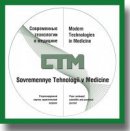
Modern Technologies in Studying the Mechanisms, Diagnostics, and Treatment of Autism Spectrum Disorders (Review)
Autism spectrum disorders (ASD) are among the most common and intractable neurological diseases characterized by high heterogeneity and requiring a person-oriented approach to diagnostics and treatment. The purpose of this review is to summarize the literature data of the last 5 years on the contribution of modern technologies to the knowledge of mechanisms, diagnostics, and treatment of ASD. Particular attention is paid to the possibilities of non-drug treatment of ASD with the help of neurointerface technologies, including the brain–computer interface and neurofeedback technologies. The advantages of the musical neurointerface elaborated by the authors with complex feedback from brain and heart biopotentials, providing the possibility of personalized treatment of ASD, are grounded.
- Borodina L.G., Pis’mennaya N.V. Medical aspects of support to children with autism spectrum disorders. Autizm i narusheniya razvitiya 2017; 15(3): 3–8, https://doi.org/10.17759/autdd.2017150301.
- Potokina A.M. Razmyshleniya klinicheskogo psikhologa ob autizme. V kn.: Tendentsii razvitiya nauki i obrazovaniya [Reflections of the clinical psychologist on autism. In: Trends in the development of science and education]. Samara; 2017; p. 35–37, https://doi.org/10.18411/lj-31-08-2017-29.
- Constantino J.N. Deconstructing autism: from unitary syndrome to contributory developmental endophenotypes. Int Rev Psychiatry 2018; 30(1): 18–24, https://doi.org/10.1080/09540261.2018.1433133.
- Simashkova N.V., Iakupova L.P., Kliushnik T.P., Koval-Zaitsev A.A. A multidiscipline clinical and biological approach to the study of psychotic types of autistic spectrum disorders in children. Zhurnal nevrologii i psikhiatrii imeni S.S. Korsakova 2013; 113(5–2): 35–42.
- Simashkova N.V., Klyushnik T.P., Koval-Zaitsev A.A., Yakupova L.P. Multidisciplinary clinical and psychological aspects of diagnosis. Autizm i narusheniya razvitiya 2016; 14(4): 51–67, https://doi.org/10.17759/autdd.2016140408.
- Weintraub K. The prevalence puzzle: autism counts. Nature 2011; 479(7371): 22–24, https://doi.org/10.1038/479022a.
- Hollin G. Autistic heterogeneity: linking uncertainties and indeterminacies. Sci Cult 2017; 26(2): 209–231, https://doi.org/10.1080/09505431.2016.1238886.
- Grebennikova E.V., Shelehov I.L., Filimonova E.A. Understanding autism spectrum disorder from an interdisciplinary perspective. Nauchno-pedagogicheskoe obozrenie 2016; 3(13): 16–22.
- Graf W.D., Miller G., Epstein L.G., Rapin I. The autism “epidemic”: ethical, legal, and social issues in a developmental spectrum disorder. Neurology 2017; 88(14): 1371–1380, https://doi.org/10.1212/wnl.0000000000003791.
- Thibaut F. New perspectives in autism spectrum disorders. Dialogues Clin Neurosci 2017; 19(4): 323.
- Ferri S.L., Abel T., Brodkin E.S. Sex differences in autism spectrum disorder: a review. Curr Psychiatry Rep 2018; 20(2): 9, https://doi.org/10.1007/s11920-018-0874-2.
- Morozov S.A., Morozova T.I., Belyavskiy B.V. On the issue of intellectual disability in autism spectrum disorders. Autizm i narusheniya razvitiya 2016; 14(1): 9–18, https://doi.org/10.17759/autdd.2016140102.
- Mamokhina U.A. Speech features in autism spectrum disorders. Autizm i narusheniya razvitiya 2017; 15(3): 24–33, https://doi.org/10.17759/autdd.2017150304.
- Besag F.M. Epilepsy in patients with autism: links, risks and treatment challenges. Neuropsychiatr Dis Treat 2017; 14: 1–10, https://doi.org/10.2147/ndt.s120509.
- Hudson C.C., Hall L., Harkness K.L. Prevalence of depressive disorders in individuals with autism spectrum disorder: a meta-analysis. J Abnorm Child Psychol 2018, https://doi.org/10.1007/s10802-018-0402-1 [Epub ahead of print].
