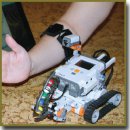
Combined Use of Command-Proportional Control of External Robotic Devices Based on Electromyography Signals
The aim of the investigation was to develop a control system combining command and proportional control of robotic devices based on electromyography (EMG) signals.
Materials and Methods. EMG signals were recorded using 8-channel bracelet MYO Thalmic (Thalmic Labs, Canada). Command control of robotic devices was exercised by EMG patterns associated with 6 static hand gestures. The patterns were classified by periodic calculation of a root mean square value of an EMG signal for all channels with further recognition by a two-layer neural network based on back propagation algorithm. Proportional control was performed by calculating the mean absolute value of an EMG signal, and command execution speed adjustment proportional to this value. The software of the control unit was connected via wireless Bluetooth interface with a mobile robot assembled from a set of LEGO NXT Mindstorms (LEGO, Denmark).
Results. We presented a soft and hardware platform combining command and proportional control of robotic devices based on EMG signals, and determined the parameters providing optimal characteristics of classification accuracy of EMG patterns, as well as the speed and accuracy of proportional control. We put forward and studied the following schemes combining command and proportional control: 1) the use of independent channels of different control types with recording EMG signals from both hands, 2) the use of independent channels of different control types with recording EMG signals from one hand only, 3) the use of all channels recording an EMG signal from one hand for classification and dynamic selection of a channel for proportional control, and 4) the use of all channels recording an EMG signal from one hand for classification with an average signal across all channels for proportional control.
Conclusion. We proposed a novel system of combined command-proportional control of robotic devices based on the neuromuscular activity signals. We studies several schemes and chose the most preferable (Scheme 4) one, and found the optimal parameters for command classification accuracy, as well as speed and accuracy of proportional control.
- Myo™ Gesture Control Armband — Wearable Technology by Thalmic Labs. URL: https://www.myo.com/.
- Delsys® Trigno™ Wireless systems and smart sensors. URL: http://www.delsys.com/products/wireless-emg/.
- Chowdhury R.H., Reaz M.B., Ali M.A., Bakar A.A., Chellappan K., Chang T.G. Surface electromyography signal processing and classification techniques. Sensors 2013; 13(9): 12431–12466, http://dx.doi.org/10.3390/s130912431.
- Bortole M., Venkatakrishnan A., Zhu F., Moreno J.C., Francisco G.E., Pons J.L., Contreras-Vidal J.L. The H2 robotic exoskeleton for gait rehabilitation after stroke: early findings from a clinical study. J Neuroeng Rehabil 2015; 12: 54, http://dx.doi.org/10.1186/s12984-015-0048-y.
- Vorobyev A.A., Petrukhin A.V., Zasypkina O.A., Krivonozhkina P.S., Pozdnyakov A.M. Exoskeleton as a new means in habilitation and rehabilitation of invalids (review). Sovremennye tehnologii v medicine 2015; 7(2): 185–197, http://dx.doi.org/10.17691/stm2015.7.2.22.
- Mehrholz J., Pohl M. Electromechanical-assisted gait training after stroke: a systematic review comparing end-effector and exoskeleton devices. J Rehabil Med 2012; 44(3): 193–199, http://dx.doi.org/10.2340/16501977-0943.
- Singh R.M., Chatterji S., Kumar A. Trends and challenges in EMG based control scheme of exoskeleton robots — a review. International Journal of Scienctific and Engineering Research 2012; 3(8): 1–8.
- Lyons K.R., Joshi S.S. A case study on classification of foot gestures via surface electromyography. In: Annu. Conf. Rehabil. Eng. Assist. Technol. Soc. Am. (RESNA). Denver, USA; 2015.
- Aszmann O.C., Roche A.D., Salminger S., Paternostro-Sluga T., Herceg M., Sturma A., Hofer C., Farina D. Bionic reconstruction to restore hand function after brachial plexus injury: a case series of three patients. Lancet 2015; 385(9983): 2183–2189, http://dx.doi.org/10.1016/S0140-6736(14)61776-1.
- Fougner A., Stavdahl O., Kyberd P.J., Losier Y.G., Parker P.A. Control of upper limb prostheses: terminology and proportional myoelectric control — a review. IEEE Trans Neural Syst Rehabil Eng 2012; 20(5): 663–677, http://dx.doi.org/10.1109/TNSRE.2012.2196711.
- Roche A.D., Rehbaum H., Farina D., Aszmann O.C. Prosthetic myoelectric control strategies: a clinical perspective. Curr Surg Rep 2014; 2: 44, http://dx.doi.org/10.1007/s40137-013-0044-8.
- Ison M., Artemiadis P. Proportional myoelectric control of robots: muscle synergy development drives performance enhancement, retainment, and generalization. IEEE Trans Robot 2015; 31(2): 259–268, http://dx.doi.org/10.1109/TRO.2015.2395731.
- Ison M., Vujaklija I., Whitsell B., Farina D., Artemiadis P. High-density electromyography and motor skill learning for robust long-term control of a 7-DoF robot arm. IEEE Trans Neur Sys Rehabil Eng 2015; 99: 1, http://dx.doi.org/10.1109/TNSRE.2015.2417775.
- Hahne J.M., Rehbaum H., Biessmann F., Meinecke F.C., Muller K.-R., Jiang N., Farina D., Parra L.C. Simultaneous and proportional control of 2D wrist movements with myoelectric signals. In: IEEE International Workshop on Machine Learning for Signal Processing 2012. Santander, Spain; 2012; p. 1–6, http://dx.doi.org/10.1109/MLSP.2012.6349712.
- Zoss A.B., Kazerooni H., Chu A. Biomechanical design of the Berkeley lower extremity exoskeleton (BLEEX). IEEE/ASME Trans Mech 2006; 11(2): 128–138, http://dx.doi.org/10.1109/TMECH.2006.871087.
- Shenoy P., Miller K.J., Crawford B., Rao P.N. Online electromyographic control of a robotic prosthesis. IEEE Trans Biomed Eng 2008; 55(3): 1128–1135, http://dx.doi.org/10.1109/TBME.2007.909536.
- Farrell T.R., Weir R.F. The optimal controller delay for myoelectric prostheses. IEEE Trans Neur Sys Rehabil Eng 2007; 15(1): 111–118, http://dx.doi.org/10.1109/TNSRE.2007.891391.
- Phinyomark A., Limsakul C., Phukpattaranont P. A novel feature extraction for robust EMG pattern recognition. J Comput 2009; 1(1): 71–80.
- Bichler O., Querlioz D., Thorpe S.J., Bourgoin J.-P., Gamrat C. Unsupervised features extraction from asynchronous silicon retina through spike-timing-dependent plasticity. In: Proceedings of the 2011 International Joint Conference on Neural Networks 2011. San Jose, USA; 2011; p. 859–866, http://dx.doi.org/10.1109/IJCNN.2011.6033311.
- Mironov V., Lobov S., Kastalskiy I., Kazantsev V. Myoelectric control system of lower limb exoskeleton for re-training motion deficiencies. Neural Information Processing 2015; 9492: 428–435, http://dx.doi.org/10.1007/978-3-319-26561-2_51.
- Lobov S., Mironov V., Kastalskiy I., Kazantsev V. A spiking neural network in sEMG feature extraction. Sensors 2015; 15(11): 27894–27904, http://dx.doi.org/10.3390/s151127894.