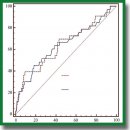
Using an Artificial Neural Network to Predict Coronary Microvascular Obstruction (No-Reflow Phenomenon) during Percutaneous Coronary Interventions in Patients with Myocardial Infarction
The aim of the study was to develop, evaluate, and validate an artificial neural network to predict coronary microvascular obstruction (CMVO) during percutaneous coronary interventions (PCI) in patients with myocardial infarctions (MI) based on the parameters, which are routinely available in an operating room when choosing a surgical approach.
Materials and Methods. 5621 patients with MI and emergency PCI were retrospectively selected from the database of the City Clinical Hospital No.13 (Nizhny Novgorod, Russia); among them, there were 3935 men (70%) and 1686 women (30%), their mean age was 61.5±10.8 years. CMVO was recorded in 201 (4%) patients (the blood flow in the infarction-related artery after PCI was less than 3 points according to TIMI flow grade). The following input parameters were assessed: age, gender, past history of coronary artery disease, previous revascularization, presence of ST-segment elevation, a class of acute heart failure, a fact of systemic thrombolytic therapy administration and its effectiveness, symptom-to-balloon time, severity of coronary thrombosis and atherosclerosis, the number of stents and the number of operated coronary arteries. The sampling was divided into a training group (n=4060), a testing group (n=717), and an independent validation group (n=844).
Results. We developed an artificial neural network by a fully connected multilayer perception with forward signal propagation and two hidden layers (the area under the ROC curve — 0.69) to predict CMVO based on the subsampling for training and testing. The network model was tested on an independent subsampling (the area under the ROC curve — 0.64, negative predictive value — 97.4%, positive predictive value — 14.6%).
Conclusion. The developed artificial neural network enables to use the parameters routinely available in an operating room when choosing a surgical approach and predict CMVO development during PCI in MI patients with accuracy sufficient for practical use.
- Gupta S., Gupta M.M. No reflow phenomenon in percutaneous coronary interventions in ST-segment elevation myocardial infarction. Indian Heart J 2016; 68(4): 539–551, https://doi.org/10.1016/j.ihj.2016.04.006.
- Niccoli G., Scalone G., Lerman A., Crea F. Coronary microvascular obstruction in acute myocardial infarction. Eur Heart J 2016; 37(13): 1024–1033, https://doi.org/10.1093/eurheartj/ehv484.
- Wang J.W., Zhou Z.Q., Chen Y.D., Wang C.H., Zhu X.L. A risk score for no reflow in patients with ST-segment elevation myocardial infarction after percutaneous coronary intervention. Clin Cardiol 2015; 38(4): 208–215, https://doi.org/10.1002/clc.22376.
- Thygesen K., Alpert J.S., Jaffe A.S., Chaitman B.R., Bax J.J., Morrow D.A., White H.D.; ESC Scientific Document Group. Fourth universal definition of myocardial infarction (2018). Eur Heart J 2018; 40(3): 237–269, https://doi.org/10.1093/eurheartj/ehy462.
- The TIMI Study Group. The thrombolysis in myocardial infarction (TIMI) trial. Phase I findings. N Engl J Med 1985; 312(14): 932–936, https://doi.org/10.1056/nejm198504043121437.
- Gibson C.M., de Lemos J.A., Murphy S.A., Marble S.J., McCabe C.H., Cannon C.P., Antman E.M., Braunwald E. Combination therapy with abciximab reduces angiographically evident thrombus in acute myocardial infarction: a TIMI 14 substudy. Circulation 2001; 103(21): 2550–2554, https://doi.org/10.1161/01.cir.103.21.2550.
- Alasadi S.A., Bhaya W.S. Review of data preprocessing techniques in data mining. J Eng Appl Sci 2017; 12(16): 4102–4107.
- Kaur H., Pannu H.S., Malhi A.K. A systematic review on imbalanced data challenges in machine learning: applications and solutions. ACM Comput Surv 2019; 52(4): 1–36, https://doi.org/10.1145/3343440.
- Pang B., Nijkamp E., Wu Y.N. Deep learning with TensorFlow: a review. J Educ Behav Stat 2020; 45(2): 227–248, https://doi.org/10.3102/1076998619872761.
- Susan S., Kumar A. The balancing trick: optimized sampling of imbalanced datasets — a brief survey of the recent state of the art. Eng Reports 2020; 3(4): e12298, https://doi.org/10.1002/eng2.12298.
- Ramchoun H., Amine M., Idrissi J., Ghanou Y., Ettaouil M. Multilayer perceptron: architecture optimization and training. Int J Interact Multimed Artif Intell 2016; 4(1): 26–30, https://doi.org/10.9781/ijimai.2016.415.
- Liu X., Pan Z., Yang H., Zhou X., Bai W., Niu X. An adaptive moment estimation method for online AUC maximization. PLoS One 2019; 14(4): e0215426, https://doi.org/10.1371/journal.pone.0215426.
- Ramos D., Franco-Pedroso J., Lozano-Diez A., Gonzalez-Rodriguez J. Deconstructing cross-entropy for probabilistic binary classifiers. Entropy (Basel) 2018; 20(3): 208, https://doi.org/10.3390/e20030208.
- Burnaev E.V., Erofeev P.D. The influence of parameter initialization on the training time and accuracy of a nonlinear regression model. J Commun Technol Electron 2016; 61(6): 646–660, https://doi.org/10.1134/s106422691606005x.
- Ying X. An overview of overfitting and its solutions. J Phys: Conf Ser 2019; 1168: 022022, https://doi.org/10.1088/1742-6596/1168/2/022022.
- Ranganathan P., Pramesh C.S., Aggarwal R. Common pitfalls in statistical analysis: measures of agreement. Perspect Clin Res 2017; 8(4): 187–191, https://doi.org/10.4103/picr.picr_123_17.
- Niccoli G., Montone R.A., Ibanez B., Thiele H., Crea F., Heusch G., Bulluck H., Hausenloy D.J., Berry C., Stiermaier T., Camici P.G., Eitel I. Optimized treatment of ST-elevation myocardial infarction the unmet need to target coronary microvascular obstruction as primary treatment goal to further improve prognosis. Circ Res 2019; 125(2): 245–258, https://doi.org/10.1161/circresaha.119.315344.
- Karamasis G.V., Kalogeropoulos A.S., Gamma R.A., Clesham G.J., Marco V., Tang K.H., Jagathesan R., Sayer J.W., Robinson N.M., Kabir A., Aggarwal R.K., Kelly P.A., Prati F., Keeble T.R., Davies J.R. Effects of stent postdilatation during primary PCI for STEMI: insights from coronary physiology and optical coherence tomography. Catheter Cardiovasc Interv 2021; 97(7): 1309–1317, https://doi.org/10.1002/ccd.28932.
- Karathanos A., Lin Y., Dannenberg L., Parco C., Schulze V., Brockmeyer M., Jung C., Heinen Y., Perings S., Zeymer U., Kelm M., Polzin A., Wolff G. Routine glycoprotein IIb/IIIa inhibitor therapy in ST-segment elevation myocardial infarction: a meta-analysis. Can J Cardiol 2019; 35(11): 1576–1588, https://doi.org/10.1016/j.cjca.2019.05.003.