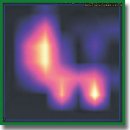
Methodology for Conducting Post-Marketing Surveillance of Software as a Medical Device Based on Artificial Intelligence Technologies
The aim of the study was to develop a methodology for conducting post-registration clinical monitoring of software as a medical device based on artificial intelligence technologies (SaMD-AI).
Materials and Methods. The methodology of post-registration clinical monitoring is based on the requirements of regulatory legal acts issued by the Board of the Eurasian Economic Commission. To comply with these requirements, the monitoring involves submission of the review of adverse events reports, the review of developers’ routine reports on the safety and efficiency of SaMD-AI, and the assessment of the system for collecting and analyzing developers’ post-registration data on the safety and efficiency of medical devices. The methodology was developed with regard to the recommendations of the International Medical Device Regulators Forum and the documents issued by the Food and Drug Administration (USA). Field-testing of this methodology was carried out using SaMD-AI designed for diagnostic imaging.
Results. The post-registration monitoring of SaMD-AI consists of three key stages: collecting user feedback, technical monitoring and clinical validation. Technical monitoring involves routine evaluation of SaMD-AI output data quality to detect and remove flaws in a timely manner, and to secure the product stability. Major outcomes include an ordered list of technical flaws in SaMD-AI and their classification using evidence from diagnostic imaging studies. The application of this methodology resulted in a gradual reduction in the number of studies with flaws due to timely improvements in artificial intelligence algorithms: the number of flaws decreased to 5% in various aspects during subsequent testing. Clinical validation confirmed that SaMD-AI is capable of producing clinically meaningful outputs related to its intended use within the functionality determined by the developer. The testing procedure and the baseline testing framework were established during the field testing.
Conclusion. The developed methodology will ensure the safety and efficiency of SaMD-AI taking into account its specifics as intangible medical devices. The methodology presented in this paper can be used by SaMD-AI developers to plan and carry out the post-registration clinical monitoring.
- Guidance for post-market surveillance and market surveillance of medical devices, including in-vitro-diagnostics. URL: https://www.who.int/docs/default-source/essential- medicines/in-vitro-diagnostics/draft- public-pmsdevices.pdf?sfvrsn=f803f68a_2.
- Guidance on Clinical Evaluation (MDR) / Performance Evaluation (IVDR) of medical device software. URL: https://ec.europa.eu/health/sites/default/files/md_ sector/docs/md_mdcg_2020_1_guidance_ clinic_eva_md_software_en.pdf.
- Postmarket Surveillance Under Section 522 of the Federal Food, Drug, and Cosmetic Act. Guidance for Industry and Food and Drug Administration Staff. URL: https://www.fda.gov/media/81015/download.
- Federal’nyy zakon ot 21.11.2011 Nо.323-FZ “Ob osnovakh okhrany zdorov’ya grazhdan v Rossiyskoy Federatsii” (s izm. i dop., vstup. v silu s 01.10.2021) [Federal Law Nо.323-FZ of November 21, 2011 “On the fundamentals of protecting the health of citizens in the Russian Federation” (as amended and supplemented, effective from October 1, 2021)].
- Prikaz Minzdrava Rossii ot 14.09.2012 Nо.175n “Ob utverzhdenii Poryadka osushchestvleniya monitoringa bezopasnosti meditsinskikh izdeliy” (zaregistrirovan Minyustom Rossii 25.12.2012 No.26356) [Order of the Ministry of Health of Russia dated September 14, 2012 Nо.175n “On approval of the procedure for monitoring the safety of medical devices” (registered by the Ministry of Justice of Russia on December 25, 2012 No.26356)].
- Reshenie Kollegii Evraziyskoy ekonomicheskoy komissii ot 22 dekabrya 2015 g. Nо.174 “Ob utverzhdenii Pravil provedeniya monitoringa bezopasnosti, kachestva i effektivnosti meditsinskikh izdeliy” [Decision of the Board of the Eurasian Economic Commission dated December 22, 2015 Nо.174 “On approval of the Rules for monitoring the safety, quality and effectiveness of medical devices”].
- Karpov O.E., Klimenko G.S., Lebedev G.S. Application of intelligent systems in health care. Sovremennye naukoemkie tehnologii 2016; 7–1: 38–43.
- Gusev A.V., Dobridnyuk S.L. Artificial intelligence in medicine and healthcare. Informacionnoe obsestvo 2017; 4–5: 78–93.
- Benjamens S., Dhunnoo P., Meskó B. The state of artificial intelligence-based FDA-approved medical devices and algorithms: an online database. NPJ Digit Med 2020; 3(1): 118, https://doi.org/10.1038/s41746-020-00324-0.
- Kelly C.J., Karthikesalingam A., Suleyman M., Corrado G., King D. Key challenges for delivering clinical impact with artificial intelligence. BMC Med 2019; 17(1): 195, https://doi.org/10.1186/s12916-019-1426-2.
