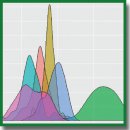
Statistical Classification of Immunosignatures under Significant Reduction of the Feature Space Dimensions for Early Diagnosis of Diseases
The aim of the study is to explore the options of significantly reducing the feature space of immunosignatures by selecting the most informative features while maintaining the reasonable quality of the human disease classification.
Materials and Methods. The immunosignature technology is based on the use of peptide microchips, where peptides with random amino acid sequences serve for diagnostic purposes. Such peptides have partial or complete similarity with the antigen epitopes. The diagnosis is made by using classification algorithms, developed from a reduced sample of immunosignature data of patients with known diagnoses.
The data. To carry out the experiments, the immunosignature data obtained from high-resolution peptide microchips containing about ten thousand peptide cells were used. The digitized data for composing the samples was obtained from the public NCBI database (identified as GSE52580).
Searching for informative parameters. To reduce the dimensionality of the data space, we conducted a search for the most informative peptides. For this purpose, we tested various statistical criteria and group discriminators (such as the Student’s t-test, the Mann–Whitney–Wilcoxon U test, the Kolmogorov–Smirnov test, and the Jeffries–Matusita distance) for their applicability to this search.
Classification methods. Classifiers based on various mathematical models were used: i.e. the support vector machine, the naive Bayesian classifier, the random forest, and the gradient boosting.
Evaluation of the quality of classification. The proportion of correct accuracy was used to evaluate both binary and multiclass classification.
Results. The present studies demonstrate that by reducing the dimensionality and by searching for the informative peptides it becomes possible to reduce the time needed for the classification processing (ranged from 16-fold to 1625-fold), as well as to reduce the feature space (240-fold) without compromising the quality of classification. It has been shown that all tested classifiers are equally successful in solving the problem of immunosignature classification.
Conclusion. The results rationalize the proposed approach to reducing the initial feature space of immunosignature data in order to accelerate the classification process without reducing its accuracy.
- World Cancer Report 2014. Geneva: World Health Organization, International Agency for Research on Cancer; 2014.
- Ntagirabiri R., Munezero B., Nizigiyimana G., Ngomirakiza J.B., Ndabaneze E. Assessment of diagnostic efficiency of the optic upper digestive endoscopy in the era of video endoscopy. Journal Africain d’Hépato-Gastroentérologie 2015; 9(2): 64–67, https://doi.org/10.1007/s12157-015-0587-7.
- O’Donnell B., Maurer A., Papandreou-Suppappola A., Stafford P. Time‑frequency analysis of peptide microarray data: application to brain cancer
immunosignatures . Cancer Inform 2015; 14(2): 219–233, https://doi.org/10.4137/cin.s17285 - Richer J., Johnston S.A., Stafford P. Epitope identification from fixed-complexity random‑sequence peptide microarrays. Mol Cell Proteomics 2015; 14(1): 136–147, https://doi.org/10.1074/mcp.m114.043513.
- Kukreja M., Johnston S.A., Stafford P. Immunosignaturing microarrays distinguish antibody profiles of related pancreatic diseases. J Proteomics Bioinform 2012; 1(S6): 001, https://doi.org/10.4172/jpb.s6-001.
- Stafford P., Cichacz Z., Woodbury N.W. Immunosignature system for diagnosis of cancer. Proc Natl Acad Sci USA 2014; 111(30): E3072–E3080, https://doi.org/10.1073/pnas.1409432111.
- Singh S., Stafford P., Schlauch K.A., Tillett R.R.,
Gollery M., Johnston S.A., Khaiboullina S.F., De Meirleir K.L., Rawat S., Mijatovic T., Subramanian K., Palotás A., Lombardi V.C. Humoral immunity profiling of subjects with myalgic encephalomyelitis using a random peptide microarray differentiates cases from controls with high specificity and sensitivity. Mol Neurobiol 2016; 55(1): 633–641, https://doi.org/10.1007/s12035-016-0334-0. - Chapoval A.I., Legutki J.B., Stafford P., Trebukhov A.V., Johnston S.A., Shoykhet Ya.N., Lazarev A.F. Immunosignature — peptide microarray for diagnostic of cancer and other diseases. Rossijskij onkologiceskij zurnal 2014; 19(4): 6–11.
- Osipova T.V., Ryabykh T.P., Baryshnikov A.Yu. Diagnostic microchips: application in oncology. Rossijskij bioterapevticeskij zurnal 2006; 5(3): 72–81.
- Andryushchenko V.S., Perets E.Yu., Lyalyukhova I.E. Klassifikatsiya immunosignaturnykh dannykh dlya zadach ranney diagnostiki opasnykh zabolevaniy. V kn.: Informatsionnye tekhnologii i matematicheskoe modelirovanie (ITMM-2017) [Classification of immunosignature data applied to the early diagnosis of dangerous diseases. In: Information technologies and mathematical modeling (ITMM-2017)]. Tomsk; 2017; p. 18–25.
- Stafford P., Halperin R., Legutki J.B., Magee D.M., Galgiani J., Johnston S.A. Physical characterization of the “
immunosignaturing effect”. Mol Cell Proteomics 2012; 11(4): M111.011593, https://doi.org/10.1074/mcp.m111.011593. - GSE52580. URL: https://www.ncbi.nlm.nih.gov/geo/query/acc.cgi?acc=GSE52580
- Student. The probable error of a mean. Biometrika 1908; 6(1): 1–25, https://doi.org/10.2307/2331554.
- Mann H.B., Whitney D.R. On a test of whether one of two random variables is stochastically larger than the other. The Annals of Mathematical Statistics 1947; 18(1): 50–60, https://doi.org/10.1214/aoms/1177730491.
- Salvia A.A. Some fundamental properties of Kolmogorov–Smirnov consonance sets. Technometrics 1980; 22(1): 109–111, https://doi.org/10.2307/1268389.
Matusita K. Statistical theory and data analysis. Biometrics 1985; 41(3): 815, https://doi.org/10.2307/2531311.- Cumming G. Replication and p intervals: p values predict the future only vaguely, but confidence intervals do much better. Perspect Psychol Sci 2008; 3(4): 286–300, https://doi.org/10.1111/j.1745-6924.2008.00079.x.
- Mammone A., Turchi M., Cristianini N. Support vector machines. Wiley Interdisciplinary Reviews: Computational Statistics 2009; 1(3): 283–289, https://doi.org/10.1002/wics.49.
- Shaik L., Swamy N.N. Efficient implementation of
class based decomposition schemes for naivebayes classifier. International Journal of Science and Research 2015; 4(11): 237–240, https://doi.org/10.21275/v4i11.nov151091. - Breiman L. Random forests. Machine Learning 2001; 45(1): 5–32, https://doi.org/10.1023/a:1010933404324.
- Natekin A., Knoll A. Gradient boosting machines, a tutorial. Front Neurorobot 2013; 7: 21, https://doi.org/10.3389/fnbot.2013.00021.
- Friedman J.H. Greedy function approximation: a gradient boosting machine. Ann Statist 2001; 29(5): 1189–1232, https://doi.org/10.1214/aos/1013203451.
- Ting K.M. Covariance Matrix. In: Sammut C., Webb G. (editors). Encyclopedia of machine learning and data mining. Boston, МА: Springer; 2016, https://doi.org/10.1007/978-1-4899-7502-7_50-1.
- Sylvain A., Celisse A. A survey of cross-validation procedures for model selection. Statistics Surveys 2010; 4(0): 40–79, https://doi.org/10.1214/09-ss054.