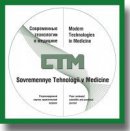
Radiomics and Digital Image Texture Analysis in Oncology (Review)
One of the most promising areas of diagnosis and prognosis of diseases is radiomics, a science combining radiology, mathematical modeling, and deep machine learning. The main concept of radiomics is image biomarkers (IBMs), the parameters characterizing various pathological changes and calculated based on the analysis of digital image texture. IBMs are used for quantitative assessment of digital imaging results (CT, MRI, ultrasound, PET). The use of IBMs in the form of “virtual biopsy” is of particular relevance in oncology.
The article provides the basic concepts of radiomics identifying the main stages of obtaining IBMs: data collection and preprocessing, tumor segmentation, data detection and extraction, modeling, statistical processing, and data validation. The authors have analyzed the possibilities of using IBMs in oncology, describing the currently known features and advantages of using radiomics and image texture analysis in the diagnosis and prognosis of cancer. The limitations and problems associated with the use of radiomics data are considered.
Although the novel effective tool for performing virtual biopsy of human tissue is at the development stage, quite a few projects have already been implemented, and medical software packages for radiomics analysis of digital images have been created.
- Avanzo M., Stancanello J., El Naqa I. Beyond imaging: the promise of radiomics. Phys Med 2017; 38: 122–139, https://doi.org/10.1016/j.ejmp.2017.05.071.
- Liu Z., Wang S., Dong D., Wei J., Fang C., Zhou X., Sun K., Li L., Li B., Wang M., Tian J. The applications of radiomics in precision diagnosis and treatment of oncology: opportunities and challenges. Theranostics 2019; 9(5): 1303–1322, https://doi.org/10.7150/thno.30309.
- Ognerubov N.A., Shatov I.A., Shatov A.V. Radiogenomics and radiomics in the diagnostics of malignant tumours: a literary review. Vestnik Tambovskogo universiteta 2017; 22(6–2): 1453–1460, https://doi.org/10.20310/1810-0198-2017-22-6-1453-1460.
- Lambin P., Leijenaar R.T.H., Deist T.M., Peerlings J., de Jong E.E.C., van Timmeren J., Sanduleanu S., Larue R.T.H.M., Even A.J.G., Jochems A., van Wijk Y., Woodruff H., van Soest J., Lustberg T., Roelofs E., van Elmpt W., Dekker A., Mottaghy F.M., Wildberger J.E., Walsh S. Radiomics: the bridge between medical imaging and personalized medicine. Nat Rev Clin Oncol 2017; 14(12): 749–762, https://doi.org/10.1038/nrclinonc.2017.141.
- Song J., Yin Y., Wang H., Chang Z., Liu Z., Cui L. A review of original articles published in the emerging field of radiomics. Eur J Radiol 2020; 127: 108991, https://doi.org/10.1016/j.ejrad.2020.108991.
- Kumar V., Gu Y., Basu S., Berglund A., Eschrich S.A., Schabath M.B., Forster K., Aerts H.J., Dekker A., Fenstermacher D., Goldgof D.B., Hall L.O., Lambin P., Balagurunathan Y., Gatenby R.A., Gillies R.J. Radiomics: the process and the challenges. Magn Reson Imaging 2012; 30(9): 1234–1248, https://doi.org/10.1016/j.mri.2012.06.010.
- Ramón Y Cajal S., Sesé M., Capdevila C., Aasen T., De Mattos-Arruda L., Diaz-Cano S.J., Hernández-Losa J., Castellví J. Clinical implications of intratumor heterogeneity: challenges and opportunities. J Mol Med (Berl) 2020; 98(2): 161–177, https://doi.org/10.1007/s00109-020-01874-2.
- Mirestean C.C., Pagute O., Buzea C., Iancu R.I., Iancu D.T. Radiomic machine learning and texture analysis — new horizons for head and neck oncology. Maedica (Bucur) 2019; 14(2): 126–130.
- Giganti F., Antunes S., Salerno A., Ambrosi A., Marra P., Nicoletti R., Orsenigo E., Chiari D., Albarello L., Staudacher C., Esposito A., Del Maschio A., De Cobelli F. Gastric cancer: texture analysis from multidetector computed tomography as a potential preoperative prognostic biomarker. Eur Radiol 2017; 27(5): 1831–1839, https://doi.org/10.1007/s00330-016-4540-y.
- Lubner M.G., Smith A.D., Sandrasegaran K., Sahani D.V., Pickhardt P.J. CT texture analysis: definitions, applications, biologic correlates, and challenges. Radiographics 2017; 37(5): 1483–1503, https://doi.org/10.1148/rg.2017170056.