- Proposed Regulatory Framework for Modifications to Artificial Intelligence Machine Learning (AI ML)-Based Software as a Medical Device (SaMD). Discussion paper and request for feedback. URL: https://www.fda.gov/media/122535/download.
- Prikaz MZ RF ot 6 iyunya 2012 g. Nо.4n “Ob utverzhdenii nomenklaturnoy klassifikatsii meditsinskikh izdeliy” [Order of the Ministry of Health of the Russian Federation of June 6, 2012 Nо.4n “On approval of the nomenclature classification of medical devices”].
- Harvey H.B., Gowda V. How the FDA regulates AI. Acad Radiol 2020; 27(1): 58–61, https://doi.org/10.1016/j.acra.2019.09.017.
- Shuren J.; IMDRF Chair. “Software as a Medical Device”: possible framework for risk categorization and corresponding considerations. 2014. URL: https://www.imdrf.org/sites/default/files/docs/ imdrf/final/technical/imdrf-tech-140918-samd- framework-risk-categorization-141013.pdf.
- Higgins D.C. OnRAMP for regulating artificial intelligence in medical products. Adv Intell Syst 2021; 3(11): 2100042, https://doi.org/10.1002/aisy.202100042.
- Software as a Medical Device (SaMD) Action Plan. URL: https://www.fda.gov/media/145022/download.
- Software as a Medical Device (SaMD): Clinical Evaluation. URL: https://www.fda.gov/regulatory-information/search- fda-guidance-documents/software-medical-device-samd- clinical-evaluation.
- General Principles of Software Validation; Final Guidance for Industry and FDA Staff. URL: https://www.fda.gov/files/medical%20devices/ published/General-Principles-of-Software-Validation--- Final-Guidance-for-Industry-and-FDA-Staff.pdf.
- Higgins D., Madai V.I. From bit to bedside: a practical framework for artificial intelligence product development in healthcare. Adv Intell Syst 2020; 2: 2000052, https://doi.org/10.1002/aisy.202000052.
- Sounderajah V., Ashrafian H., Golub R.M., Shetty S., De Fauw J., Hooft L., Moons K., Collins G., Moher D., Bossuyt P.M., Darzi A., Karthikesalingam A., Denniston A.K., Mateen B.A., Ting D., Treanor D., King D., Greaves F., Godwin J., Pearson-Stuttard J., Harling L., McInnes M., Rifai N., Tomasev N., Normahani P., Whiting P., Aggarwal R., Vollmer S., Markar S.R., Panch T., Liu X.; STARD-AI Steering Committee. Developing a reporting guideline for artificial intelligence-centred diagnostic test accuracy studies: the STARD-AI protocol. BMJ Open 2021; 11(6): e047709, https://doi.org/10.1136/bmjopen-2020-047709.
- Morozov S.P., Vladzymyrskyy A.V., Ledikhova N.V., Andrejchenko A.E., Arzamasov K.M., Balanjuk E.A., Gombolevskij V.A., Ermolaev S.O., Zhivodenko V.S., Idrisov I.M., Kirpichev Ju.S., Logunova T.A., Nuzhdina V.A., Omeljanskaja O.V., Rakovchen V.G., Slepushkina A.V. Moscow experiment on computer vision in radiology: involvement and participation of radiologists. Vrac i informacionnye tehnologii 2020; 4: 14–23, https://doi.org/10.37690/1811-0193-2020-4-14-23.
- Eksperiment po ispol’zovaniyu innovatsionnykh tekhnologiy v oblasti komp’yuternogo zreniya dlya analiza meditsinskikh izobrazheniy i dal’neyshego primeneniya v sisteme zdravookhraneniya goroda Moskvy [Experiment on the use of innovative computer vision technologies for medical image analysis and subsequent applicability in the healthcare system of Moscow]. URL: https://mosmed.ai/ai/.
- Morozov S.P., Vladzymyrskyy A.V., Klyashtornyy V.G., Andreychenko A.E., Kulberg N.S., Gombolevsky V.A., Sergunova K.A. Klinicheskie ispytaniya programmnogo obespecheniya na osnove intellektual'nykh tekhnologiy (luchevaya diagnostika). V kn.: Seriya “Luchshie praktiki luchevoy i instrumental'noy diagnostiki”. Vypusk 57 [Clinical acceptance of software based on artificial intelligence technologies (radiology). In: Series “Best practices in medical imaging”. Issue 57]. Moscow; 2019; 51 p.
- Andreychenko A.E., Logunova T.A., Gombolevskiy V.A., Nikolaev A.E., Vladzymyrskyy A.V., Sinitsyn V.E., Morozov S.P. A methodology for selection and quality control of the radiological computer vision deployment at the megalopolis scale. medRxiv; 2022, https://doi.org/10.1101/2022.02.12.22270663.