- Rodgers J., Ofield A. Understanding,
recognising and treating co-occurring anxiety in autism. Curr Dev Disord Rep 2018; 5(1): 58–64, https://doi.org/10.1007/s40474-018-0132-7. - Stroganova T.A., Orekhova E.V., Galuta I.A. Monotropism of attention in autistic children. Eksperimental’naya psikhologiya 2014; 7(4): 66–82.
- Stroganova T.A., Orekhova E.V., Galuta I.A. Neural basis of attention orienting abnormalities in children with autism. Eksperimental’naya psikhologiya 2015; 8(3): 7–23, https://doi.org/10.17759/exppsy.2015080302.
- Boxhoorn S., Lopez E., Schmidt C., Schulze D., Hänig S., Freitag C.M. Attention profiles in autism spectrum disorder and subtypes of attention-deficit/hyperactivity disorder. Eur Child Adolesc Psychiatry 2018; 27(11): 1433–1447, https://doi.org/10.1007/s00787-018-1138-8.
- Nason B. Core challenges of autism. Sensory aspects of autism. Autizm i narusheniya razvitiya 2016; 14(3): 42–48, https://doi.org/10.17759/autdd.2016140304.
- Bogdashina O.B. Synaesthesia in autism. Autizm i narusheniya razvitiya 2016; 14(3): 21–31, https://doi.org/10.17759/autdd.2016140302.
- DuBois D., Lymer E., Gibson B.E., Desarkar P., Nalder E. Assessing sensory рrocessing dysfunction in adults and adolescents with autism spectrum disorder: a scoping review. Brain Sci 2017; 7(8): 108, https://doi.org/10.3390/brainsci7080108.
- Perez Repetto L., Jasmin E., Fombonne E., Gisel E., Couture M. Longitudinal study of sensory features in children with
autism spectrum disorder. Autism Res Treat 2017; 2017: 1934701, https://doi.org/10.1155/2017/1934701. - Machurina T.N. Infantile autism: diagnosis, therapy, rehabilitation. International Scientific Review 2016; 20(30): 105–108.
- Nason B. Core challenges of autism. Emotionality on spectrum. Autizm i narusheniya razvitiya 2017; 15(3): 58–68, https://doi.org/10.17759/autdd.2017150308.
- Chaste P., Leboyer M. Autism risk factors: genes, environment, and gene-environment interactions. Dialogues Clin Neurosci 2012; 14(3): 281–292.
- Siu M.T., Weksberg R. Epigenetics of autism spectrum disorder. Adv Exp Med Biol 2017; 978: 63–90, https://doi.org/10.1007/978-3-319-53889-1_4.
- Gilbert J., Man H.Y. Fundamental elements in autism: from neurogenesis and neurite growth to synaptic plasticity. Front Cell Neurosci 2017; 11: 359, https://doi.org/10.3389/fncel.2017.00359.
- Andrews D.S., Avino T.A., Gudbrandsen M., Daly E., Marquand A., Murphy C.M., Lai M.C., Lombardo M.V., Ruigrok A.N., Williams S.C., Bullmore E.T., The
Mrc Aims Consortium, Suckling J., Baron-Cohen S., Craig M.C., Murphy D.G., Ecker C. In vivo evidence of reduced integrity of the gray-white matter boundary in autism spectrum disorder. Cereb Cortex 2017; 27(2): 877–887, https://doi.org/10.1093/cercor/bhw404. - Albitskaya Zh.V. Early children autism — issues and difficulties of initial diagnostics in the case of interdisciplinary interaction. Medicinskij al’manah 2016; 2(42): 108–111, https://doi.org/10.21145/2499-9954-2016-2-108-111.
- Baldova S.N., Belova A.N., Sheyko G.E., Borzikov V.V., Kuznetsov A.N., Polyakova A.G., Loskutova N.V. Quantitative electroencephalography in autism spectrum disorders research. Prakticheskaya meditsina 2017; 1(102): 35–39.
- Gurau O., Bosl W.J., Newton C.R. How useful is electroencephalography in the diagnosis of autism spectrum disorders and the delineation of subtypes: a systematic review. Front Psychiatry 2017; 8: 121, https://doi.org/10.3389/fpsyt.2017.00121.
