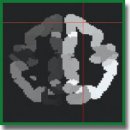
Towards Semantic Brain Mapping Methodology Based on a Multidimensional Markup of Continuous Russian-Language Texts: an Attempt at Validation and Development
In the present study, we combine linguistic annotation of oral texts in Russian with the registration of BOLD signal in functional MRI experiments to determine how and where semantic categories are represented in the human brain. Using the same stimuli material, we also analyze the differences in cortical activation in three thematic domains: description of nature, description of working principles of technical devices and more self-referential texts, addressing the question of human identity in conflict situations. We discuss methodological problems within the two approaches (microanalysis and macroanalysis) to study brain activation in natural conditions, i.e. under a continuous speech flow. Within the thematic domain studies, only minimally significant differences in brain activation were registered during the listening to texts from the three thematic groups. This outcome leads to the conclusion that the approach of thematic group contrasts (cognitive subtraction methodology) is not sufficient to study the mechanisms of text comprehension, and should be replaced by the modeling of multidimensional representations of semantic categories in time. Within the semantic category approach, we describe the neurolinguistic process of text understanding as the activation of 15 clusters responsible for semantic categories (e.g. “Conflict”, “Mental”, “Social”). Our data demonstrate that the clusters are widely distributed across the human brain. In contrast to the previous studies, we suggest that deep subcortical structures are involved in the processing of certain categories as well. The observed lateralization of category processing underlines the involvement of the right hemisphere in the processing of meaning.
- Standing L. Learning 10000 pictures. Q J Exp Psychol 1973; 25(2): 207–222, https://doi.org/10.1080/14640747308400340.
- Velichkovsky B.M. Visual cognition and its spatiotemporal context. In: Klix F., Hoffmann J., van der Meer E. (editors). Cognitive research in psychology. North Holland, Amsterdam; 1982; p. 63–79.
- Brady T.F., Konkle T., Alvarez G.A., Oliva A. Visual long-term memory has a massive storage capacity for object details. Proc Natl Acad Sci U S A 2008; 105(38): 14325–14329, https://doi.org/10.1073/pnas.0803390105.
- Warrington E.K. The selective impairment of semantic memory. Q J Exp Psychol 1975; 27(4): 635–657, https://doi.org/10.1080/14640747508400525.
- Huth A.G., de Heer W.A., Griffiths T.L., Theunissen F.E., Gallant J.L. Natural speech reveals the semantic maps that tile human cerebral cortex. Nature 2016; 532(7600): 453–458, https://doi.org/10.1038/nature17637.
- Ushakov V.L., Orlov V.A., Kartashov S.I., Malakhov D.G., Korosteleva A.N., Skiteva L.I., Zaidelman L.Ya., Zinina A.A., Zabotkina V.I., Velichkovsky B.M., Kotov A.A. Contrasting human brain responses to literature descriptions of nature and to technical instructions. In: Kryzhanovsky B., Dunin-Barkowski W., Redko V., Tiumentsev Y. (editors). Advances in neural computation, machine learning, and cognitive research II. Neuroinformatics 2018. Studies in Computational Intelligence. Vol. 799. Springer, Cham; 2018; p. 284–290, https://doi.org/10.1007/978-3-030-01328-8_34.
- Friston K.J. Imaging neuroscience: principles or maps? Proc Natl Acad Sci U S A 1998; 95(3): 796–802, https://doi.org/10.1073/pnas.95.3.796.
- Friston K.J., Price C.J., Fletcher P., Moore C., Frackowiak R.S., Dolan R.J. The trouble with cognitive subtraction. Neuroimage 1996; 4(2): 97–104, https://doi.org/10.1006/nimg.1996.0033.
- Korobov M. Morphological analyzer and generator for Russian and Ukrainian languages. In: Khachay M., Konstantinova N., Panchenko A., Ignatov D., Labunets V. (editors). Analysis of images, social networks and texts. AIST 2015. Communications in computer and information science. Vol. 542. Springer, Cham; 2015; p. 320–332, https://doi.org/10.1007/978-3-319-26123-2_31.
- Mikolov T., Chen K., Corrado G., Dean J. Efficient estimation of word representations in vector space. In: Proceedings of the 1st International Conference on Learning Representations (ICLR 2013). USA; 2013; p. 1–12.
