73, https://doi.org/10.17691/stm2023.15.2.07.
- Brown L., Leck A.K., Gichangi M., Burton M.J., Denning D.W. The global incidence and diagnosis of fungal keratitis. Lancet Infect Dis 2021; 21(3): e49–e57, https://doi.org/10.1016/S1473-3099(20)30448-5.
- Hoffman J.J., Burton M.J., Leck A. Mycotic keratitis — a global threat from the filamentous fungi. J Fungi (Basel) 2021; 7(4): 273, https://doi.org/10.3390/jof7040273.
- Brown L., Kamwiziku G., Oladele R.O., Burton M.J., Prajna N.V., Leitman T.M., Denning D.W. The case for fungal keratitis to be accepted as a neglected tropical disease. J Fungi (Basel) 2022; 8(10): 1047, https://doi.org/10.3390/jof8101047.
- Whitcher J.P., Srinivasan M., Upadhyay M.P. Prevention of corneal ulceration in the developing world. Int Ophthalmol Clin 2002; 42(1): 71–77, https://doi.org/10.1097/00004397-200201000-00010.
- Ung L., Bispo P.J.M., Shanbhag S.S., Gilmore M.S., Chodosh J. The persistent dilemma of microbial keratitis: global burden, diagnosis, and antimicrobial resistance. Surv Ophthalmol 2019; 64(3): 255–271, https://doi.org/10.1016/j.survophthal.2018.12.003.
- Deorukhkar S., Katiyar R., Saini S. Epidemiological features and laboratory results of bacterial and fungal keratitis: a five-year study at a rural tertiary-care hospital in western Maharashtra, India. Singapore Med J 2012; 53(4): 264–267.
- Svetozarskiy S.N., Andreev A.N., Shcherbakova S.V. Fungal keratitis after penetrating keratoplasty. Vestnik oftal’mologii 2019; 135(4): 98–102, https://doi.org/10.17116/oftalma201913504198.
- Shao Y., Jie Y., Liu Z.G.; Expert Workgroup of Guidelines for the application of artificial intelligence in the diagnosis of anterior segment diseases (2023); Ophthalmic Imaging and Intelligent Medicine Branch of Chinese Medicine Education Association; Ophthalmology Committee of International Association of Translational Medicine; Chinese Ophthalmic Imaging Study Groups. Guidelines for the application of artificial intelligence in the diagnosis of anterior segment diseases (2023). Int J Ophthalmol 2023; 16(9): 1373–1385, https://doi.org/10.18240/ijo.2023.09.03.
- Redmon J., Divvala S., Girshick R., Farhadi A. You only look once: unified, real-time object detection. Proceedings of the IEEE Conference on Computer Vision and Pattern Recognition (CVPR) 2016; 779–788, https://doi.org/10.1109/CVPR.2016.91.
- Bochkovskiy A., Wang C.-Y., Liao Н.-Y.M. YOLOv4: optimal speed and accuracy of object detection. ArXiv 2020, https://doi.org/10.48550/arXiv.2004.10934.
- Breht E.A., Konshina V.N. Application of YOLO neural network for defect recognition. Intellektual’nye tehnologii na transporte 2022; 2(30): 41–47.
- Xu Y., Kong M., Xie W., Duan R., Fang Z., Lin Y., Zhu Q., Tang S., Wu F., Yao Y.-F. Deep sequential feature learning in clinical image classification of infectious keratitis. Engineering 2021; 7(7): 1002–1010, https://doi.org/10.1016/j.eng.2020.04.012.
- Dalmon C., Porco T.C., Lietman T.M., Prajna N.V., Prajna L., Das M.R., Kumar J.A., Mascarenhas J., Margolis T.P., Whitcher J.P., Jeng B.H., Keenan J.D., Chan M.F., McLeod S.D., Acharya N.R. The clinical differentiation of bacterial and fungal keratitis: a photographic survey. Invest Ophthalmol Vis Sci 2012; 53(4): 1787–1791, https://doi.org/10.1167/iovs.11-8478.
- Kuo M.T., Hsu B.W., Yin Y.K., Fang P.C., Lai H.Y., Chen A., Yu M.S., Tseng V.S. A deep learning approach in diagnosing fungal keratitis based on corneal photographs. Sci Rep 2020; 10(1): 14424, https://doi.org/10.1038/s41598-020-71425-9.
- Hu S., Sun Y., Li J., Xu P., Xu M., Zhou Y., Wang Y., Wang S., Ye J. Automatic diagnosis of infectious keratitis based on slit lamp images analysis. J Pers Med 2023; 13(3): 519, https://doi.org/10.3390/jpm13030519.
