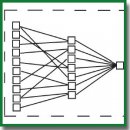
The Neural Network Technology Application for Prediction of Preeclampsia in Pregnant Women with Chronic Arterial Hypertension
The aim of the study was to assess biomedical risk factors for preeclampsia in pregnant women with chronic arterial hypertension (CAH) and on this basis to create the neural network system for calculating the probability of developing preeclampsia in these women.
Materials and Methods. Pregnancy and delivery outcomes were analyzed in 548 patients with pre-existing arterial hypertension (AH): 318 with CAH and 230 with preeclampsia secondary to CAH. Risk factors were calculated using the OpenEpi program (UK). A combined method of global optimization and neural network method of information compression were used when training the developed neural network system.
Results. There were identified the main risk factors for developing preeclampsia in pregnant women with CAH: hereditary burden of hypertension; hypertensive disorders in previous pregnancies; hypertension during more than five years; the initial diastolic blood pressure being more than 80 mm Hg; body mass index more than 30; tobacco smoking; nulliparity; chronic pyelonephritis and gastritis; hypertensive disease stage II; degree II and III AH; hypertensive retinal angiopathy; left ventricular hypertrophy; lack of regular antihypertensive therapy before and during pregnancy; late treatment initiation. The data obtained were used to train and test the neural network software and to develop the “Neuro_Chronic — neural network system for predicting secondary preeclampsia in pregnant women with chronic arterial hypertension”. The system includes two modules. The first module is designed to train the neural network software model using a given set of images, the second module provides evaluation of preeclampsia developing during pregnancy in a particular patient in the form of five probability options — from very low to very high — after entering the parameters obtained during the anamnestic and clinical examination into the corresponding fields.
Conclusion. Revealing the proposed predictors of preeclampsia in pregnant women with CAH and entering these data into the developed computer program will enable physicians to determine the probability of preeclampsia developing during gestation at the outpatient stage and to take timely preventive measures in pregnant women at high-risk.
- Rezul’taty konfidentsial’nogo audita materinskoy smertnosti v Rossiyskoy Federatsii v 2014 godu: metodicheskoe pis’mo Minzdrava Rossii [The results of confidential audit of maternal mortality in the Russian Federation in 2014: methodology letter, the Ministry of Healthcare of the Russian Federation]. Moscow; 2015.
- Frolova O.G., Shuvalov M.P., Guseva E.V., Ryabinkina I.N. Maternal mortality at the present stage of development of the healthcare system. Akusherstvo i ginekologiia 2016; 12: 121–124, https://doi.org/10.18565/aig.2016.12.121-4.
- Kinzhalova S.V., Pestryaeva L.A., Makarov R.A., Davydova N.S. Differential diagnosis of hypertensive disorders in pregnancy. Akusherstvo i ginekologiia 2015; 11: 36–40.
- Shahbazova N.A. Pregnancy outcomes in gestational hypertensive disorders. Akusherstvo i ginekologiia 2015; 2: 20–26.
- Makarov O.V., Tkacheva O.N., Volkova E.V. Preeklampsiya i khronicheskaya arterial’naya gipertenziya. Klinicheskie aspekty [Preeclampsia and chronic hypertension. Clinical aspects]. Moscow: GEOTAR-Media; 2010.
Storya L.,Chappellb L.C. Preterm preeclampsia: what every neonatologist should know. Early Hum Dev 2017; 114: 26–30, https://doi.org/10.1016/j.earlhumdev.2017.09.010.- Dolgushina V.F., Chulkov V.S., Vereina N.K., Sinitsyn S.P. Assessing the relationship of clinical and genetic factors to pregnancy complications and outcomes in women with preeclampsia in the presence of chronic hypertension. Rossiyskiy vestnik akushera-ginekologa 2014; 14(6): 4–8.
- Kurochka M.P. Analysis of preeclampsia and eclampsia risk factors in cases of maternal death. Saratovskiy nauchno-meditsinskiy zhurnal 2013; 9(2): 230–234.
- Suzuki S., Igarashi M. Risk factors for preeclampsia in Japanese twin pregnancies: comparison with those in singleton pregnancies. Arch Gynecol Obstet 2009; 280(3): 389–393, https://doi.org/10.1007/s00404-009-0932-4.
- López-Carbajal M.J., Manríquez-Moreno M.E., Gálvez-Camargo D., Ramírez-Jiménez E. Risk factors associated to preclampsia. Rev Med Inst Mex Seguro Soc 2012; 50(5): 471–476.
- Bushtyreva I.O., Kurochka M.P., Gaida O.V. Prognostic criteria for preeclampsia. Rossiiskii vestnik akushera-ginekologa 2017; 17(2): 59–63, https://doi.org/10.17116/rosakush201717259-63.
- Giguère Y., Charland M., Bujold E., Bernard N., Grenier S., Rousseau F., Lafond J., Légaré F., Forest J.C. Combining biochemical and ultrasonographic markers in predicting preeclampsia: a systematic review. Clin Chem 2010; 56(3): 361–375, https://doi.org/10.1373/clinchem.2009.134080.