- Limkin E.J., Sun R., Dercle L., Zacharaki E.I., Robert C., Reuzé S., Schernberg A., Paragios N., Deutsch E., Ferté C. Promises and challenges for the implementation of computational medical imaging (radiomics) in oncology. Ann Oncol 2017; 28(6): 1191–1206, https://doi.org/10.1093/annonc/mdx034.
- Yip S.S.F., Aerts H.J.W.L. Applications and limitations of radiomics. Phys Med Biol 2016; 61(13): R150–R166, https://doi.org/10.1088/0031-9155/61/13/r150.
- Neri E., Del Re M., Paiar F., Erba P., Cocuzza P., Regge D., Danesi R. Radiomics and liquid biopsy in oncology: the holons of systems medicine. Insights Imaging 2018; 9(6): 915–924, https://doi.org/10.1007/s13244-018-0657-7.
- Gardin I., Grégoire V., Gibon D., Kirisli H., Pasquier D., Thariat J., Vera P. Radiomics: principles and radiotherapy applications. Crit Rev Oncol Hematol 2019; 138: 44–50, https://doi.org/10.1016/j.critrevonc.2019.03.015.
- Chaddad A., Kucharczyk M.J., Daniel P., Sabri S., Jean-Claude B.J., Niazi T., Abdulkarim B. Radiomics in glioblastoma: current status and challenges facing clinical implementation. Front Oncol 2019; 9: 374, https://doi.org/10.3389/fonc.2019.00374.
- Ng F., Kozarski R., Ganeshan B., Goh V. Assessment of tumor heterogeneity by CT texture analysis: can the largest cross-sectional area be used as an alternative to whole tumor analysis? Eur J Radiol 2013; 82(2): 342–348, https://doi.org/10.1016/j.ejrad.2012.10.023.
- Ibrahim A., Primakov S., Beuque M., Woodruff H.C., Halilaj I., Wu G., Refaee T., Granzier R., Widaatalla Y., Hustinx R., Mottaghy F.M., Lambin P. Radiomics for precision medicine: сurrent challenges, future prospects, and the proposal of a new framework. Methods 2020; S1046-2023(20)30111-0, https://doi.org/10.1016/j.ymeth.2020.05.022.
- Koçak B., Durmaz E.Ş., Ateş E., Kılıçkesmez Ö. Radiomics with artificial intelligence: a practical guide for beginners. Diagn Interv Radiol 2019; 25(6): 485–495, https://doi.org/10.5152/dir.2019.19321.
- Bashir U., Siddique M.M., Mclean E., Goh V., Cook G.J. Imaging heterogeneity in lung cancer: techniques, applications, and challenges. AJR Am J Roentgenol 2016; 207(3): 534–543, https://doi.org/10.2214/ajr.15.15864.
- Galavis P.E., Hollensen C., Jallow N., Paliwal B., Jeraj R. Variability of textural features in FDG PET images due to different acquisition modes and reconstruction parameters. Acta Oncol 2010; 49(7): 1012–1016, https://doi.org/10.3109/0284186x.2010.498437.
- Mackin D., Fave X., Zhang L., Fried D., Yang J., Taylor B., Rodriguez-Rivera E., Dodge C., Jones A.K., Court L. Measuring computed tomography scanner variability of radiomics features. Invest Radiol 2015; 50(11): 757–765, https://doi.org/10.1097/rli.0000000000000180.
- Zhao B., Tan Y., Tsai W.Y., Qi J., Xie C., Lu L., Schwartz L.H. Reproducibility of radiomics for deciphering tumor phenotype with imaging. Sci Rep 2016; 6: 23428, https://doi.org/10.1038/srep23428.
- Oliver J.A., Budzevich M., Zhang G.G., Dilling T.J., Latifi K., Moros E.G. Variability of Image features computed from conventional and respiratory-gated PET/CT images of lung cancer. Transl Oncol 2015; 8(6): 524–534, https://doi.org/10.1016/j.tranon.2015.11.013.
- Leijenaar R.T.H., Carvalho S., Velazquez E.R., van Elmpt W.J.C., Parmar C., Hoekstra O.S., Hoekstra C.J., Boellaard R., Dekker A.L., Gillies R.J., Aerts H.J., Lambin P. Stability of FDG-PET radiomics features: an integrated analysis of test-retest and inter-observer variability. Acta Oncol 2013; 52(7): 1391–1397, https://doi.org/10.3109/0284186x.2013.812798.
