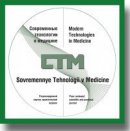
Sample Size Calculation for Clinical Trials of Medical Decision Support Systems with Binary Outcome
Currently, software products for use in medicine are actively developed. Among them, the dominant share belongs to clinical decision support systems (CDSS), which can be intelligent (based on mathematical models obtained by machine learning methods or other artificial intelligence technologies) or non-intelligent. For the state registration of CDSSs as software medical products, clinical trials are required, and the protocol of trial is developed jointly by the developer and an authorized medical organization. One of the mandatory components of the protocol is the calculation of the sample size.
This article discusses the calculation of the sample size for the most common case, the binary outcome in diagnostic/screening and predictive systems. For diagnostic/screening models, cases of a non-comparative study, comparative study with testing of the superiority hypothesis, comparative study with testing of a hypothesis of non-inferiority in cross-sectional studies are considered. For predictive models, cases of randomized controlled trials of the complex intervention “prediction + prediction-dependent patient management” with testing of the hypothesis of superiority and non-inferiority are considered.
It is emphasized that representativeness of the sample and other design components are no less important in clinical trials than sample size. They are even more important since systematic biases in clinical trials are primary, and even the most sophisticated statistical analysis cannot compensate for design defects. The reduction of clinical trials to external validation of models (i.e. evaluation of accuracy metrics on external data) seems completely unreasonable. It is recommended to perform clinical trials with the design adequate to the tasks, so that further clinical and economic analysis and comprehensive assessment of medical technologies are possible.
The sample size calculation methods described in the article can potentially be applied to a wider range of medical devices.
- Gusev A.V., Morozov S.P., Kutichev V.A., Novitsky R.E. Legal regulation of artificial intelligence software in healthcare in the Russian Federation. Medicinskie tehnologii. Ocenka i vybor 2021; 1: 36–45, https://doi.org/10.17116/medtech20214301136.
- Prikaz Ministerstva zdravookhraneniya RF ot 30 avgusta 2021 g. No.885 “Ob utverzhdenii Poryadka otsenki sootvetstviya meditsinskikh izdeliy v forme tekhnicheskikh ispytaniy, toksikologicheskikh issledovaniy, klinicheskikh ispytaniy v tselyakh gosudarstvennoy registratsii meditsinskikh izdeliy” [Order of the Ministry of Health of the Russian Federation of August 30, 2021 No.885 “On approval of the Procedure for assessing the conformity of medical devices in the form of technical tests, toxicological studies, clinical trials for the purpose of state registration of medical devices”]. URL: https://docs.cntd.ru/document/608935477.
- MDRF/SaMD WG/N41FINAL:2017. Software as a Medical Device (SaMD): Clinical Evaluation. URL: http://www.imdrf.org/docs/imdrf/ final/technical/imdrf-tech-170921-samd- n41-clinical-evaluation_1.pdf.
- Wallert J., Tomasoni M., Madison G., Held C. Predicting two-year survival versus non-survival after first myocardial infarction using machine learning and Swedish national register data. BMC Med Inform Decis Mak 2017; 17(1): 99, https://doi.org/10.1186/s12911-017-0500-y.
- Ye C., Fu T., Hao S., Zhang Y., Wang O., Jin B., Xia M., Liu M., Zhou X., Wu Q., Guo Y., Zhu C., Li Y.M., Culver D.S., Alfreds S.T., Stearns F., Sylvester K.G., Widen E., McElhinney D., Ling X. Prediction of incident hypertension within the next year: prospective study using statewide electronic health records and machine learning. J Med Internet Res 2018; 20(1): e22, https://doi.org/10.2196/jmir.9268.
- Park J., Kim J.W., Ryu B., Heo E., Jung S.Y., Yoo S. Patient-level prediction of cardio-cerebrovascular events in hypertension using nationwide claims data. J Med Internet Res 2019; 21(2): e11757, https://doi.org/10.2196/11757.
- Rebrova O.Yu. Life cycle of decision support systems as medical technologies. Vrac i informacionnye tehnologii 2020; 1: 27–37, https://doi.org/10.37690/1811-0193-2020-1-27-37.
- Bossuyt P.M., Reitsma J.B., Bruns D.E., Gatsonis C.A., Glasziou P.P., Irwig L., Lijmer J.G., Moher D., Rennie D., de Vet H.C.W., Kressel H.Y., Rifai N., Golub R.M., Altman D.G., Hooft L., Korevaar D.A., Cohen J.F.; STARD Group. STARD 2015: an updated list of essential items for reporting diagnostic accuracy studies. BMJ 2015; 351: h5527, https://doi.org/10.1136/bmj.h5527.
- Collins G.S., Reitsma J.B., Altman D.G., Moons K.G. Transparent reporting of a multivariable prediction model for individual prognosis or diagnosis (TRIPOD): the TRIPOD statement. BMJ 2015; 350: g7594, https://doi.org/10.1136/bmj.g7594.
- Snell K.I.E., Archer L., Ensor J., Bonnet L., Debray T.P.A., Philips B., Collins G.S., Riley R.D. External validation of clinical prediction models: simulation-based sample size calculations were more reliable than rules-of-thumb. J Clin Epidemiol 2021; 135: 79–89, https://doi.org/10.1016/j.jclinepi.2021.02.011.
- Riley R.D., Debray T.P.A., Collins G.S., Archer L., Ensor J., van Smeden M., Snell K.I.E. Minimum sample size for external validation of a clinical prediction model with a binary outcome. Stat Med 2021; 40(19): 4230–4251, https://doi.org/10.1002/sim.9025.
- Archer L., Snell K.I.E., Ensor J., Hudda M.T., Collins G.S., Riley R.D. Minimum sample size for external validation of a clinical prediction model with a continuous outcome. Stat Med 2021; 40(1): 133–146, https://doi.org/10.1002/sim.8766.
- Riley R.D., Collins G.S., Ensor J., Archer L., Booth S., Mozumder S.I., Rutherford M.J., van Smeden M., Lambert P.C., Snell K.I.E. Minimum sample size calculations for external validation of a clinical prediction model with a time-to-event outcome. Stat Med 2022; 41(7): 1280–1295, https://doi.org/10.1002/sim.9275.
- Feng D., Cortese G., Baumgartner R. A comparison of confidence/credible interval methods for the area under the ROC curve for continuous diagnostic tests with small sample size. Stat Methods Med Res 2017; 26(6): 2603–2621, https://doi.org/10.1177/0962280215602040.