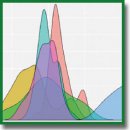
Technology of Informative Feature Selection for Immunosignature Analysis
The main difficulty in practical work with data obtained via immunosignature analysis is high dimensionality and the presence of a significant number of uninformative or false-informative features due to the specific character of the technology. To ensure practically relevant quality of data analysis and classification, it is necessary to take due account of this specific character.
The aim of the study is to create and test the technology for effective reduction of immunosignature data dimensionality, which provides practically relevant and high quality of classification with due regard for the properties of the data obtained.
Materials and Methods. The study involved the use of two normalized data sets obtained from the public biomedical repository and containing the results of immunosignature analysis.
The technology for selecting informative features was proposed within the framework of the study. It consisted of three successive steps: 1) breaking a multiclass task into a series of binary tasks using the “one vs all” strategy; 2) screening of false-informative features is performed for each binary comparison by comparing the values of the median of the sets “one” and “all”; 3) ranking of the remaining features according to their informative value and selection of the most informative ones for each binary comparison.
To assess the quality of the proposed technology for informative feature selection, we used the results obtained after application of classification based on the filtered data. Support vector method that proved itself in the problems of high-dimensional data classification was used as a classification model.
Results. Effectiveness of the proposed technology for informative feature selection was determined. This technology allows us to provide high quality of classification while significantly reducing the feature space. The number of features eliminated in the second step is approximately 50% for each data set under consideration, which greatly simplifies subsequent data analysis. After the third step, when the feature space is reduced to 15 features, the quality of classification by the macro-average F1-score metric is assessed as 98.9% for the GSE52581 dataset. For the GSE52581 dataset, with the feature space reduced to 266 features, the quality of classification by the macro-average F1-score metric is 91.3%.
Conclusion. The results of the work demonstrate the promising outlook of the proposed technology for informative feature selection as applied to the data of immunosignature analysis.
- Zlokachestvennye novoobrazovaniya v Rossii v 2018 godu (zabolevaemost’ i smertnost’) [Malignant neoplasms in Russia in 2018 (morbidity and mortality)]. Pod red. Kaprina A.D., Starinskogo V.V., Petrovoy G.V. [Kaprin A.D., Starinskiy V.V., Petrova G.V. (editors)]. Moscow: MNIOI im. P.A. Gertsena — filial FGBU “NMITs radiologii” Minzdrava Rossii; 2019; 250 p.
- World Health Organization. Guide to cancer early diagnosis. World Health Organization; 2017. URL: https://apps.who.int/iris/bitstream/handle/ 10665/254500/9789241511940%20eng.pdf;jsessionid= F414948FB143C37513D7C21E675BA9C8?sequence=1.
- Stafford P., Halperin R., Legutki J.B., Magee D.M., Galgiani J., Johnston S.A. Physical characterization of the “immunosignaturing effect”. Mol Cell Proteomics 2012; 11(4): M111.011593, https://doi.org/10.1074/mcp.m111.011593.
- Blum A.L., Langley P. Selection of relevant features and examples in machine learning. Artificial Intelligence 1997; 97(1–2): 245–271, https://doi.org/10.1016/s0004-3702(97)00063-5.
- Kukreja M., Johnston S.A., Stafford P. Immunosignaturing microarrays distinguish antibody profiles of related pancreatic diseases. J Proteomics Bioinform 2013; S6(1): 1–5, https://doi.org/10.4172/jpb.s6-001.
- Stafford P., Cichacz Z., Woodbury N.W., Johnston S.A. Immunosignature system for diagnosis of cancer. Proc Natl Acad Sci U S A 2014; 111(30): E3072–E3080, https://doi.org/10.1073/pnas.1409432111.
- Anisimov D.S., Podlesnykh S.V., Kolosova E.A., Shcherbakov D.N., Petrova V.D., Dzhonston S.A., Lazarev A.F., Oskorbin N.M., Shapoval A.I., Ryazanov M.A. Projection to latent structures as a strategy for peptides microarray data analysis. Matematicheskaa biologia i bioinformatika 2017; 12(2): 435–445, https://doi.org/10.17537/2017.12.435.
- Subramanian J., Simon R. Overfitting in prediction models — is it a problem only in high dimensions. Contemp Clin Trials 2013; 36(2): 636–641, https://doi.org/10.1016/j.cct.2013.06.011.
- Stafford P., Zbigniew C., Johnston S. An immunosignature system for diagnosis of cancer [Cancer immunosignaturing — test 1]. National Center for Biotechnology Information Search database; 2013. URL: https://www.ncbi.nlm.nih.gov/geo/query/acc.cgi?acc=GSE52580.
- Stafford P., Zbigniew C., Johnston S. An immunosignature system for diagnosis of cancer [Cancer immunosignaturing — test 2]. National Center for Biotechnology Information Search database; 2013. URL: https://www.ncbi.nlm.nih.gov/geo/query/acc.cgi?acc=GSE52581.
- Wickham H. Tidy data. J Stat Softw 2014; 59(10), https://doi.org/10.18637/jss.v059.i10.
- Izetta J., Verdes P.F., Granitto P.M. Improved multiclass feature selection via list combination. Expert Syst Appl 2017; 88: 205–215, https://doi.org/10.1016/j.eswa.2017.06.043.
- Bommert A., Sun X., Bischl B., Rahnenführer J., Lang M. Benchmark for filter methods for feature selection in high-dimensional classification data. Computational Statistics & Data Analysis 2020; 143: 106839, https://doi.org/10.1016/j.csda.2019.106839.
- Shannon C.E. A mathematical theory of communication. Bell System Technical Journal 1948; 27(3): 379–423, https://doi.org/10.1002/j.1538-7305.1948.tb01338.x.
- Andryushchenko V.S., Uglov A.S., Zamyatin A.V. Statistical classification of immunosignatures under significant reduction of the feature space dimensions for early diagnosis of diseases. Sovremennye tehnologii v medicine 2018; 10(3): 14–20, https://doi.org/10.17691/stm2018.10.3.2.
- Cortes C., Vapnik V. Support-vector networks. Mach Learn 1995; 20(3): 273–297, https://doi.org/10.1007/BF00994018.
- Powers D. Evaluation: from precision, recall and F-factor to ROC, informedness, markedness & correlation. J Mach Learn Tech 2007; 2: 37–63.