- Tixier F., Hatt M., Le Rest C.C., Le Pogam A., Corcos L., Visvikis D. Reproducibility of tumor uptake heterogeneity characterization through textural feature analysis in 18F-FDG PET. J Nucl Med 2012; 53(5): 693–700, https://doi.org/10.2967/jnumed.111.099127.
- Guezennec C., Bourhis D., Orlhac F., Robin P., Corre J.B., Delcroix O., Gobel Y., Schick U., Salaün P.Y., Abgral R. Inter-observer and segmentation method variability of textural analysis in pre-therapeutic FDG PET/CT in head and neck cancer. PloS One 2019; 14(3): e0214299, https://doi.org/10.1371/journal.pone.0214299.
- Yip S., McCall K., Aristophanous M., Chen A.B., Aerts H.J., Berbeco R. Comparison of texture features derived from static and respiratory-gated PET images in non-small cell lung cancer. PloS One 2014; 9(12): e115510, https://doi.org/10.1371/journal.pone.0115510.
- Wibmer A., Hricak H., Gondo T., Matsumoto K., Veeraraghavan H., Fehr D., Zheng J., Goldman D., Moskowitz C., Fine S.W., Reuter V.E., Eastham J., Sala E., Vargas H.A. Haralick texture analysis of prostate MRI: utility for differentiating non-cancerous prostate from prostate cancer and differentiating prostate cancers with different Gleason scores. Eur Radiol 2015; 25(10): 2840–2850, https://doi.org/10.1007/s00330-015-3701-8.
- Gnep K., Fargeas A., Gutiérrez-Carvajal R.E., Commandeur F., Mathieu R., Ospina J.D., Rolland Y., Rohou T., Vincendeau S., Hatt M., Acosta O., de Crevoisier R. Haralick textural features on T2-weighted MRI are associated with biochemical recurrence following radiotherapy for peripheral zone prostate cancer. J Magn Reson Imaging 2017; 45(1): 103–117, https://doi.org/10.1002/jmri.25335.
- Weygand J., Fuller C.D., Ibbott G.S., Mohamed A.S., Ding Y., Yang J., Hwang K.P., Wang J. Spatial precision in magnetic resonance imaging-guided radiation therapy: the role of geometric distortion. Int J Radiat Oncol Biol Phys 2016; 95(4): 1304–1316, https://doi.org/10.1016/j.ijrobp.2016.02.059.
- Sudarshan V.K., Mookiah M.R., Acharya U.R., Chandran V., Molinari F., Fujita H., Ng K.H. Application of wavelet techniques for cancer diagnosis using ultrasound images: a review. Comput Biol Med 2016; 69: 97–111, https://doi.org/10.1016/j.compbiomed.2015.12.006.
- Andrėkutė K., Linkevičiūtė G., Raišutis R., Valiukevičienė S., Makštienė J. Automatic differential diagnosis of melanocytic skin tumors using ultrasound data. Ultrasound Med Biol 2016; 42(12): 2834–2843, https://doi.org/10.1016/j.ultrasmedbio.2016.07.026.
- Nadeau M.J., Desrochers A., Lamontagne M., Larivière C., Gagnon D.H. Quantitative ultrasound imaging of Achilles tendon integrity in symptomatic and asymptomatic individuals: reliability and minimal detectable change. J Foot Ankle Res 2016; 9: 30, https://doi.org/10.1186/s13047-016-0164-3.
- Aerts H.J.W.L., Velazquez E.R., Leijenaar R.T., Parmar C., Grossmann P., Carvalho S., Bussink J., Monshouwer R., Haibe-Kains B., Rietveld D., Hoebers F., Rietbergen M.M., Leemans C.R., Dekker A., Quackenbush J., Gillies R.J., Lambin P. Decoding tumour phenotype by noninvasive imaging using a quantitative radiomics approach. Nat Commun 2014; 5: 4006, https://doi.org/10.1038/ncomms5006.
- Vallières M., Laberge S., Diamant A., El Naqa I. Enhancement of multimodality texture-based prediction models via optimization of PET and MR image acquisition protocols: a proof of concept. Phys Med Biol 2017; 62(22): 8536–8565, https://doi.org/10.1088/1361-6560/aa8a49.
- Bedard P.L., Hansen A.R., Ratain M.J., Siu L.L. Tumour heterogeneity in the clinic. Nature 2013; 501(7467): 355–364, https://doi.org/10.1038/nature12627.
- Ganeshan B., Miles K.A. Quantifying tumour heterogeneity with CT. Cancer Imaging 2013; 13(1): 140–149, https://doi.org/10.1102/1470-7330.2013.0015.