- Meler E., Figueras F., Bennasar M., Gomez O., Crispi F., Gratacos E. The prognostic role of uterine artery Doppler investigation in patients with severe early-onset preeclampsia. Am J Obstet Gynecol 2010; 202(6): 551–554, https://doi.org/10.1016/j.ajog.2010.01.048.
- Mulla Z.D., Nuwayhid B.S., Garcia K.M., Flood-Shaffer K., Van Hook J.W., Hampton R.M. Risk factors for a prolonged length of stay in women hospitalized for preeclampsia in Texas. Hypertens Pregnancy 2010; 29(1): 54–68, https://doi.org/10.3109/10641950902777754.
- Sidorova I.S., Nikitina N.A. Preeclampsia in the focus of a practitioner’s attention. Akusherstvo i ginekologiia 2014; 6: 4–9.
- Volkova E.V., Lysiuk E.Iu., Dzhokhadze L.S., Makarov O.V. Specific features of changes in pro- and antiangiogenic factors in pregnant women with chronic arterial hypertension. Rossiyskiy vestnik akushera-ginekologa 2013; 13(3): 9–13.
- Perni U.C., Wikström A.K., Cnattingius S., Villamor E. Interpregnancy change in smoking habits and risk of preeclampsia: a population-based study. Am J Hypertens 2012; 25(3): 372–378, https://doi.org/10.1038/ajh.2011.225.
- Lisonkova S., Joseph K.S. Left truncation bias as a potential explanation for the protective effect of smoking on preeclampsia. Epidemiology 2015; 26(3): 436–440, https://doi.org/10.1097/ede.0000000000000268.
- Karumanchi S.A., Levine R.J. How does smoking reduce the risk of preeclampsia? Hypertension 2010; 55(5): 1100–1101, https://doi.org/10.1161/hypertensionaha.109.148973.
- Wikström A.K., Stephansson O., Cnattingius S. Tobacco use during pregnancy and preeclampsia risk: effects of cigarette smoking and snuff. Hypertension 2010; 55(5): 1254–1259, https://doi.org/10.1161/hypertensionaha.109.147082.
- Rossiev D.A. Meditsinskaya neyroinformatika. V kn.: Neyroinformatika — 96 [Medical neuroinformatics. In: Neuroinformatics — 96]. URL: http://ww w.gotai.net/documents/doc-art-003-08.aspx.
- Gipertenzivnye rasstroystva vo vremya beremennosti, v rodakh i poslerodovom periode. Preeklampsiya. Eklampsiya: klinicheskie rekomendatsii (protokol lecheniya) [Hypertensive disorders during pregnancy, the intrapartum and postpartum periods. Preeclampsia. Eclampsia: clinical guidelines (treatment protocol)]. Moscow; 2016.
- Panova I.A., Rokotyanskaya E.A., Khlipunova D.A., Smirnova E.V. Prediktory preeklampsii u zhenshchin s khronicheskoy arterial’noy gipertenziey, beremennost’ kotorykh zavershilas’ prezhdevremennymi rodami. V kn.: Aktual’nye voprosy okhrany reproduktivnogo zdorov’ya sem’i v svete “Kontseptsii demograficheskoy politiki na period do 2025 goda” [Predictors of preeclampsia in women with chronic hypertension whose pregnancy ended in preterm delivery. In: Topical issues of reproductive healthcare and family planning in light of “The concept of demographic policy for the period up to 2025”]. Pod red. Malyshkinoy A.I. [Malyshkina A.I. (editor)]. Ivanovo: Izdatel’stvo MIK; 2014; p. 69–72.
- Panova I.A., Malyshkina A.I., Rokotianskaia E.A., Smirnova E.V. Risk factors for addition of preeclampsia in women with chronic hypertension. Rossiyskiy vestnik akushera-ginekologa 2014; 14(6): 37–42.
- Posiseeva L.V., Talaev A.M., Panova I.A., Talaeva E.M. Rheorenographic criteria for the prediction of gestosis in pregnant women with chronic pyelonephritis. Rossiyskiy vestnik akushera-ginekologa 2004; 4(4): 4–5.
- Fetisova I.N., Panova I.A., Rokotyanskaya E.A., Ratnikova S.Yu., Smirnova E.V., Fetisov N.S. The genetic factors which exert influence on preeclampsia development. Vestnik Ivanovskoy meditsinskoy akademii 2015; 20(3): 13–16.
- Yasinsky I.F. On the information compression by means of the neural network funnel. Izvestiya vysshikh uchebnykh zavedeniy. Tekhnologiya tekstil’noy promyshlennosti 2012; 5(341): 154–159.
- Yasinskiy I.F. Synthetic algorithm of optimization and adjustment of neural networks. Izvestiya vysshikh uchebnykh zavedeniy. Tekhnologiya tekstil’noy promyshlennosti 2007; 3(298): 119–123.
- Golovko V.A. Neyrokompyutery i ikh primenenie. Neyronnye seti. Kniga 4. Obuchenie, organizatsiya i primenenie [Neurocomputers and their application. Book 4. Neural networks: training, organization and application]. Moscow: IPRZhR; 2001; 256 p.