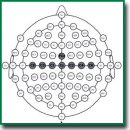
Exoskeleton Control System Based on Motor-Imaginary Brain–Computer Interface
The aim of the investigation was to develop the neuro-integrated control system for a lower-limb robotic exoskeleton (RE) using brain–computer interface (BCI) technology based on recognition of EEG patterns evoked by motor imagery of limb movement.
Materials and Methods. The proposed neuro-integrated RE control system based on BCI technology consists of three main modules: EEG signal recording module, EEG signal classifier and the software for transmission of commands to RE. EEG patterns evoked by motor imagery are recognized by the classifier based on linear discriminant analysis that uses the features identified by spatial filtering applying CSP method for all types of commands pairwise. The proposed algorithms for classification of motor imagery patterns and user training techniques make it possible to reliably distinguish several (up to 4) different commands. After training and testing the classifier, the operator may proceed to control the external device, i.e. the lower-limb RE. RE control software has been developed for easy system customization. The software has a simple graphical user interface and allows the user to change the mapping of RE patterns and commands in the operation process.
Results. As a result of testing in 14 healthy volunteers, the average accuracy of lower limb exoskeleton control based on the developed motor imagery BCI for three commands was found to average 70% in three sessions.
Conclusion. The developed RE control system based on BCI technology offers fairly high accuracy for three commands. The operators successfully learn to practice motor imagery and operate the BCI contour, even if they have no previous experience of work with brain–machine interfaces.
- Kaplan A.Ya. Neurophysiological foundations and practical realizations of the brain–machine interfaces in the technology in neurological rehabilitation. Human Physiology 2016; 42(1): 103–110, https://doi.org/10.1134/s0362119716010102.
- Fedotchev А.I., Parin S.B., Polevaya S.A., Velikova S.D. Brain–computer interface and neurofeedback technologies: current state, problems and clinical prospects (review). Sovremennye tehnologii v medicine 2017; 9(1): 175–184, https://doi.org/10.17691/stm2017.9.1.22.
- Nudo R.J., Milliken G.W., Jenkins W.M., Merzenich M.M. Use-dependent alterations of movement representations in primary motor cortex of adult squirrel monkeys. J Neurosci 1996; 16(2): 785–807.
- Bach-Y-Rita P. Theoretical and practical considerations in the restoration of function after stroke. Top Stroke Rehabil 2001; 8(3): 1–15, https://doi.org/10.1310/8t1t-etxu-8pdf-9x7f.
- Taub E., Uswatte G., Elbert T. New treatments in neurorehabilitation founded on basic research. Nat Rev Neurosci 2002; 3(3): 228–236, https://doi.org/10.1038/nrn754.
- Kwakkel G., Wagenaar R.C., Twisk J.W., Lankhorst G.J., Koetsier J.C. Intensity of leg and arm training after primary middle-cerebral-artery stroke: a randomised trial. Lancet 1999; 354(9174): 191–196, https://doi.org/10.1016/s0140-6736(98)09477-x.
- Marchal-Crespo L., Reinkensmeyer D.J. Review of control strategies for robotic movement training after neurologic injury. J Neuroeng Rehabil 2009; 6(1): 20, https://doi.org/10.1186/1743-0003-6-20.
- Frolov A.A., Biryukova E.V., Bobrov P.D., Mokienko O.A., Platonov A.K., Pryanichnikov V.E., Chernikova L.A. Principles of neurorehabilitation based on the brain–computer interface and biologically adequate control of the exoskeleton. Human Physiology 2013; 39(2): 196–208, https://doi.org/10.1134/s0362119713020035.
- Van der Kooij H., Koopman B., van Asseldonk E.H.F. Body weight support by virtual model control of an impedance controlled exoskeleton (LOPES) for gait training. Conf Proc IEEE Eng Med Biol Soc 2008; 2008: 1969–1972, https://doi.org/10.1109/iembs.2008.4649574.
- Banala S.K., Seok H.K., Agrawal S.K., Scholz J.P. Robot assisted gait training with active leg exoskeleton (ALEX). IEEE Trans Neural Syst Rehabil Eng 2009; 17(1): 2–8, https://doi.org/10.1109/tnsre.2008.2008280.
- Wang S., Wang L., Meijneke C., van Asseldonk E., Hoellinger T., Cheron G., Ivanenko Y., La Scaleia V., Sylos-Labini F., Molinari M., Tamburella F., Pisotta I., Thorsteinsson F., Ilzkovitz M., Gancet J., Nevatia Y., Hauffe R., Zanow F., van der Kooij H. Design and control of the MINDWALKER exoskeleton. IEEE Trans Neural Syst Rehabil Eng 2015; 23(2): 277–286, https://doi.org/10.1109/tnsre.2014.2365697.