- Zeng K., Kang J., Ouyang G., Li J., Han J., Wang Y., Sokhadze E.M., Casanova M.F., Li X. Disrupted brain network in children with
autism spectrum disorder. Sci Rep 2017; 7(1): 16253, https://doi.org/10.1038/s41598-017-16440-z. - Dickinson A., DiStefano C., Senturk D., Jeste S.S. Peak alpha frequency is a neural marker of cognitive function across the autism spectrum. Eur J Neurosci 2018; 47(6): 643–651, https://doi.org/10.1111/ejn.13645.
- Di Palma S., Tonacci A., Narzisi A., Domenici C., Pioggia G., Muratori F., Billeci L. Monitoring of autonomic response to sociocognitive tasks during treatment in children with autism spectrum disorders by wearable technologies: a feasibility study. Comput Biol Med 2017; 85: 143–152, https://doi.org/10.1016/j.compbiomed.2016.04.001.
- Belova A.N., Borzikov V.V., Kuznetsov A.N., Komkova O.V. Activty of vegetative nervous system in accordance with the results of cardiac variability rhythm study in the case of children having disorders of autistic nature. Medicinskij al’manah 2017; 5(50): 130–136, https://doi.org/10.21145/2499-9954-2017-5-130-136.
- Duan F., Watanabe K., Yoshimura Y., Kikuchi M., Minabe Y., Aihara K. Detection of atypical network development patterns in children with autism spectrum disorder using magnetoencephalography. PLoS One 2017; 12(9): e0184422, https://doi.org/10.1371/journal.pone.0184422.
- Wu D., José J.V., Nurnberger J.I., Torres E.B. A biomarker characterizing neurodevelopment with applications in autism. Sci Rep 2018; 8(1): 614, https://doi.org/10.1038/s41598-017-18902-w.
- Galiana-Simal A., Muñoz-Martinez V., Calero-Bueno P., Vela-Romero M., Beato-Fernandez L. Towards a future molecular diagnosis of autism: recent advances in biomarkers research from saliva samples. Int J Dev Neurosci 2018; 67: 1–5, https://doi.org/10.1016/j.ijdevneu.2018.03.004.
- Qiao Y., Wu M., Feng Y., Zhou Z., Chen L., Chen F. Alterations of oral microbiota distinguish children with autism spectrum disorders from healthy controls. Sci Rep 2018; 8(1): 1597, https://doi.org/10.1038/s41598-018-19982-y.
- Li G., Lee O., Rabitz H.
High efficiency classification of children withautism spectrum disorder. PLoS One 2018; 13(2): e0192867, https://doi.org/10.1371/journal.pone.0192867. - Trevisan D.A., Roberts N., Lin C., Birmingham E. How do adults and teens with self-declared autism spectrum disorder experience eye contact? A qualitative analysis of first-hand accounts. PLoS One 2017; 12(11): e0188446, https://doi.org/10.1371/journal.pone.0188446.
- Cabibihan J.J., Javed H., Aldosari M., Frazier T.W., Elbashir H. Sensing technologies for autism spectrum disorder screening and intervention. Sensors 2016; 17(1): E46, https://doi.org/10.3390/s17010046.
- Vargas-Cuentas N.I., Roman-Gonzalez A., Gilman R.H., Barrientos F., Ting J., Hidalgo D., Jensen K., Zimic M. Developing an eye-tracking algorithm as a potential tool for early diagnosis of autism spectrum disorder in children. PLoS One 2017; 12(11): e0188826, https://doi.org/10.1371/journal.pone.0188826.
- Edmunds S.R., Rozga A., Li Y., Karp E.A., Ibanez L.V., Rehg J.M., Stone W.L. Brief report: using a point-of-view camera to measure eye gaze in young children with autism spectrum disorder during naturalistic social interactions: a pilot study. J Autism Dev Disord 2017; 47(3): 898–904, https://doi.org/10.1007/s10803-016-3002-3.
- Moriuchi J.M., Klin A., Jones W. Mechanisms of diminished attention to eyes in autism. Am J Psychiatry 2017; 174(1): 26–35, https://doi.org/10.1176/appi.ajp.2016.15091222.