- Liu Z., Wang Y., Liu X., Du Y., Tang Z., Wang K., Wei J., Dong D., Zang Y., Dai J., Jiang T., Tian J. Radiomics analysis allows for precise prediction of epilepsy in patients with low-grade gliomas. Neuroimage Clin 2018; 19: 271–278, https://doi.org/10.1016/j.nicl.2018.04.024.
- Guo J., Liu Z., Shen C., Li Z., Yan F., Tian J., Xian J. MR-based radiomics signature in differentiating ocular adnexal lymphoma from idiopathic orbital inflammation. Eur Radiol 2018; 28(9): 3872–3881, https://doi.org/10.1007/s00330-018-5381-7.
- Tang Z., Liu Z., Li R., Yang X., Cui X., Wang S., Yu D., Li H., Dong E., Tian J. Identifying the white matter impairments among ART-naïve HIV patients: a multivariate pattern analysis of DTI data. Eur Radiol 2017; 27(10): 4153–4162, https://doi.org/10.1007/s00330-017-4820-1.
- Shen C., Liu Z., Guan M., Song J., Lian Y., Wang S., Tang Z., Dong D., Kong L., Wang M., Shi D., Tian J. 2D and 3D CT radiomics features prognostic performance comparison in non-small cell lung cancer. Transl Oncol 2017; 10(6): 886–894, https://doi.org/10.1016/j.tranon.2017.08.007.
- Shen C., Liu Z., Wang Z., Guo J., Zhang H., Wang Y., Qin J., Li H., Fang M., Tang Z., Li Y., Qu J., Tian J. Building CT radiomics based nomogram for preoperative esophageal cancer patients lymph node metastasis prediction. Transl Oncol 2018; 11(3): 815–824, https://doi.org/10.1016/j.tranon.2018.04.005.
- Nioche C., Orlhac F., Boughdad S., Reuzé S., Goya-Outi J., Robert C., Pellot-Barakat C., Soussan M., Frouin F., Buvat I. LIFEx: a freeware for radiomic feature calculation in multimodality imaging to accelerate advances in the characterization of tumor heterogeneity. Cancer Res 2018; 78(16): 4786–4789, https://doi.org/10.1158/0008-5472.can-18-0125.
- Zhang L., Fried D.V., Fave X.J., Hunter L.A., Yang J., Court L.E. IBEX: an open infrastructure software platform to facilitate collaborative work in radiomics. Med Phys 2015; 42(3): 1341–1353, https://doi.org/10.1118/1.4908210.
- Buvat I., Orlhac F., Soussan M. Tumor texture analysis in PET: where do we stand? J Nucl Med 2015; 56(11): 1642–1644, https://doi.org/10.2967/jnumed.115.163469.
- van Griethuysen J.J.M., Fedorov A., Parmar C., Hosny A., Aucoin N., Narayan V., Beets-Tan R.G.H., Fillion-Robin J.C., Pieper S., Aerts H.J.W.L. Computational radiomics system to decode the radiographic phenotype. Cancer Res 2017; 77(21): e104–e107, https://doi.org/10.1158/0008-5472.can-17-0339.
- Ger R.B., Cardenas C.E., Anderson B.M., Yang J., Mackin D.S., Zhang L., Court L.E. Guidelines and experience using imaging biomarker explorer (IBEX) for radiomics. J Vis Exp 2018; 131: 57132, https://doi.org/10.3791/57132.
- Bettinelli A., Branchini M., De Monte F., Scaggion A., Paiusco M. Technical note: an IBEX adaption toward image biomarker standardization. Med Phys 2020; 47(3): 1167–1173, https://doi.org/10.1002/mp.13956.
- Zwanenburg A., Vallières M., Abdalah M.A., Aerts H.J.W.L., Andrearczyk V., Apte A., Ashrafinia S., Bakas S., Beukinga R.J., Boellaard R., Bogowicz M., Boldrini L., Buvat I., Cook G.J.R., Davatzikos C., Depeursinge A., Desseroit M.C., Dinapoli N., Dinh C.V., Echegaray S., El Naqa I., Fedorov A.Y., Gatta R., Gillies R.J., Goh V., Götz M., Guckenberger M., Ha S.M., Hatt M., Isensee F., Lambin P., Leger S., Leijenaar R.T.H., Lenkowicz J., Lippert F., Losnegård A., Maier-Hein K.H., Morin O., Müller H., Napel S., Nioche C., Orlhac F., Pati S., Pfaehler E.A.G., Rahmim A., Rao A.U.K., Scherer J., Siddique M.M., Sijtsema N.M., Socarras Fernandez J., Spezi E., Steenbakkers R.J.H.M., Tanadini-Lang S., Thorwarth D., Troost E.G.C., Upadhaya T., Valentini V., van Dijk L.V., van Griethuysen J., van Velden F.H.P., Whybra P., Richter C., Löck S. The image biomarker standardization initiative: standardized quantitative radiomics for high-throughput image-based phenotyping. Radiology 2020; 295(2): 328–338, https://doi.org/10.1148/radiol.2020191145.