- Soleimani M., Esmaili K., Rahdar A., Aminizadeh M., Cheraqpour K., Tabatabaei S.A., Mirshahi R., Bibak Z., Mohammadi S.F., Koganti R., Yousefi S., Djalilian A.R. From the diagnosis of infectious keratitis to discriminating fungal subtypes; a deep learning-based study. Sci Rep 2023; 13(1): 22200, https://doi.org/10.1038/s41598-023-49635-8.
- Koyama A., Miyazaki D., Nakagawa Y., Ayatsuka Y., Miyake H., Ehara F., Sasaki S.I., Shimizu Y., Inoue Y. Determination of probability of causative pathogen in infectious keratitis using deep learning algorithm of slit-lamp images. Sci Rep 2021; 11(1): 22642, https://doi.org/10.1038/s41598-021-02138-w.
- Tiwari M., Piech C., Baitemirova M., Prajna N.V., Srinivasan M., Lalitha P., Villegas N., Balachandar N., Chua J.T., Redd T., Lietman T.M., Thrun S., Lin C.C. Differentiation of active corneal infections from healed scars using deep learning. Ophthalmology 2022; 129(2): 139–146, https://doi.org/10.1016/j.ophtha.2021.07.033.
- Ghosh A.K., Thammasudjarit R., Jongkhajornpong P., Attia J., Thakkinstian A. Deep learning for discrimination between fungal keratitis and bacterial keratitis: deepkeratitis. Cornea 2022; 41(5): 616–622, https://doi.org/10.1097/ICO.0000000000002830.
- Hanif A., Prajna N.V., Lalitha P., NaPier E., Parker M., Steinkamp P., Keenan J.D., Campbell J.P., Song X., Redd T.K. Assessing the impact of image quality on deep learning classification of infectious keratitis. Ophthalmol Sci 2023; 3(4): 100331, https://doi.org/10.1016/j.xops.2023.100331.
- Takhchidi K.P., Gliznitsa P.V., Svetozarskiy S.N., Bursov A.I., Shusterzon K.A. Labelling of data on fundus color pictures used to train a deep learning model enhances its macular pathology recognition capabilities. Bulletin of Russian State Medical University 2021; (4): 28–33, https://doi.org/10.24075/brsmu.2021.040.
- Li D.J., Huang B.L., Peng Y. Comparisons of artificial intelligence algorithms in automatic segmentation for fungal keratitis diagnosis by anterior segment images. Front Neurosci 2023; 17: 1195188, https://doi.org/10.3389/fnins.2023.1195188.
- Redd T.K., Santina L.D., Prajna N.V., Lalitha P., Acharya N., Lietman T. Automated differentiation of bacterial from fungal keratitis using deep learning. Invest Ophthalmol Vis Sci 2021; 62(8): 2161.
- Hung N., Shih A.K.-Y., Lin C., Kuo M.-T., Hwang Y.-S., Wu W.-C., Kuo C.-F., Kang E.Y.-C., Hsiao C.-H. Using slit-lamp images for deep learning-based identification of bacterial and fungal keratitis: model development and validation with different convolutional neural networks. Diagnostics 2021; 11(7): 1246, https://doi.org/10.3390/diagnostics11071246.
- Kuo M.T., Hsu B.W., Lin Y.S., Fang P.C., Yu H.J., Chen A., Yu M.S., Tseng V.S. Comparisons of deep learning algorithms for diagnosing bacterial keratitis via external eye photographs. Sci Rep 2021; 11(1): 24227, https://doi.org/10.1038/s41598-021-03572-6.
- Gu H., Guo Y., Gu L., Wei A., Xie S., Ye Z., Xu J., Zhou X., Lu Y., Liu X., Hong J. Deep learning for identifying corneal diseases from ocular surface slit-lamp photographs. Sci Rep 2020; 10(1): 17851, https://doi.org/10.1038/s41598-020-75027-3.
- Essalat M., Abolhosseini M., Le T.H., Moshtaghion S.M., Kanavi M.R. Interpretable deep learning for diagnosis of fungal and acanthamoeba keratitis using in vivo confocal microscopy images. Sci Rep 2023; 13(1): 8953, https://doi.org/10.1038/s41598-023-35085-9.
- Krutikov V.K., Kosogorova L.A., Yakunina M.V., Yakunina V.A., Sharov S.V. Standards for the use of artificial intelligence in domestic medicine. Ekonomika i predprinimatelstvo 2022; 3(140): 1184–1186.