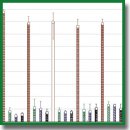
Assessment of Clinical Decision Support System Efficiency in Spinal Neurosurgery for Personalized Minimally Invasive Technologies Used on Lumbar Spine
The aim of the study was to assess clinical decision support system (CDSS) in spinal surgery for personalized minimally invasive technologies on lumbar spine.
Materials and Methods. The prospective study involved 59 patients operated on using CDSS based on a personalized surgical algorithm considering patient-specific parameters of lumbar segments. Among them, 11 patients underwent total disk replacement (TDR), 25 and 23 patients had minimally invasive (MI-TLIF) and open (O-TLIF) dorsal rigid stabilization, respectively, according to an original technology. The comparative analysis was carried out using retrospective findings of 196 patients operated on involving TDR (n=42), MI-TLIF (n=79), and O-TLIF (n=75). The efficiency of CDSS medical algorithms was assessed by pain syndrome in the lumbar spine and lower limbs, as well as by patients’ functional status on discharge according to ODI, 3 and 6 months after the operation.
Results. The comparison by gender characteristics and anthropometric data revealed no significant intergroup differences among the groups under study (p>0.05). Intergroup analysis of functional status by ODI, pain intensity in lower limbs and lumbar spine showed better clinical outcomes in patients operated using CDSS compared to a retrospective group (p<0.05): 6 months after TDR and O-TLIF, and 3 months after MI-TLIF.
Conclusion. The study findings demonstrated high efficiency of CDSS developed for personalized surgical treatment of patients with degenerative lumbar spine diseases taking into consideration individual biometric parameters of lumbar segments.
- Bagley C., MacAllister M., Dosselman L., Moreno J., Aoun S.G., El Ahmadieh T.Y. Current concepts and recent advances in understanding and managing lumbar spine stenosis. F1000Res 2019; 8: 137, https://doi.org/10.12688/f1000research.16082.1.
- Krivoshein A.E., Konev V.P., Kolesov S.V., Moskovsky S.N., Ignatiev Yu.T., Nikitenko S.A., Erofeev S.A. Morphological and radiological parameters of the facet joints depending on the degree of intervertebral disc degeneration. Genij ortopedii 2020; 26(4): 565–570.
- Byvaltsev V.A., Kalinin A.A., Konovalov N.A. Minimally invasive spinal surgery: stages of development. Voprosy nejrohirurgii imeni N.N. Burdenko 2019; 83(5): 92–100, https://doi.org/10.17116/neiro20198305192.
- Eliseev A.S., Bokov A.E., Mlyavykh S.G., Mordvinov A.A. Philosophy of some problems of spinal neurosurgery. Voprosy nejrohirurgii imeni N.N. Burdenko 2021; 85(1): 28–35, https://doi.org/10.17116/neiro20218501128.
- Afaunov A.A., Basankin I.V., Kuzmenko A.V., Shapovalov V.K. Analysis of the causes of revision operations in the surgical treatment of patients with degenerative lumbar stenosis etiology. Kubanskij nauchnyj medicinskij vestnik 2013; 7: 173–176.
- Mohi Eldin M.M., Hassan A.S. Percutaneous transpedicular fixation: technical tips and pitfalls of sextant and pathfinder systems. Asian Spine J 2016; 10(1): 111–122, https://doi.org/10.4184/asj.2016.10.1.111.
- Baranowska A., Baranowska J., Baranowski P. Analysis of reasons for failure of surgery for degenerative disease of lumbar spine. Ortop Traumatol Rehabil 2016; 18(2): 117–129, https://doi.org/10.5604/15093492.1205004.
- Baskov A.V., Evsyukov A.A., Ogleznev K.Ya., Sidorov E.V. Predicting the results of surgical treatment of acquired spinal stenosis at the level of the lumbar spine. Voprosy nejrohirurgii imeni N.N. Burdenko 2003; 2: 20–26.
- Belykh E., Kalinin A.A., Patel A.A., Miller E.J., Bohl M.A., Stepanov I.A., Bardonova L.A., Kerimbaev T., Asantsev A.O., Giers M.B., Preul M.C., Byvaltsev V.A. Apparent diffusion coefficient maps in the assessment of surgical patients with lumbar spine degeneration. PLoS One 2017; 12(8): e0183697, https://doi.org/10.1371/journal.pone.0183697.
- Ancker J.S., Edwards A., Nosal S., Hauser D., Mauer E., Kaushal R.; with the HITEC Investigators. Effects of workload, work complexity, and repeated alerts on alert fatigue in a clinical decision support system. BMC Med Inform Decis Mak 2017; 17(1): 36, https://doi.org/10.1186/s12911-017-0430-8.