- Owada K., Kojima M., Yassin W., Kuroda M., Kawakubo Y., Kuwabara H., Kano Y., Yamasue H. Computer-analyzed facial expression as a surrogate marker for autism spectrum social core symptoms. PLoS One 2018; 13(1): e0190442, https://doi.org/10.1371/journal.pone.0190442.
- Barton K.S., Tabor H.K., Starks H., Garrison N.A., Laurino M., Burke W. Pathways from autism spectrum disorder diagnosis to genetic testing. Genet Med 2018; 20(7): 737–744, https://doi.org/10.1038/gim.2017.166.
- Ungar W.J.
Next generation sequencing and health technology assessment in autism spectrum disorder. J Can Acad Child Adolesc Psychiatry 2015; 24(2): 123–127. - Payakachat N., Tilford J.M., Ungar W.J. National Database for Autism Research (NDAR): big data opportunities for health services research and health technology assessment. Pharmacoeconomics 2016; 34(2): 127–138, https://doi.org/10.1007/s40273-015-0331-6.
- Fernandez B.A., Scherer S.W. Syndromic autism spectrum disorders: moving from a clinically defined to a molecularly defined approach. Dialogues Clin Neurosci 2017; 19(4): 353–371.
- Masi A., DeMayo M.M., Glozier N., Guastella A.J. An overview of autism spectrum disorder, heterogeneity and treatment options. Neurosci Bull 2017; 33(2): 183–193, https://doi.org/10.1007/s12264-017-0100-y.
- Morozov S.A., Morozova S.S., Morozova T.I. Some of the early help features for children with autism spectrum disorders. Autizm i narusheniya razvitiya 2017; 15(2): 19–31, https://doi.org/10.17759/autdd.2017150202.
- Juárez A.P., Weitlauf A.S., Nicholson A., Pasternak A., Broderick N., Hine J., Stainbrook J.A., Warren Z. Early identification of ASD through telemedicine: potential value for underserved populations. J Autism Dev Disord 2018; 48(8): 2601–2610, https://doi.org/10.1007/s10803-018-3524-y.
- Smith C.J., Rozga A., Matthews N., Oberleitner R., Nazneen N., Abowd G. Investigating the accuracy of a novel telehealth diagnostic approach for autism spectrum disorder. Psychol Assess 2017; 29(3): 245–252, https://doi.org/10.1037/pas0000317.
- Lai M.-C., Lombardo M.V., Baron-Cohen S. Autism. Lancet 2014; 383(9920): 896–910, https://doi.org/10.1016/s0140-6736(13)61539-1.
- Serbina L.F. Investigation of the problem of correction of the mental development of children with ASD using the neuropsychological approach. Vestnik Leningradskogo gosudarstvennogo universiteta imeni A.S. Pushkina 2017; 4: 92–96.
- Tachibana Y., Miyazaki C., Ota E., Mori R., Hwang Y., Kobayashi E., Terasaka A., Tang J., Kamio Y. A systematic review and meta-analysis of comprehensive interventions for pre-school children with autism spectrum disorder (ASD). PLoS One 2017; 12(12): e0186502, https://doi.org/10.1371/journal.pone.0186502.
- Kuder S.J., Accardo A. What works for college students with
autism spectrum disorder. J Autism Dev Disord 2018; 48(3): 722–731, https://doi.org/10.1007/s10803-017-3434-4. - Brondino N., Fusar-Poli L., Rocchetti M.,
Provenzani U., Barale F., Politi P. Complementary and alternative therapies for autism spectrum disorder. Evid Based Complement Alternat Med 2015; 2015: 258589, https://doi.org/10.1155/2015/258589. - LaGasse A.B. Social outcomes in children with autism spectrum disorder: a review of music therapy outcomes. Patient Relat Outcome Meas 2017; 8: 23–32, https://doi.org/10.2147/prom.s106267.
- Chenausky K.V., Schlaug G. From intuition to intervention: developing an intonation-based treatment for autism. Ann N Y Acad Sci 2018; 1423(1): 229–241, https://doi.org/10.1111/nyas.13609.
