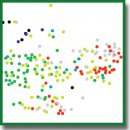
Age Classification in Forensic Medicine Using Machine Learning Techniques
The aim of the study was to assess the capabilities of age determination (age group) at death using classification techniques by histomorphometric characteristics of osseous and cartilaginous tissue aging.
Materials and Methods. The study material was a database containing the findings of morphometric researches of osseous and cartilaginous tissue histologic specimens from 294 categorized male corpses aged 10–93 years. For data analysis and classification we used modern machine learning methods: k-NN, SVM, logistic regression, CatBoost, SGD, naive Bayes, random forest, nonlinear dimensionality reduction methods (t-SNE and uMAP), and recursive feature elimination for feature selection.
Results. The used techniques (algorithms) provided effective representation of a complex data set (76 histomorphometricfeatures), allowing to reveal the cluster structure inside the low dimensional feature space, thus fitting the classifier becomes even more reasonable. During feature selection, we estimated their importance for age group classification and studied the relationship between classification quality and the number of features inside the feature space. Data pre-processing made it possible to get rid of noise and keep most informative features, thereby accelerating a learning process and improving the classification quality. Data projection showed more well-defined cluster structure in the space of selected features. The accuracy of establishing certain groups was equal to 90%. It proves high efficiency of machine learning techniques used for forensic age diagnostics based on histomorphometric findings.
- Garvin H., Passalacqua N.V., Uh N.M., Gipson D.R., Overbury R.S., Cabo L.L. Developments in forensic anthropology: age-at-death estimation. In: Dirkmaat D.C. (editor). A companion to forensic anthropology. Chichester: Wiley-Blackwell; 2012; p. 202–223, https://doi.org/10.1002/9781118255377.ch10.
- Glybochko P.V., Pigolkin Yu.I., Nikolenko V.N., Zolotenkova G.V., Efimov A.A., Alekseev Yu.D., Fedulova M.V., Savenkova E.N., Kurzin L.M., Goncharova N.N., Yurchenko M.A., Miroshnichenko N.V. Sudebno-meditsinskaya diagnostika vozrasta [Forensic diagnostics of age]. Moscow: Pervyy MGMU imeni I.M. Sechenova; 2016.
- Schmitt A., Murail P., Cunha E., Rougé D. Variability of the pattern of aging on the human skeleton: evidence from bone indicators and implications on age at death estimation. J Forensic Sci 2002; 47(6): 1203–1209, https://doi.org/10.1520/jfs15551j.
- Mays S. The effect of factors other than age upon skeletal age indicators in the adult. Ann Hum Biol 2015; 42(4): 332–341, https://doi.org/10.3109/03014460.2015.1044470.
- Ferrante L., Skrami E., Gesuita R., Cameriere R. Bayesian calibration for forensic age estimation. Stat Med 2015; 34(10): 1779–1790, https://doi.org/10.1002/sim.6448.
- Bucci A., Skrami E., Faragalli A., Gesuita R., Cameriere R., Carle F., Ferrante L. Segmented Bayesian calibration approach for estimating age in forensic science. Biom J 2019; 61(6): 1575–1594, https://doi.org/10.1002/bimj.201900016.
- Hartnett K.M. Analysis of age-at-death estimation using data from a new, modern autopsy sample — part I: pubic bone. J Forensic Sci 2010; 55(5): 1145–1151, https://doi.org/10.1111/j.1556-4029.2010.01399.x.
- Pigolkin Yu.I., Zolotenkova G.V., Sereda A.P., Zolotenkov D.D., Gridina N.V. Histometric symptoms of age-sensitive changes of bone tissue. Adv Gerontol 2018; 31(2): 203–210.
- Pigolkin Yu.I., Poletaeva M.P., Zolotenkova G.V., Volkov A.V. The age-specific changes in the histological structure of the thyroid cartilage in the men. Sudebno-medicinskaja ekspertiza 2017; 60(5): 11–14, https://doi.org/10.17116/sudmed201760511-14.
- Pigolkin Yu.I., Poletaeva М.P., Zolotenkova G.V. Age determine by the age of the thyroid cartilage by the radiological method in forensic medicine. Rossijskij ehlektronnyj zhurnal luchevoj diagnostiki 2017; 7(4): 23–29, https://doi.org/10.21569/2222-7415-2017-7-4-23-29.
