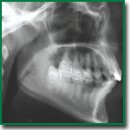
Technology for High-Sensitivity Analysis of Medical Diagnostic Images
Control and analysis of small, inaccessible to human vision changes in medical images make it possible to focus on diagnostically important radiological signs important for the correct diagnosis.
The aim of the study was to develop information technology facilitating the early diagnosis of diseases using medical images.
Materials and Methods. To control changes in the image, we used its transformation based on solving a particular case of the knapsack problem. The proposed transformation is highly sensitive to any changes in the image and provides the possibility to record deviations visually with high accuracy. Medical images were obtained using cone beam computed tomography.
Results. Practical evaluation of the information technology on tomograms showed the following: the transformed images of healthy bone tissue fragments from different parts of the jaw have similar shapes and nearly the same range of brightness. The transformed image of bone tissue after treatment has a shape close to that of the transformed image of healthy bone tissue. The transformed image of the affected bone tissue has a shape and brightness range differing from the shape and color of the transformed images of healthy bone tissue and bone tissue after treatment. However, transformation of medical images obtained with the Planmeca ProMax 3D Classic device (Finland) allows recording changes that account for less than 0.0001% of the entire image.
Conclusion. The proposed method allows human vision to capture changes as small as nearly one pixel in the transformed image, which is impossible with the original medical image. Increasing the color contrast of the transformed medical image makes it possible to reveal the structure of the analyzed medical image fragment. The proposed image transformation method can be used for early diagnosis of diseases and in other fields of medicine.
- Yavna D.V., Babenko V.V. A psychophysiologically sound method for assessing the amount of information in an image. Inzenernyj vestnik Dona 2014; 4: 1–13.
- Applegate R.A., Thibos L.N., Hilmantel G. Optics of aberroscopy and super vision. J Cataract Refract Surg 2001; 27(7): 1093–1107, https://doi.org/10.1016/s0886-3350(01)00856-2.
- Petrov I.B. Mathematical modeling in medicine and biology by medium mechanics models. Proc MIPT 2009; 1(1): 5–16.
- Voropayeva O.F., Shokin Yu.I. Numerical simulation in medicine: formulations of the problems and some results of calculations. Vycislitelʹnye tehnologii 2012; 17(4): 29–55.
- Beklemysheva K.A., Vasyukov A.V., Petrov I.B. Numerical simulation of dynamic processes in biomechanics using the grid-characteristic method. Computational Mathematics and Mathematical Physics 2015; 55(8): 1346–1355, https://doi.org/10.7868/s0044466915080049.
- Parmar C., Barry J.D., Hosny A., Quackenbush J., Aerts H.J.W.L. Data analysis strategies in medical imaging. Clin Cancer Res 2018; 24(15): 3492–3450, https://doi.org/10.1158/1078-0432.ccr-18-0385.
- Eardi L., Aston J.A.D. Statistical analysis of functions on surfaces, with an application to medical imaging. J Am Stat Assoc 2019; 115: 1420–1434, https://doi.org/10.1080/01621459.2019.1635479.
- Saturi R., Dara R., Chand P.P. Extracting subset of relevant features for breast cancer to improve accuracy of classifier. IJITEE 2019; 8(11): 1670–1674, https://doi.org/10.35940/ijitee.k1507.0981119.
- Sinha A., Dolz J. Multi-scale guided attention for medical image segmentation. IEEE J Biomed Health Inform 2021; 25(1): 121–130, https://doi.org/10.1109/jbhi.2020.2986926.
- Zimichev E.A., Kazanskiy N.L., Serafimovich P.G. Spectral-spatial classification with k-means++ particional clustering. Computer Optics 2014; 38(2): 281–286.
- Yuan Y., Lo Y.C. Automatic skin lesion segmentation with fully convolutional-deconvolutional networks. IEEE J Biomed Health Inform 2019; 23(2): 519–526, https://doi.org/10.1109/jbhi.2017.2787487.
- Song X., Wu L., Liu G. Unsupervised color texture segmentation based on multi-scale region-level Markov random field models. Comput Opt 2019; 43(2): 264–269.
- Zakani F.R., Bouksim M., Khadija A., Aboulfatah M., Gadi T. Segmentation of 3D meshes combining the artificial neural network classifier and the spectral clustering. Comput Opt 2018; 42(2): 312–319.
- Abul’khanov S.R., Kazanskiy N.L. Information pattern in imaging of a rough surface. IOP Conference Series: Materials Science and Engineering 2018; 302: 012068, https://doi.org/10.1088/1757-899x/302/1/012068.
- Martello S., Toth P. Knapsack problems. Chichester: John Wiley and Sons Ltd; 1990.
- Moon J.W., Moser L. Some packing and covering theorems. Colloq Math 1967; 17: 103–110.
- Kazanskiy N., Kuznetsov M. The necessary bound of rectangle’s square for packing into this any system of five and more than five finite quantity squares with total area 1. Procedia Eng 2017; 201: 801–805, https://doi.org/10.1016/j.proeng.2017.09.601.
- Honsberger R. Mathematical morsels. Washington: Mathematical association of America; 1978.