- Gillies R.J., Kinahan P.E., Hricak H. Radiomics: images are more than pictures, they are data. Radiology 2016; 278(2): 563–577, https://doi.org/10.1148/radiol.2015151169.
- van Velden F.H., Kramer G.M., Frings V., Nissen I.A., Mulder E.R., de Langen A.J., Hoekstra O.S., Smit E.F., Boellaard R. Repeatability of radiomic features in non-small-cell lung cancer [18F]FDG-PET/CT studies: impact of reconstruction and delineation. Mol Imaging Biol 2016; 18(5): 788–795, https://doi.org/10.1007/s11307-016-0940-2.
- Balagurunathan Y., Gu Y., Wang H., Kumar V., Grove O., Hawkins S., Kim J., Goldgof D.B., Hall L.O., Gatenby R.A., Gillies R.J. Reproducibility and prognosis of quantitative features extracted from CT images. Transl Oncol 2014; 7(1): 72–87, https://doi.org/10.1593/tlo.13844.
- Armato S.G. 3rd, McLennan G., Bidaut L., McNitt-Gray M.F., Meyer C.R., Reeves A.P., Zhao B., Aberle D.R., Henschke C.I., Hoffman E.A., Kazerooni E.A., MacMahon H., Van Beeke E.J., Yankelevitz D., Biancardi A.M., Bland P.H., Brown M.S., Engelmann R.M., Laderach G.E., Max D., Pais R.C., Qing D.P., Roberts R.Y., Smith A.R., Starkey A., Batrah P., Caligiuri P., Farooqi A., Gladish G.W., Jude C.M., Munden R.F., Petkovska I., Quint L.E., Schwartz L.H., Sundaram B., Dodd L.E., Fenimore C., Gur D., Petrick N., Freymann J., Kirby J., Hughes B., Casteele A.V., Gupte S., Sallamm M., Heath M.D., Kuhn M.H., Dharaiya E., Burns R., Fryd D.S., Salganicoff M., Anand V., Shreter U., Vastagh S., Croft B.Y. The Lung Image Database Consortium (LIDC) and Image Database Resource Initiative (IDRI): a completed reference database of lung nodules on CT scans. Med Phys 2011; 38(2): 915–931, https://doi.org/10.1118/1.3528204.
- Huang Y.Q., Liang C.H., He L., Tian J., Liang C.S., Chen X., Ma Z.L., Liu Z.Y. Development and validation of a radiomics nomogram for preoperative prediction of lymph node metastasis in colorectal cancer. J Clin Oncol 2016; 34(18): 2157–2164, https://doi.org/10.1200/jco.2015.65.9128.
- Liu Z., Zhang X.Y., Shi Y.J., Wang L., Zhu H.T., Tang Z., Wang S., Li X.T., Tian J., Sun Y.S. Radiomics analysis for evaluation of pathological complete response to neoadjuvant chemoradiotherapy in locally advanced rectal cancer. Clin Cancer Res 2017; 23(23): 7253–7262, https://doi.org/10.1158/1078-0432.ccr-17-1038.
- Rios Velazquez E., Parmar C., Liu Y., Coroller T.P., Cruz G., Stringfield O., Ye Z., Makrigiorgos M., Fennessy F., Mak R.H., Gillies R., Quackenbush J., Aerts H.J.W.L. Somatic mutations drive distinct imaging phenotypes in lung cancer. Cancer Res 2017; 77(14): 3922–3930, https://doi.org/10.1158/0008-5472.can-17-0122.
- Kramer A.A., Zimmerman J.E. Assessing the calibration of mortality benchmarks in critical care: the Hosmer–Lemeshow test revisited. Crit Care Med 2007; 35(9): 2052–2056, https://doi.org/10.1097/01.ccm.0000275267.64078.b0.
- Rizzo S., Botta F., Raimondi S., Origgi D., Fanciullo C., Morganti A.G., Bellomi M. Radiomics: the facts and the challenges of image analysis. Eur Radiol Exp 2018; 2(1): 36, https://doi.org/10.1186/s41747-018-0068-z.