- Weitlauf A.S., Sathe N., McPheeters M.L., Warren Z.E. Interventions targeting sensory challenges in autism spectrum disorder: a systematic review. Pediatrics 2017; 139(6): e20170347, https://doi.org/10.1542/peds.2017-0347.
- Lee B., Lee J., Cheon J.H., Sung H.K., Cho S.H., Chang G.T. The efficacy and safety of acupuncture for the treatment of children with autism spectrum disorder: a systematic review and meta-analysis. Evid Based Complement Alternat Med 2018; 2018: 1057539, https://doi.org/10.1155/2018/1057539.
- Field T. Massage therapy research review. Complement Ther Clin Pract 2016; 24: 19–31, https://doi.org/10.1016/j.ctcp.2016.04.005.
- Bittner M.D., Rigby B.R., Silliman-French L., Nichols D.L., Dillon S.R. Use of technology to facilitate physical activity in children with autism spectrum disorders: a pilot study. Physiol Behav 2017; 177: 242–246, https://doi.org/10.1016/j.physbeh.2017.05.012.
- Yu C.C.W., Wong S.W.L., Lo F.S.F., So R.C.H., Chan D.F.Y. Study protocol: a randomized controlled trial study on the effect of a game-based exercise training program on promoting physical fitness and mental health in children with autism spectrum disorder. BMC Psychiatry 2018; 18(1): 56, https://doi.org/10.1186/s12888-018-1635-9.
- Lodinova O.A. The game as a method of correction of the emotional development of children with autism. Nauchnyy al’manakh 2017; 6–1(32): 143–146.
- Lau H.M., Smit J.H., Fleming T.M., Riper H. Serious games for mental health: are they accessible, feasible, and effective? A systematic review and meta-analysis. Front Psychiatry 2017; 7: 209, https://doi.org/10.3389/fpsyt.2016.00209.
- Friedrich E.V., Sivanathan A., Lim T., Suttie N., Louchart S., Pillen S., Pineda J.A. An effective neurofeedback intervention to improve social interactions in children with autism spectrum disorder. J Autism Dev Disord 2015; 45(12): 4084–4100, https://doi.org/10.1007/s10803-015-2523-5.
- Arns M., Batail J.M., Bioulac S., Congedo M., Daudet C., Drapier D., Fovet T., Jardri R., Le-Van-Quyen M., Lotte F., Mehler D., Micoulaud-Franchi J.A., Purper-Ouakil D., Vialatte F.; NExT group. Neurofeedback: one of today’s techniques in psychiatry? Encephale 2017; 43(2): 135–145, https://doi.org/10.1016/j.encep.2016.11.003.
- Costa E., Silva J.A., Steffen R.E. The future of psychiatry: brain devices. Metabolism 2017; 69S: S8–S12, https://doi.org/10.1016/j.metabol.2017.01.010.
- Kaplan A.Ya. Neurophysiological foundations and practical realizations of the brain-machine interfaces the technology in neurological rehabilitation. Fiziologiia cheloveka 2016; 42(1): 118–127, https://doi.org/10.7868/s0131164616010100.
- Levitskaya O.S., Lebedev M.A. Brain-computer interface: the future in the present. Bulletin of Russian State Medical University 2016; 2: 4–15, https://doi.org/10.24075/brsmu.2016-02-01.
- Carelli L., Solca F., Faini A., Meriggi P., Sangalli D., Cipresso P., Riva G., Ticozzi N., Ciammola A., Silani V., Poletti B. Brain-computer interface for clinical purposes: cognitive assessment and rehabilitation. Biomed Res Int 2017; 2017: 1695290, https://doi.org/10.1155/2017/1695290.
- Volkova K.V., Dagaev N.I., Kiselev A.S., Kasumov V.R., Aleksandrov M.V., Osadchiy A.E. The brain-computer interface: the experience of building, using, and possible ways to improve performance. Zhurnal vysshei nervnoi deyatelnosti imeni I.P. Pavlova 2017; 67(4): 504–520, https://doi.org/10.7868/s0044467717040128.
- Frolov A.A., Bobrov P.D. Brain-computer interface: neurophysiological background, clinical application. Zhurnal vysshei nervnoi deyatelnosti imeni I.P. Pavlova 2017; 67(4): 365–376, https://doi.org/10.7868/s0044467717040013.