- Slesarev O., Bayricov I., Trunin D., Abul’khanov S., Kazanskiy N. Influence of data visualization of temporomandibular joint on the formation of clinical groups. RAD Conference Proceedings 2017; 2: 212–216, https://doi.org/10.21175/radproc.2017.43.
- Jones H.A., Langmur I., Mackay G.M.J. The rates of evaporation and the vapor pressures of tungsten, molybdenum, platinum, nickel, iron, copper and silver. Phys Rev 1927; 30(2): 201–214, https://doi.org/10.1103/physrev.30.201.
- Wilson A.D. Tungsten filament life under constant-current heating. J Appl Phys 1969; 40(4): 1956–1964, https://doi.org/10.1063/1.1657874.
- Valstar E.R., Nelissen R.G.H.H., Reiber J.H.C., Rozing P.M. The use of Roentgen stereophotogrammetry to study micromotion of orthopaedic implants. ISPRS J Photogramm Remote Sens 2002; 56(5–6): 376–389, https://doi.org/10.1016/s0924-2716(02)00064-3.
- Ilyasova N.Yu., Shirokanev A.S., Kupriyanov A.V., Paringer R.A. Technology of intellectual feature selection for a system of automatic formation of a coagulate plan on retina. Computer Optics 2019; 43(2): 304–315.
- Grupp R.B., Murphy R.J., Hegeman R.A., Alexander C.P., Unberath M., Otake Y., McArthur B.A., Armand M., Taylor R.H. Fast and automatic periacetabular osteotomy fragment pose estimation using intraoperatively implanted fiducials and single-view fluoroscopy. Phys Med Biol 2020; 65: 245019, https://doi.org/10.1088/1361-6560/aba089.
- Polyarush N.F., Slesarev O.V., Polyarush M.V. The method of layer-by-layer imaging of the temporomandibular joint. Patent RU 2177722. 2002.
- Slesarev O.V. Anatomic rationale and radiological experience in using an individual anatomical landmark during linear imaging of the human temporomandibular joint. Vestnik rentgenologii i radiologii 2014; 3: 46–51.
- Shahidi R., Lorensen B., Kikinis R., Flynn J., Kaufman A.E., Napel S. Surface rendering versus volume rendering in medical imaging: techniques and applications. Proc 7th Annual IEEE Visualiz ‘96 1996: 439–440, https://doi.org/10.1109/visual.1996.568151.
- Tomlinson S.B., Hendricks B.K., Cohen-Gadol A. Immersive three-dimensional modeling and virtual reality for enhanced visualization of operative neurosurgical anatomy. World Neurosurg 2019; 131: 313–320, https://doi.org/10.1016/j.wneu.2019.06.081.
- Al-Temimi A.M.S., Pilidi V.S. Improvements of programing methods for finding reference lines on X-Ray images. Comput Opt 2019; 43(3): 397–401, https://doi.org/10.18287/2412-6179-2019-43-3-397-401.
- Vasilchenko V.A., Burkovskiy V.L., Danilov A.D. Algorithmization of the process of recognition of states of living objects based on special X-ray images. Computer Optics 2019; 43(2): 296–303.
- Gaidel A.V., Kapishnikov A.V., Pyshkina Yu.S., Kolsanov A.V., Khramov A.G. Method of nephroscintigraphic dynamic images analysis. Computer Optics 2018; 42(4): 688–694.
- Kazanskiy N.L., Popov S.B. Machine vision system for singularity detection in monitoring the long process. Optical Memory and Neural Networks (Information Optics) 2010; 19(1): 23–30, https://doi.org/10.3103/s1060992x10010042.
- Mikhaylichenko А.A., Demyanenko Y.М. Detection of the bone contours of the knee joints on medical X-ray images. Computer Optics 2019; 43(3): 455–463, https://doi.org/10.18287/2412-6179-2019-43-3-455-463.
- Kazanskiy N.L., Khonina S.N., Butt M.A. Plasmonic sensors based on Metal-insulator-metal waveguides for refractive index sensing applications: a brief review. Physica E Low Dimens Syst Nanostruct 2020; 117: 113798, https://doi.org/10.1016/j.physe.2019.113798.
- Jędrzejewska-Szczerska M., Karpienko K., Wróbel M.S., Tuchin V.V. Sensors for rapid detection of environmental toxicity in blood of poisoned people. In: Nikolelis D.P., Nikoleli G.P. (editors) Biosensors for security and bioterrorism applications. Switzerland: Springer International Publishing; 2016; p. 413–430, https://doi.org/10.1007/978-3-319-28926-7_19.
- Kazanskiy N.L., Butt M.A., Degtyarev S.A., Khonina S.N. Achievements in the development of plasmonic waveguide sensors for measuring the refractive index. Computer Optics 2020; 44(3): 295–318.
- Ebrahimi A., Scott J., Ghorbani K. Microwave reflective biosensor for glucose level detection in aqueous solutions. Sens Actuator A Phys 2019; 301: 111662, https://doi.org/10.1016/j.sna.2019.111662.
- Govind G., Akhtar M.J. Metamaterial-inspired microwave microfluidic sensor for glucose monitoring in aqueous solutions. IEEE Sens J 2019; 19(24): 11900–11907, https://doi.org/10.1109/jsen.2019.2938853.