- Arimura H., Soufi M., Ninomiya K., Kamezawa H., Yamada M. Potentials of radiomics for cancer diagnosis and treatment in comparison with computer-aided diagnosis. Radiol Phys Technol 2018; 11(4): 365–374, https://doi.org/10.1007/s12194-018-0486-x.
- Chicklore S., Goh V., Siddique M., Roy A., Marsden P.K., Cook G.J. Quantifying tumour heterogeneity in 18F-FDG PET/CT imaging by texture analysis. Eur J Nucl Med Mol Imaging 2013; 40(1): 133–140, https://doi.org/10.1007/s00259-012-2247-0.
- Parekh V., Jacobs M.A. Radiomics: a new application from established techniques. Expert Rev Precis Med Drug Dev 2016; 1(2): 207–226, https://doi.org/10.1080/23808993.2016.1164013.
- Scalco E., Rizzo G. Texture analysis of medical images for radiotherapy applications. Br J Radiol 2017; 90(1070): 20160642, https://doi.org/10.1259/bjr.20160642.
- Kwak J.T., Xu S., Wood B.J. Efficient data mining for local binary pattern in texture image analysis. Expert Syst Appl 2015; 42(9): 4529–4539, https://doi.org/10.1016/j.eswa.2015.01.055.
- McCann M.T., Mixon D.G., Fickus M.C., Castro C.A., Ozolek J.A., Kovacevic J. Images as occlusions of textures: a framework for segmentation. IEEE Trans Image Process 2014; 23(5): 2033–2046, https://doi.org/10.1109/tip.2014.2307475.
- Pyka T., Bundschuh R.A., Andratschke N., Mayer B., Specht H.M., Papp L., Zsótér N., Essler M. Textural features in pre-treatment [F18]-FDG-PET/CT are correlated with risk of local recurrence and disease-specific survival in early stage NSCLC patients receiving primary stereotactic radiation therapy. Radiat Oncol 2015; 10: 100, https://doi.org/10.1186/s13014-015-0407-7.
- Arimura H., Soufi M., Kamezawa H., Ninomiya K., Yamada M. Radiomics with artificial intelligence for precision medicine in radiation therapy. J Radiat Res 2019; 60(1): 150–157, https://doi.org/10.1093/jrr/rry077.
- Mayerhoefer M.E., Materka A., Langs G., Häggström I., Szczypiński P., Gibbs P., Cook G. Introduction to radiomics. J Nucl Med 2020; 61(4): 488–495, https://doi.org/10.2967/jnumed.118.222893.
- Wu J., Tha K.K., Xing L., Li R. Radiomics and radiogenomics for precision radiotherapy. J Radiat Res 2018; 59(suppl 1): i25–i31, https://doi.org/10.1093/jrr/rrx102.
- Wang J., Shen L., Zhong H., Zhou Z., Hu P., Gan J., Luo R., Hu W., Zhang Z. Radiomics features on radiotherapy treatment planning CT can predict patient survival in locally advanced rectal cancer patients. Sci Rep 2019; 9(1): 15346, https://doi.org/10.1038/s41598-019-51629-4.
- Bae S., Choi Y.S., Ahn S.S., Chang J.H., Kang S.G., Kim E.H., Kim S.H., Lee S.K. Radiomic MRI phenotyping of glioblastoma: improving survival prediction. Radiology 2018; 289(3): 797–806, https://doi.org/10.1148/radiol.2018180200.
- Oikonomou A., Khalvati F., Tyrrell P.N., Haider M.A., Tarique U., Jimenez-Juan L., Tjong M.C., Poon I., Eilaghi A., Ehrlich L., Cheung P. Radiomics analysis at PET/CT contributes to prognosis of recurrence and survival in lung cancer treated with stereotactic body radiotherapy. Sci Rep 2018; 8(1): 4003, https://doi.org/10.1038/s41598-018-22357-y.
- Kirienko M., Cozzi L., Antunovic L., Lozza L., Fogliata A., Voulaz E., Rossi A., Chiti A., Sollini M. Prediction of disease-free survival by the PET/CT radiomic signature in non-small cell lung cancer patients undergoing surgery. Eur J Nucl Med Mol Imaging 2018; 45(2): 207–217, https://doi.org/10.1007/s00259-017-3837-7.
- Wu W., Parmar C., Grossmann P., Quackenbush J., Lambin P., Bussink J., Mak R., Aerts H.J. Exploratory study to identify radiomics classifiers for lung cancer histology. Front Oncol 2016; 6: 71, https://doi.org/10.3389/fonc.2016.00071.