- Ying G.S., Maguire M.G., Glynn R.J., Rosner B. Calculating sensitivity, specificity, and predictive values for correlated eye data. Investig Ophthalmol Vis Sci 2020; 61(11): 29, https://doi.org/10.1167/iovs.61.11.29.
- Arroyo-Palacios J., Rudz M., Fidler R., Smith W., Ko N., Park S., Bai Y., Hu X. Characterization of shape differences among ICP pulses predicts outcome of external ventricular drainage weaning trial. Neurocrit Care 2016; 25(3): 424–433, https://doi.org/10.1007/s12028-016-0268-4.
- Narkevich A.N., Vinogradov K.A. Methods for determining the minimum required sample size in medical research. Social’nye aspekty zdorov’a naselenia 2019; 65(6): 10.
- Pepe M.S. Chapter 8: study design and hypothesis testing. In: The statistical evaluation of medical tests for classification and prediction. New York: Oxford University Press; 2003; p. 319.
- Pavlov N.A., Andreychenko A.E., Vladzymyrskyy A.V., Revazyan A.A., Kirpichev Y.S., Morozov S.P. Reference medical datasets (MosMedData) for independent external evaluation of algorithms based on artificial intelligence in diagnostics. Digital Diagnostics 2021; 2(1): 49–66.
- Zinchenko V.V., Khoruzhaya A.N., Sharova D.E., Akhmad E.S., Mokienko O.A., Vladzymyrskyy A.V., Morozov S.P. Standardization in regulating artificial intelligence systems in Russian healthcare. Kazanskij medicinskij zurnal 2021; 102(6): 923–933.
- GOST R 59921.5—2022 “Sistemy iskusstvennogo intellekta v klinicheskoy meditsine. Chast’ 5. Trebovaniya k strukture i poryadku primeneniya nabora dannykh dlya obucheniya i testirovaniya algoritmov” [GOST R 59921.5—2022 “Artificial intelligence systems in clinical medicine. Part 5. Requirements for the structure and order of using a data set for training and testing algorithms”].
- Park Y., Jackson G.P., Foreman M.A., Gruen D., Hu J., Das A.K. Evaluating artificial intelligence in medicine: phases of clinical research. JAMIA Open 2020; 3(3): 326–331, https://doi.org/10.1093/jamiaopen/ooaa033.
- Health Sciences Authority Singapore. Regulatory Guidelines for Software Medical Devices — a Life Cycle Approach. Revision 2.0. URL: https://www.hsa.gov.sg/docs/default-source/hprg-mdb/ gudiance-documents-for-medical-devices/regulatory-guidelines-for- software-medical-devices---a-life-cycle-approach_r2-(2022-apr)-pub.pdf.
- Zinchenko V., Chetverikov S., Akhmad E., Arzamasov K., Vladzymyrskyy A., Andreychenko A., Morozov S. Changes in software as a medical device based on artificial intelligence technologies. Int J Comput Assist Radiol Surg 2022; 17(10): 1969–1977, https://doi.org/10.1007/s11548-022-02669-1.
- Ronquillo J.G., Zuckerman D.M. Software-related recalls of health information technology and other medical devices: implications for FDA regulation of digital health. Milbank Q 2017; 95(3): 535–553, https://doi.org/10.1111/1468-0009.12278.
- ISO/TR 20416:2020. Medical devices — Post-market surveillance for manufacturers. URL: https://www.iso.org/standard/67942.html.
- Article 78 — Post-market surveillance system of the manufacturer. URL: https://lexparency.org/eu/32017R0746/ART_78/.
- GOST R 59921.2—2021 “Sistemy iskusstvennogo intellekta v klinicheskoy meditsine. Chast’ 2. Programma i metodika tekhnicheskikh ispytaniy” [GOST R 59921.2—2021 “Artificial intelligence systems in clinical medicine. Part 2. Program and methodology of technical validation”].
- GOST R 59921.3—2021 “Sistemy iskusstvennogo intellekta v klinicheskoy meditsine. Chast’ 3. Upravlenie izmeneniyami v sistemakh iskusstvennogo intellekta s nepreryvnym obucheniem” [GOST R 59921.3—2021 “Artificial intelligence systems in clinical medicine. Part 3. Change management in Al systems with lifelong learning capability”].
- GOST R 59921.4—2021 “Sistemy iskusstvennogo intellekta v klinicheskoy meditsine. Chast’ 4. Otsenka i kontrol’ ekspluatatsionnykh parametrov” [GOST R 59921.4—2021 “Artificial intelligence systems in clinical medicine. Part 4. Evaluation and control of performance parameters”].
- GOST R 59921.6—2021 “Sistemy iskusstvennogo intellekta v klinicheskoy meditsine. Chast’ 6. Obshchie trebovaniya k ekspluatatsii” [GOST R 59921.6—2021 “Artificial intelligence systems in clinical medicine. Part 6. General performance requirements”].