- Kutuzov A., Kuzmenko E. WebVectors: a toolkit for building web interfaces for semantic vector models. In: Analysis of images, social networks and texts. AIST 2016. Communications in computer and information science. Vol. 661. Springer, Cham; 2017; p. 155–161, https://doi.org/10.1007/978-3-319-52920-2_15.
- Lyashevskaya O.N., Sharov S.A. Chastotnyy slovar’ sovremennogo russkogo yazyka (na materialakh Natsional’nogo korpusa russkogo yazyka) [A frequency dictionary of contemporary Russian (based on the materials of the Russian National Corpus)]. Moscow: Azbukovnik; 2009.
- Marsman J.B., Renken R., Velichkovsky B.M., Hooymans J.M.M., Cornelissen F.W. Fixation based event-related (FIBER) analysis: using eye fixations as events in functional magnetic resonance imaging (fMRI) to reveal cortical processing during the free exploration of visual images. Hum Brain Mapp 2012; 33(2): 307–318, https://doi.org/10.1002/hbm.21211.
- Velichkovsky B.M., Korosteleva A.N., Pannasch S., Helmert J.R., Orlov V.A., Sharaev M.G., Velichkovsky B.B., Ushakov V.L. Two visual systems and their eye movements: a fixation-based event-related experiment with ultrafast fMRI reconciles competing views. Sovremennye tehnologii v medicine 2019; 11(4): 7–18, https://doi.org/10.17691/stm2019.11.4.01.
- Kerestes R., Chase H.W., Phillips M.L., Ladouceur C.D., Eickhoff S.B. Multimodal evaluation of the amygdala’s functional connectivity. Neuroimage 2017; 148: 219–229, https://doi.org/10.1016/j.neuroimage.2016.12.023.
- Aquino K.M., Schira M.M., Robinson P.A., Drysdale P.M., Breakspear M. Hemodynamic traveling waves in human visual cortex. PLoS Comput Biol 2012; 8(3): e1002435, https://doi.org/10.1371/journal.pcbi.1002435.
- Alexander D.M., Trengove C., van Leeuwen C. Donders is dead: cortical traveling waves and the limits of mental chronometry in cognitive neuroscience. Cogn Process 2015; 16(4): 365–375, https://doi.org/10.1007/s10339-015-0662-4.
- Verkhlyutov V.M., Balaev V.V., Ushakov V.L., Velichkovsky B.M. A novel methodology for simulation of EEG traveling waves on the folding surface of the human cerebral cortex. In: Kryzhanovsky B., Dunin-Barkowski W., Redko V., Tiumentsev Y. (editors). Advances in neural computation, machine learning, and cognitive research II. Neuroinformatics 2018. Studies in computational intelligence. Vol. 799. Springer, Cham; 2019; p. 51–63, https://doi.org/10.1007/978-3-030-01328-8_4.
- Poldrack R.A., Yarkoni T. From brain maps to cognitive ontologies: informatics and the search for mental structure. Annu Rev Psychol 2016; 67: 587–612, https://doi.org/10.1146/annurev-psych-122414-033729.
- Nastase S.A., Gazzola V., Hasson U., Keysers C. Measuring shared responses across subjects using intersubject correlation. Soc Cogn Affect Neurosci 2019; 14(6): 667–685, https://doi.org/10.1093/scan/nsz037.
- Shailee J., Huth A.G. Incorporating context into language encoding models for fMRI. In: Proceeding of the 32nd Conference on Neural Information Processing Systems (NeurIPS). Canada; 2018; p. 6629–6638, https://doi.org/10.1101/327601.
- Hamilton L.S., Huth A.G. The revolution will not be controlled: natural stimuli in speech neuroscience. Lang Cogn Neurosci 2018; 1–10, https://doi.org/10.1080/23273798.2018.1499946.
- Ojemann G.A. Models of the brain organization for higher integrative functions derived with electrical stimulation techniques. Hum Neurobiol 1982; 1(4): 243–249.
- Fodor J.A. Modularity of mind. Cambridge, MA: MIT Press; 1985.
- Velichkovsky B.M. Neurosemanics — new direction of interdisciplinary cognitive research. Voprosy Psychologii 2019; 64(6): 3–18.