- Filatau A., Litvin A., Litvin V., Kovalev V. Anisotropy of CT images in the differential diagnosis of chronic pancreatitis and pancreatic cancer. Pancreatology 2014; 14(3 Suppl 1): S89–S90, https://doi.org/10.1016/j.pan.2014.05.682.
- Wu M., Tan H., Gao F., Hai J., Ning P., Chen J., Zhu S., Wang M., Dou S., Shi D. Predicting the grade of hepatocellular carcinoma based on non-contrast-enhanced MRI radiomics signature. Eur Radiol 2019; 29(6): 2802–2811, https://doi.org/10.1007/s00330-018-5787-2.
- Vallières M., Freeman C.R., Skamene S.R., El Naqa I. A radiomics model from joint FDG-PET and MRI texture features for the prediction of lung metastases in soft-tissue sarcomas of the extremities. Phys Med Biol 2015; 60(14): 5471–5496, https://doi.org/10.1088/0031-9155/60/14/5471.
- Trebeschi S., Drago S.G., Birkbak N.J., Kurilova I., Cǎlin A.M., Delli Pizzi A., Lalezari F., Lambregts D.M.J., Rohaan M.W., Parmar C., Rozeman E.A., Hartemink K.J., Swanton C., Haanen J.B.A.G., Blank C.U., Smit E.F., Beets-Tan R.G.H., Aerts H.J.W.L. Predicting response to cancer immunotherapy using noninvasive radiomic biomarkers. Ann Oncol 2019; 30(6): 998–1004, https://doi.org/10.1093/annonc/mdz108.
- Horvat N., Veeraraghavan H., Khan M., Blazic I., Zheng J., Capanu M., Sala E., Garcia-Aguilar J., Gollub M.J., Petkovska I. MR imaging of rectal cancer: radiomics analysis to assess treatment response after neoadjuvant therapy. Radiology 2018; 287(3): 833–843, https://doi.org/10.1148/radiol.2018172300.
- Chang Y.N., Chang H.H. Automatic brain MR image denoising based on texture feature-based artificial neural networks. Biomed Mater Eng 2015; 26(Suppl 1): S1275–S1282, https://doi.org/10.3233/bme-151425.
- Jiang J., Hu Y.C., Liu C.J., Halpenny D., Hellmann M.D., Deasy J.O., Mageras G., Veeraraghavan H. Multiple resolution residually connected feature streams for automatic lung tumor segmentation from CT images. EEE Trans Med Imaging 2019; 38(1): 134–144, https://doi.org/10.1109/tmi.2018.2857800.
- Yi D., Zhou M., Chen Z., Gevaert O. 3-D convolutional neural networks for glioblastoma segmentation. arXiv 2016; 1611.04534; URL: http://arxiv.org/abs/1611.04534.
- Ghaffari M., Sowmya A., Oliver R. Automated brain tumor segmentation using multimodal brain scans: a survey based on models submitted to the BraTS 2012–2018 challenges. IEEE Rev Biomed Eng 2020; 13: 156–168, https://doi.org/10.1109/rbme.2019.2946868.
- Chen L., Shen C., Zhou Z., Maquilan G., Albuquerque K., Folkert M.R., Wang J. Automatic PET cervical tumor segmentation by combining deep learning and anatomic prior. Phys Med Biol 2019; 64(8): 085019, https://doi.org/10.1088/1361-6560/ab0b64.
- Ardila D., Kiraly A.P., Bharadwaj S., Choi B., Reicher J.J., Peng L., Tse D., Etemadi M., Ye W., Corrado G., Naidich D.P., Shetty S. End-to-end lung cancer screening with three-dimensional deep learning on low-dose chest computed tomography. Nat Med 2019; 25(6): 954–961, https://doi.org/10.1038/s41591-019-0447-x.
- Abdelaziz Ismael S.A., Mohammed A., Hefny H. An enhanced deep learning approach for brain cancer MRI images classification using residual networks. Artif Intell Med 2020; 102: 101779, https://doi.org/10.1016/j.artmed.2019.101779.
- Sibille L., Seifert R., Avramovic N., Vehren T., Spottiswoode B., Zuehlsdorff S., Schäfers M. 18F-FDG PET/CT uptake classification in lymphoma and lung cancer by using deep convolutional neural networks. Radiology 2020; 294(2): 445–452, https://doi.org/10.1148/radiol.2019191114.
