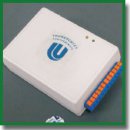
A Mobile Exoskeleton Control System Using Electromyographic Signals from Human Muscles
The aim of the study was to develop a wireless system of registration and analysis of electromyographic (EMG) signals from human muscles to provide intelligent assistance to patients with motor disorders.
Results. Single-channel and four-channel systems for translating muscle tension signals into exoskeleton control commands and control algorithms have been developed and tested. They have an advantage in mobility, portability and availability of wireless EMG signal sensors-amplifiers (myographs). Experimental testing of both systems has demonstrated a high quality of EMG signal and the ability of proportional actuator control. The mobile myographic can be applied in the field of functional diagnosis, neurointerfaces, medical rehabilitation, assistive devices, control systems, and gaming applications.
- Kaplan A.Ya. Neurophysiological foundations and practical realizations of the brain-machine interfaces the technology in neurological rehabilitation. Fiziologiya cheloveka 2016; 42(1): 118–127, https://doi.org/10.7868/S0131164616010100.
- De Luca C.J., Kuznetsov M., Gilmore L.D., Roy S.H. Inter-electrode spacing of surface EMG sensors: reduction of crosstalk contamination during voluntary contractions. J Biomech 2012; 45(3): 555–561, https://doi.org/10.1016/j.jbiomech.2011.11.010.
- Saponas T.S., Tan D.S., Morris D., Balakrishnan R. Demonstrating the feasibility of using forearm electromyography for muscle-computer interfaces. In: CHI ‘08 Proceedings of the SIGCHI Conference on Human Factors in Computing Systems. ACM Press; 2008, 515–524, https://doi.org/10.1145/1357054.1357138.
- Chaudhary A., Raheja J.L. Bent fingers’ angle calculation using supervised ANN to control electro-mechanical robotic hand. Computers & Electrical Engineering 2013; 39(2): 560–570, https://doi.org/10.1016/j.compeleceng.2012.07.012.
- Mathiassen S.E., Winkel J., Hägg G.M. Normalization of surface EMG amplitude from the upper trapezius muscle in ergonomic studies — a review. J Electromyogr Kinesiol 1995; 5(4): 197–226, https://doi.org/10.1016/1050-6411(94)00014-X.
- Jiang Y., Sakoda S., Hoshigawa S., Ye H., Yabuki Y., Nakamura T., Ishihara M., Takagi T., Takayama S., Yokoi H. Development and evaluation of simplified EMG prosthetic hands. In: 2014 IEEE International Conference on Robotics and Biomimetics (ROBIO 2014). IEEE; 2014; 1368–1373, https://doi.org/10.1109/ROBIO.2014.7090524.
- Lobov S., Mironov V.,
Kastalskiy I., Kazantsev V. A spiking neural network in sEMG feature extraction. Sensors 2015; 15(11): 27894–27904, https://doi.org/10.3390/s151127894. - Siqueira Júnior A.L.D., Soares A.B. A novel method for EMG decomposition based on matched filters. Res Biomed Eng 2015; 31(1): 44–55, https://doi.org/10.1590/2446-4740.0643.
- Huang H.-P., Liu Y.-H., Liu L.-W., Wong C.-S. EMG classification for prehensile postures using
cascaded architecture of neural networks with self-organizing maps. In: 2003 IEEE International Conference on Robotics and Automation. IEEE; 2003; 1497–1502, https://doi.org/10.1109/ROBOT.2003.1241803. - Christodoulou C.I., Pattichis C.S. Unsupervised pattern recognition for the classification of EMG signals. IEEE Trans Biomed Eng 1999; 46(2): 169–178, https://doi.org/10.1109/10.740879.
- Micera S., Carpaneto J., Raspopovic S. Control of hand prostheses using peripheral information. IEEE Rev Biomed Eng 2010; 3: 48–68, https://doi.org/10.1109/RBME.2010.2085429.
- Molanes R.F., Fariña J., Rodríguez-Andina J.J., Portela M. Design of a muscle activity monitor for rehabilitation of post-stroke patients. In: 2015 IEEE International Conference on Industrial Technology (ICIT). IEEE; 2015; p. 1427–1432, https://doi.org/10.1109/icit.2015.7125297.
- Tsukahara A., Kawanishi R., Hasegawa Y., Sankai Y. Sit-to-stand and stand-to-sit transfer support for complete paraplegic patients with robot suit HAL. Advanced Robotics 2010; 24(11): 1615–1638, https://doi.org/10.1163/016918610x512622.
- Strausser K.A., Kazerooni H. The development and testing of a
human machine interface for a mobile medical exoskeleton. In: 2011 IEEE/RSJ International Conference on Intelligent Robots and Systems. IEEE; 2011; p. 4911–4916, https://doi.org/10.1109/iros.2011.6095025. - Zeilig G.,
Weingarden H., Zwecker M., Dudkiewicz I., Bloch A., Esquenazi A. Safety and tolerance of the ReWalk™ exoskeleton suit for ambulation by people with complete spinal cord injury: a pilot study. J Spinal Cord Med 2012; 35(2): 96–101, https://doi.org/10.1179/2045772312y.0000000003. - Koller J.R., Jacobs D.A., Ferris D.P., Remy C.D. Learning to walk with an adaptive gain proportional myoelectric controller for a robotic ankle exoskeleton. J Neuroeng Rehabil 2015; 12(1): 97, https://doi.org/10.1186/s12984-015-0086-5.
- Tang Z., Zhang K., Sun S., Gao Z., Zhang L., Yang Z. An upper-limb power-assist exoskeleton using proportional myoelectric control. Sensors 2014; 14(4): 6677–6694, https://doi.org/10.3390/s140406677.
- George T., Shalu G.K., Sivanandan K.S. Sensing, processing and application of EMG signals for HAL (Hybrid Assistive Limb). In: International Conference on Sustainable Energy and Intelligent Systems (SEISCON 2011). IET; 2011; p. 749–753, https://doi.org/10.1049/cp.2011.0463.
- Dudnik A.V., Lobov S.A.,
Kastalskiy I.A. The program (MyoStep) for detecting gait phases based on the electromyogram of the leg muscles. Inventor’s Certificate RU 2016663327. 2017. - Khoruzhko M.A., Pimashkin A.S., Shamshin M.O. The program (ExoMyo) for proportional exoskeleton control using musle activity signals. Inventor’s Certificate RU 2017611289. 2017.