- Pigolkin Yu.I., Zolotenkova G.V., Berezovskii D.P. Methodological basis for determining a person’s age. Sudebno-meditsinskaya ekspertisa 2020; 63(3): 45–50, https://doi.org/10.17116/sudmed20206303145.
- Kovalev А.V., Аmetrin M.D., Zolotenkova G.V., Gerasimov А.N., Gornostaev D.V., Poletaeva M.P. Forensic medical determination of the age based on the analysis of CT-scanograms of the skull and the craniovertebral region in the sagittal projection. Sudebno-meditsinskaya ekspertisa 2018; 61(1): 21–27, https://doi.org/10.17116/sudmed201861121-27.
- Pigolkin Yu.I., Tkachenko S.B., Zolotenkova G.V., Velenko P.S., Zolotenkov D.D., Safroneeva Yu.L. The comprehensive evaluation of the age-specific changes in the skin. Sudebno-meditsinskaya ekspertisa 2018; 61(3): 15–18, https://doi.org/10.17116/sudmed201861315-18.
- Pigolkin Yu.I., Zolotenkova G.V. Age-specific changes in the cerebral cortex capillaries. Sudebno-meditsinskaya ekspertisa 2014; 57(1): 4–10.
- Buk Z., Kordik P., Bruzek J., Schmitt А., Snorek М. The age at death assessment in a multi-ethnic sample of pelvic bones using nature-inspired data mining methods. Forensic Sci Int 2012; 220(1–3): 294.e1–294.e9, https://doi.org/10.1016/j.forsciint.2012.02.019.
- Moskalenko V.A., Nikolskiy A.V., Zolotykh N.Yu., Kozlov A.A., Kosonogov K.A., Kalyakulina A.I., Yusipov I.I., Levanov V.M. Cyberheart-diagnostics software package for automated electrocardiogram analysis based on machine learning techniques. Sovremennye tehnologii v medicine 2019; 11(2): 86–91, https://doi.org/10.17691/stm2019.11.2.12.
- Andryushchenko V.S., Uglov A.S., Zamyatin A.V. Statistical classification of immunosignatures under significant reduction of the feature space dimensions for early diagnosis of diseases. Sovremennye tehnologii v medicine 2018; 10(3): 14–20, https://doi.org/10.17691/stm2018.10.3.2.
- Samoyavcheva S.V., Shkarin V.V. Capabilities of cluster analysis in interpretation of 24-hour blood pressure monitoring data in patients with arterial hypertension and left ventricular remodeling. Sovremennye tehnologii v medicine 2015; 7(4): 113–118, https://doi.org/10.17691/stm2015.7.4.15.
- Kerley E.R. The microscopic determination of age in human bone. Am J Phys Anthropol 1965; 23(2): 149–164, https://doi.org/10.1002/ajpa.1330230215.
- Stout S.D. The use of cortical bone histology to estimate age at death. In: Işcan M.Y. (editor). Age markers in the human skeleton. Springfield: Charles C. Thomas; 1989, https://doi.org/10.1002/ajhb.1310030516.
- Crowder C.M., Pfeiffer S. The application of cortical bone histomorphometry to estimate age at death. In: Latham K.E., Finnegan J.M., Rhine S. (editors). Age estimation of the human skeleton. Springfield: Charles C. Thomas; 2010.
- Crowder C.M., Dominguez V.M. A new method for histological age estimation of the femur. In: Proceedings of the American Academy of Forensic Sciences; Vol. 18. Atlanta; 2012; p. 374–375.
- Doyle E., Márquez-Grant N., Field L., Holmes T., Arthurs O.J., van Rijn R.R., Hackman L., Kasper K., Lewis J., Loomis P., Elliott D., Kroll J., Viner M., Blau S., Brough A., de las Heras S.M., Garamendi P.M. Guidelines for best practice: imaging for age estimation in the living. J Forensic Radiol Imaging 2019; 16: 38–49, https://doi.org/10.1016/j.jofri.2019.02.001.
- Crowder C. Evaluating the use of quantitative bone histology to estimate adult age at death. PhD Thesis. Toronto: University of Toronto, Department of Anthropology; 2005.