- Danilov G.V., Shifrin M.A., Kotik K.V., Ishankulov T.A., Orlov Yu.N., Kulikov A.S., Potapov A.A. Artificial intelligence technologies in neurosurgery: a systematic literature review using topical modeling methods. Part I: principal areas of research (review). Sovremennye tehnologii v medicine 2020; 12(5): 106–113, https://doi.org/10.17691/stm2020.12.5.12.
- Reddy S., Fox J., Purohit M.P. Artificial intelligence-enabled healthcare delivery. J R Soc Med 2019; 112(1): 22–28, https://doi.org/10.1177/0141076818815510.
- Chang M., Canseco J.A., Nicholson K.J., Patel N., Vaccaro A.R. The role of machine learning in spine surgery: the future is now. Front Surg 2020; 7: 54, https://doi.org/10.3389/fsurg.2020.00054.
- Obermeyer Z., Emanuel E.J. Predicting the future — big data, machine learning, and clinical medicine. N Engl J Med 2016; 375(13): 1216–1219, https://doi.org/10.1056/nejmp1606181.
- Byval'tsev V.A., Kalinin A.A., Stepanov I.A. Ispol'zovanie lechebnogo algoritma vypolneniya minimal'no-invazivnykh operativnykh vmeshatel'stv pri degenerativnykh zabolevaniyakh poyasnichnogo otdela pozvonochnika na osnove kompleksnoy predoperatsionnoy klinicheskoy i instrumental'noy otsenki. Metodicheskie rekomendatsii [The use of a therapeutic algorithm for performing minimally invasive surgical interventions for degenerative diseases of the lumbar spine based on a comprehensive preoperative clinical and instrumental assessment. Guidelines]. Irkutsk: IGMU; 2019.
- Byvaltsev V.A., Kalinin A.A. Method of minimally invasive surgical management of stenosis of spinal canal of lumbar spine. Patent RU 2731809. 2018.
- Byvaltsev V.A., Sorokovikov V.A., Kalinin A.A., Belykh E.G. Method for spinal canal repair accompanying lumbosacral stenosis. Patent RU 2531927. 2014.
- Triantafyllidis A.K., Tsanas A. Applications of machine learning in real-life digital health interventions: review of the literature. J Med Internet Res 2019; 21(4): e12286, https://doi.org/10.2196/12286.
- Deo R.C. Machine learning in medicine. Circulation 2015; 132(20): 1920–1930, https://doi.org/10.1161/circulationaha.115.001593.
- Staartjes V.E., de Wispelaere M.P., Vandertop W.P., Schröder M.L. Deep learning-based preoperative predictive analytics for patient-reported outcomes following lumbar discectomy: feasibility of center-specific modeling. Spine J 2019; 19(5): 853–861, https://doi.org/10.1016/j.spinee.2018.11.009.
- Wirries A., Geiger F., Hammad A., Oberkircher L., Blümcke I., Jabari S. Artificial intelligence facilitates decision-making in the treatment of lumbar disc herniations. Eur Spine J 2021; 30(8): 2176–2184, https://doi.org/10.1007/s00586-020-06613-2.
- Siccoli A., de Wispelaere M.P., Schröder M.L., Staartjes V.E. Machine learning-based preoperative predictive analytics for lumbar spinal stenosis. Neurosurg Focus 2019; 46(5): E5, https://doi.org/10.3171/2019.2.focus18723.
- Lee N.J., Sardar Z.M., Boddapati V., Mathew J., Cerpa M., Leung E., Lombardi J., Lenke L.G., Lehman R.A. Can machine learning accurately predict postoperative compensation for the uninstrumented thoracic spine and pelvis after fusion from the lower thoracic spine to the sacrum? Global Spine J 2020; 8: 2192568220956978, https://doi.org/10.1177/2192568220956978.
- Campagner A., Berjano P., Lamartina C., Langella F., Lombardi G., Cabitza F. Assessment and prediction of spine surgery invasiveness with machine learning techniques. Comput Biol Med 2020; 121: 103796, https://doi.org/10.1016/j.compbiomed.2020.103796.
- Raman T., Vasquez-Montes D., Varlotta C., Passias P.G., Errico T.J. Decision tree-based modelling for identification of predictors of blood loss and transfusion requirement after adult spinal deformity surgery. Int J Spine Surg 2020; 14(1): 87–95, https://doi.org/10.14444/7012.
- Lafage R., Pesenti S., Lafage V., Schwab F.J. Self-learning computers for surgical planning and prediction of postoperative alignment. Eur Spine J 2018; 27(Suppl 1): 123–128, https://doi.org/10.1007/s00586-018-5497-0.
- Char D.S., Shah N.H., Magnus D. Implementing machine learning in health care — addressing ethical challenges. N Engl J Med 2018; 378(11): 981–983, https://doi.org/10.1056/nejmp1714229.
- Shaw J., Rudzicz F., Jamieson T., Goldfarb A. Artificial intelligence and the implementation challenge. J Med Internet Res 2019; 21(7): e13659, https://doi.org/10.2196/13659.