- Kaplan A.Ya., Lim J.J., Jin K.S., Park B.W., Byeon J.G., Tarasova S.U. Unconscious operant conditioning in the paradigm of brain-computer interface based on color perception. Int J Neurosci 2005; 115(6): 781–802, https://doi.org/10.1080/00207450590881975.
- Wolpaw J.R., Birbaumer N., McFarland D.J., Pfurtscheller G., Vaughan T.M. Brain–computer interfaces for communication and control. Clin Neurophysiol 2002; 113(6): 767–791, https://doi.org/10.1016/s1388-2457(02)00057-3.
- Kelly S.P., Lalor E.C., Finucane C., McDarby G., Reilly R.B. Visual spatial attention control in an independent brain–computer interface. IEEE Trans Biomed Eng 2005; 52(9): 1588–1596, https://doi.org/10.1109/tbme.2005.851510.
- Kaplan A.Ya., Zhigulskaya D.D., Kirjanov D.A. Studying the ability to control human phantom fingers in P300 brain-computer interface. Bulletin of Russian State Medical University 2016; 2: 24–28.
- Farwell L.A., Donchin E. Talking off the top of your head: toward a mental prosthesis utilizing event-related brain potentials. Electroencephalogr Clin Neurophysiol 1988; 70(6): 510–523, https://doi.org/10.1016/0013-4694(88)90149-6.
- Brunner P., Bianchi L., Guger C., Cincotti F., Schalk G. Current trends in hardware and software for brain–computer interfaces (BCIs). J Neural Eng 2011; 8(2): 025001, https://doi.org/10.1088/1741-2560/8/2/025001.
- Kwak N.S., Müller K.R., Lee S.W. A lower limb exoskeleton control system based on steady state visual evoked potentials. J Neural Eng 2015; 12(5): 056009, https://doi.org/10.1088/1741-2560/12/5/056009.
- Kaplan A., Vasilyev A., Liburkina S., Yakovlev L. Poor BCI performers still could benefit from motor imagery training. In: Schmorrow D., Fidopiastis C. (editors). Foundations of augmented cognition: neuroergonomics and operational neuroscience. AC 2016. Lecture notes in computer science. Vol 9743. Springer International Publishing, Cham; 2016; p. 46–56, https://doi.org/10.1007/978-3-319-39955-3_5.
- Mulder Th. Motor imagery and action observation: cognitive tools for rehabilitation. J Neural Transm (Vienna) 2007; 114(10): 1265–1278, https://doi.org/10.1007/s00702-007-0763-z.
- Vasilyev A.N., Liburkina S.P., Kaplan A.Ya. Lateralization of EEG рatterns in humans during motor imagery of arm movements in the brain–computer interface. Zhurnal vysshey nervnoy deyatel’nosti im. I.P. Pavlova 2016; 66(3): 302–312, https://doi.org/10.7868/s0044467716030126.
- De Vries S., Tepper M., Feenstra W., Oosterveld H., Boonstra A.M., Otten B. Motor imagery ability in stroke patients: the relationship between implicit and explicit motor imagery measures. Front Hum Neurosci 2013; 7: 790, https://doi.org/10.3389/fnhum.2013.00790.
- Do A.H., Wang P.T., King C.E., Chun S.N., Nenadic Z. Brain-computer interface controlled robotic gait orthosis. J Neuroeng Rehabil 2013; 10(1): 111, https://doi.org/10.1186/1743-0003-10-111.
- Contreras-Vidal J.L., Grossman R.G. NeuroRex: a clinical neural interface roadmap for EEG-based brain machine interfaces to a lower body robotic exoskeleton. Conf Proc IEEE Eng Med Biol Soc 2013; 2013: 1579–1582, https://doi.org/10.1109/embc.2013.6609816.
- Donati A.R., Shokur S., Morya E., Campos D.S., Moioli R.C., Gitti C.M., Augusto P.B., Tripodi S., Pires C.G., Pereira G.A., Brasil F.L., Gallo S., Lin A.A., Takigami A.K., Aratanha M.A., Joshi S., Bleuler H., Cheng G., Rudolph A., Nicolelis M.A. Long-term training with a brain-machine interface-based gait protocol induces partial neurological recovery in paraplegic patients. Sci Rep 2016; 6: 30383, https://doi.org/10.1038/srep30383.
- Hjort B. An on-line transformation of EEG scalp potentials into orthogonal source derivations. Electroencephalogr Clin Neurophysiol 1975; 39(5): 526–530, https://doi.org/10.1016/0013-4694(75)90056-5.
- Koles Z.J., Lazar M.S., Zhou S.Z. Spatial patterns underlying population differences in the background EEG. Brain Topogr 1990; 2(4): 275–284, https://doi.org/10.1007/bf01129656.
- Mineev S.A., Novikov V.A., Kuzmina I.V., Shatalin R.A., Grin I.V. Goniometric sensor interface for exoskeleton system control device. Biomed Eng 2016; 49(6): 357–361, https://doi.org/10.1007/s10527-016-9566-6.