- Renton T., Tibbles A., Topolovec-Vranic J. Neurofeedback as a form of cognitive rehabilitation therapy following stroke: a systematic review. PLoS One 2017; 12(5): e0177290, https://doi.org/10.1371/journal.pone.0177290.
- Sitaram R., Ros T., Stoeckel L., Haller S., Scharnowski F., Lewis-Peacock J., Weiskopf N., Blefari M.L., Rana M., Oblak E., Birbaumer N., Sulzer J. Closed-loop brain training: the science of neurofeedback. Nat Rev Neurosci 2017; 18(2): 86–100, https://doi.org/10.1038/nrn.2016.164.
- Kaplan A.Ya., Kochetova A.G., Shishkin S.L., Basyul I.A., Ganin I.P., Vasilev A.N., Liburkina S.P. Experimental and theoretical foundations and practical implementation of technology brain-computer interface. Bulletin of Siberian Medicine 2013; 12(2): 21–29.
- McFarland D.J., Vaughan T.M. Brain-computer interface (BCI) in practice. Prog Brain Res 2016; 228: 389–404, https://doi.org/10.1016/bs.pbr.2016.06.005.
- Gaume A., Vialatte A., Mora-Sánchez A., Ramdani C., Vialatte F.B. A psychoengineering paradigm for the neurocognitive mechanisms of biofeedback and neurofeedback. Neurosci Biobehav Rev 2016; 68: 891–910, https://doi.org/10.1016/j.neubiorev.2016.06.012.
- Marzbani H., Marateb H.R., Mansourian M. Neurofeedback: a comprehensive review on system design, methodology and clinical applications. Basic Clin Neurosci 2016; 7(2): 143–158, https://doi.org/10.15412/j.bcn.03070208.
- Fedotchev А.I., Parin S.B., Polevaya S.A., Velikova S.D. Brain-computer interface and neurofeedback technologies: current state, problems and clinical prospects (review). Sovremennye tehnologii v medicine 2017; 9(1): 175–184, https://doi.org/10.17691/stm2017.9.1.22.
- Higdon R., Earl R.K., Stanberry L., Hudac C.M., Montague E., Stewart E., Janko I., Choiniere J., Broomall W., Kolker N., Bernier R.A., Kolker E. The promise of multi-omics and clinical data integration to identify and target personalized healthcare approaches in autism spectrum disorders. OMICS 2015; 19(4): 197–208, https://doi.org/10.1089/omi.2015.0020.
- Enriquez-Geppert S., Huster R.J., Herrmann C.S. EEG-neurofeedback as a tool to modulate cognition and behavior: a review tutorial. Front Hum Neurosci 2017; 11: 51, https://doi.org/10.3389/fnhum.2017.00051.
- Hurt E., Arnold L.E., Lofthouse N. Quantitative EEG neurofeedback for the treatment of pediatric attention-deficit/hyperactivity disorder, autism spectrum disorders, learning disorders, and epilepsy. Child Adolesc Psychiatr Clin N Am 2014; 23(3): 465–486, https://doi.org/10.1016/j.chc.2014.02.001.
- Pineda J.A., Carrasco K., Datko M., Pillen S., Schalles M. Neurofeedback training produces normalization in behavioural and electrophysiological measures of high-functioning autism. Philos Trans R Soc Lond B Biol Sci 2014; 369(1644): 20130183, https://doi.org/10.1098/rstb.2013.0183.
- Zivoder I., Martic-Biocina S., Kosic A.V., Bosak J. Neurofeedback application in the treatment of autistic spectrum disorders (ASD). Psychiatr Danub 2015; 27(Suppl 1): S39–S394.
- Wang Y., Sokhadze E.M., El-Baz A.S., Li X., Sears L., Casanova M.F., Tasman A. Relative power of specific EEG bands and their ratios during neurofeedback training in children with autism spectrum disorder. Front Hum Neurosci 2016; 9: 723, https://doi.org/10.3389/fnhum.2015.00723.
- Cheung S., Han E., Kushki A., Anagnostou E., Biddiss E. Biomusic: an auditory interface for detecting physiological indicators of anxiety in children. Front Neurosci 2016; 10: 401, https://doi.org/10.3389/fnins.2016.00401.