- Lou B., Doken S., Zhuang T., Wingerter D., Gidwani M., Mistry N., Ladic L., Kamen A., Abazeed M.E. An image-based deep learning framework for individualizing radiotherapy dose. Lancet Digit Health 2019; 1(3): e136–e147, https://doi.org/10.1016/s2589-7500(19)30058-5.
- Ypsilantis P.P., Siddique M., Sohn H.M., Davies A., Cook G., Goh V., Montana G. Predicting response to neoadjuvant chemotherapy with PET imaging using convolutional neural networks. PloS One 2015; 10(9): e0137036, https://doi.org/10.1371/journal.pone.0137036.
- Coroller T.P., Grossmann P., Hou Y., Rios Velazquez E., Leijenaar R.T., Hermann G., Lambin P., Haibe-Kains B., Mak R.H., Aerts H.J.W.L. CT-based radiomic signature predicts distant metastasis in lung adenocarcinoma. Radiother Oncol 2015; 114(3): 345–350, https://doi.org/10.1016/j.radonc.2015.02.015.
- Coroller T.P., Agrawal V., Narayan V., Hou Y., Grossmann P., Lee S.W., Mak R.H., Aerts H.J.W.L. Radiomic phenotype features predict pathological response in non-small cell lung cancer. Radiother Oncol 2016; 119(3): 480–486, https://doi.org/10.1016/j.radonc.2016.04.004.
- Mattonen S.A., Palma D.A., Johnson C., Louie A.V., Landis M., Rodrigues G., Chan I., Etemad-Rezai R., Yeung T.P., Senan S., Ward A.D. Detection of local cancer recurrence after stereotactic ablative radiation therapy for lung cancer: physician performance versus radiomic assessment. Int J Radiat Oncol Biol Phys 2016; 94(5): 1121–1128, https://doi.org/10.1016/j.ijrobp.2015.12.369.
- Cook G.J., Yip C., Siddique M., Goh V., Chicklore S., Roy A., Marsden P., Ahmad S., Landau D. Are pretreatment 18F-FDG PET tumor textural features in non-small cell lung cancer associated with response and survival after chemoradiotherapy? J Nucl Med 2013; 54(1): 19–26, https://doi.org/10.2967/jnumed.112.107375.
- Huang Y., Liu Z., He L., Chen X., Pan D., Ma Z., Liang C., Tian J., Liang C. Radiomics signature: a potential biomarker for the prediction of disease-free survival in early-stage (I or II) non-small cell lung cancer. Radiology 2016; 281(3): 947–957, https://doi.org/10.1148/radiol.2016152234.
- Summers R.M. Texture analysis in radiology: does the emperor have no clothes? Abdom Radiol (NY) 2017; 42(2): 342–345, https://doi.org/10.1007/s00261-016-0950-1.
- Chalkidou A., O’Doherty M.J., Marsden P.K. False discovery rates in PET and CT studies with texture features: a systematic review. PloS One 2015; 10(5): e0124165, https://doi.org/10.1371/journal.pone.0124165.
- Alic L., Niessen W.J., Veenland J.F. Quantification of heterogeneity as a biomarker in tumor imaging: a systematic review. PloS One 2014; 9(10): e110300, https://doi.org/10.1371/journal.pone.0110300.
- Han S., Woo S., Suh C.H., Kim Y.J., Oh J.S., Lee J.J. A systematic review of the prognostic value of texture analysis in 18F-FDG PET in lung cancer. Ann Nucl Med 2018; 32(9): 602–610, https://doi.org/10.1007/s12149-018-1281-9.
- Leijenaar R.T., Carvalho S., Hoebers F.J., Aerts H.J., van Elmpt W.J., Huang S.H., Chan B., Waldron J.N., O’sullivan B., Lambin P. External validation of a prognostic CT-based radiomic signature in oropharyngeal squamous cell carcinoma. Acta Oncol 2015; 54(9): 1423–1429, https://doi.org/10.3109/0284186x.2015.1061214.
- Fave X., Cook M., Frederick A., Zhang L., Yang J., Fried D., Stingo F., Court L. Preliminary investigation into sources of uncertainty in quantitative imaging features. Comput Med Imaging Graph 2015; 44: 54–61, https://doi.org/10.1016/j.compmedimag.2015.04.006.
- Zanfardino M., Franzese M., Pane K., Cavaliere C., Monti S., Esposito G., Salvatore M., Aiello M. Bringing radiomics into a multi-omics framework for a comprehensive genotype-phenotype characterization of oncological diseases. J Transl Med 2019; 17(1): 337, https://doi.org/10.1186/s12967-019-2073-2.