- Fedotchev A.I., Radchenko G.S. Music therapy and “brain music” state of the art, problems and perspectives problems and perspectives. Uspekhi fiziologicheskikh nauk 2013; 44(4): 35–50.
- Konstantinov K.V., Leonova M.K., Miroshnikov D.B., Klimenko V.M. Specifics of perception of acoustic image of intrinsic bioelectric brain activity. Rossiiskii fiziologicheskii zhurnal imeni I.M. Sechenova 2014; 100(6): 710–721.
- Bergstrom I., Seinfeld S., Arroyo-Palacios J., Slater M., Sanchez-Vives M.V. Using music as a signal for biofeedback. Int J Psychophysiol 2014; 93(1): 140–149, https://doi.org/10.1016/j.ijpsycho.2013.04.013.
- Gui K., Liu H., Zhang D. Towards multimodal human-robot interaction to enhance active participation of users in gait rehabilitation. IEEE Trans Neural Syst Rehabil Eng 2017; 25(11): 2054–2066, https://doi.org/10.1109/tnsre.2017.2703586.
- Choi I., Rhiu I., Lee Y., Yun M.H., Nam C.S. A systematic review of hybrid brain-computer interfaces: taxonomy and usability perspectives. PLoS One 2017; 12(4): e0176674, https://doi.org/10.1371/journal.pone.0176674.
- Hong K.S., Khan M.J. Hybrid brain-computer interface techniques for improved classification accuracy and increased number of commands: a review. Front Neurorobot 2017; 11: 35, https://doi.org/10.3389/fnbot.2017.00035.
- Fedotchev A.I., Oh S.J., Semikin G.I. Combination of neurofeedback technique with music therapy for effective correction of stress-induced disorders. Sovremennye tehnologii v medicine 2014; 6(3): 60–63.
- Fedotchev A.I., Bondar A.T., Bakhchina A.V., Grigorieva V.N., Katayev A.A., Parin S.B., Radchenko G.S., Polevaya S.A. Transformation of patient’s EEG oscillators into music-like signals for correction of stress-induced functional states. Sovremennye tehnologii v medicine 2016; 8(1): 93–98, https://doi.org/10.17691/stm2016.8.1.12.
- Fedotchev A.I., Bondar’ A.T., Bakhchina A.V., Parin S.B., Polevaya S.A., Radchenko G.S. Music-acoustic signals controlled by subject’s brain potentials in the correction of unfavorable functional states. Uspekhi fiziologicheskikh nauk 2016; 47(1): 69–79.
- Fedotshev A.I. Stress, the consequences of its influence on humans and modern non-drug methods of stress-induced states reduction. Uspekhi fiziologicheskikh nauk 2009; 40(1): 77–91.
- Oh S.J., Semikin G.I., Fedotchev A.I. Utilization of forward and backward interactions in human-machine system for elimination of specialist’s functional reliability risks. Zivaa psihologia 2015; 2(4): 291–300, https://doi.org/10.18334/lp.2.4.35130.
- Fedotchev A.I., Zemlyanaya A.A., Polevaya S.A., Savchuk L.V. Attention deficit hyperactivity disorder and current possibilities of its treatment by the method of neurofeedback training. Zhurnal nevrologii i psihiatrii imeni S.S. Korsakova 2016; 116(5): 98–101, https://doi.org/10.17116/jnevro20161165198-101.
- Zemlyanaya A.A., Fedotchev A.I. Individual approaches to diagnosis and treatment of epilepsia (review). Sovremennye tehnologii v medicine 2018; 10(3): 204–212, https://doi.org/10.17691/stm2018.10.3.25.
- Friedrich E.V., Suttie N., Sivanathan A., Lim T., Louchart S., Pineda J.A. Brain-computer interface game applications for combined neurofeedback and biofeedback treatment for children on the autism spectrum. Front Neuroeng 2014; 7: 21, https://doi.org/10.3389/fneng.2014.00021.
- Fedotchev A.I., Zhuravlev G.I., Eksina K.I., Silantieva O.M., Polevaya S.A. Evaluation of efficiency of musical EEG neurointerface with additional control contour from heart rhythm. Rossiiskii fiziologicheskii zhurnal imeni I.M. Sechenova 2018; 104(1): 122–128.