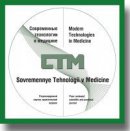
Diagnosis of Schizophrenia Based on the Data of Various Modalities: Biomarkers and Machine Learning Techniques (Review)
Schizophrenia is a socially significant mental disorder resulting frequently in severe forms of disability. Diagnosis, choice of treatment tactics, and rehabilitation in clinical psychiatry are mainly based on the assessment of behavioral patterns, socio-demographic data, and other investigations such as clinical observations and neuropsychological testing including examination of patients by the psychiatrist, self-reports, and questionnaires. In many respects, these data are subjective and therefore a large number of works have appeared in recent years devoted to the search for objective characteristics (indices, biomarkers) of the processes going on in the human body and reflected in the behavioral and psychoneurological patterns of patients. Such biomarkers are based on the results of instrumental and laboratory studies (neuroimaging, electro-physiological, biochemical, immunological, genetic, and others) and are successfully being used in neurosciences for understanding the mechanisms of the emergence and development of nervous system pathologies.
Presently, with the advent of new effective neuroimaging, laboratory, and other methods of investigation and also with the development of modern methods of data analysis, machine learning, and artificial intelligence, a great number of scientific and clinical studies is being conducted devoted to the search for the markers which have diagnostic and prognostic value and may be used in clinical practice to objectivize the processes of establishing and clarifying the diagnosis, choosing and optimizing treatment and rehabilitation tactics, predicting the course and outcome of the disease.
This review presents the analysis of the works which describe the correlates between the diagnosis of schizophrenia, established by health professionals, various manifestations of the psychiatric disorder (its subtype, variant of the course, severity degree, observed symptoms, etc.), and objectively measured characteristics/quantitative indicators (anatomical, functional, immunological, genetic, and others) obtained during instrumental and laboratory examinations of patients.
A considerable part of these works has been devoted to correlates/biomarkers of schizophrenia based on the data of structural and functional (at rest and under cognitive load) MRI, EEG, tractography, and immunological data. The found correlates/biomarkers reflect anatomic disorders in the specific brain regions, impairment of functional activity of brain regions and their interconnections, specific microstructure of the brain white matter and the levels of connectivity between the tracts of various structures, alterations of electrical activity in various parts of the brain in different EEG spectral ranges, as well as changes in the innate and adaptive links of immunity.
Current methods of data analysis and machine learning to search for schizophrenia biomarkers using the data of diverse modalities and their application during building and interpretation of predictive diagnostic models of schizophrenia have been considered in the present review.
- Jaeschke K., Hanna F., Ali S., Chowdhary N., Dua T., Charlson F. Global estimates of service coverage for severe mental disorders: findings from the WHO Mental Health Atlas 2017. Glob Ment Health (Camb) 2021; 8: e27, https://doi.org/10.1017/gmh.2021.19.
- Harvey P.D., Heaton R.K., Carpenter W.T. Jr., Green M.F., Gold J.M., Schoenbaum M. Diagnosis of schizophrenia: consistency across information sources and stability of the condition. Schizophr Res 2012; 140(1–3): 9–14, https://doi.org/10.1016/j.schres.2012.03.026.
- de Gracia Dominguez M., Viechtbauer W., Simons C.J., van Os J., Krabbendam L. Are psychotic psychopathology and neurocognition orthogonal? A systematic review of their associations. Psychol Bull 2009; 135(1): 157–171, https://doi.org/10.1037/a0014415.
- Doi N., Hoshi Y., Itokawa M., Yoshikawa T., Ichikawa T., Arai M., Usui C., Tachikawa H. Paradox of schizophrenia genetics: is a paradigm shift occurring? Behav Brain Funct 2012; 8: 28, https://doi.org/10.1186/1744-9081-8-28.
- George B., Klijn A. Psychosis susceptibility syndrome: an alternative name for schizophrenia. Lancet Psychiatry 2014; 1(2): 110–111, https://doi.org/10.1016/s2215-0366(14)70249-4.
- Marder S.R., Galderisi S. The current conceptualization of negative symptoms in schizophrenia. World Psychiatry 2017; 16(1): 14–24, https://doi.org/10.1002/wps.20385.
- Galderisi S., Mucci A., Buchanan R.W., Arango C. Negative symptoms of schizophrenia: new developments and unanswered research questions. Lancet Psychiatry 2018; 5(8): 664–677, https://doi.org/10.1016/s2215-0366(18)30050-6.
- Foussias G., Agid O., Fervaha G., Remington G. Negative symptoms of schizophrenia: clinical features, relevance to real world functioning and specificity versus other CNS disorders. Eur Neuropsychopharmacol 2014; 24(5): 693–709, https://doi.org/10.1016/j.euroneuro.2013.10.017.
- Nakagami E., Xie B., Hoe M., Brekke J.S. Intrinsic motivation, neurocognition and psychosocial functioning in schizophrenia: testing mediator and moderator effects. Schizophr Res 2008; 105(1–3): 95–104, https://doi.org/10.1016/j.schres.2008.06.015.
- Nakagami E., Hoe M., Brekke J.S. The prospective relationships among intrinsic motivation, neurocognition, and psychosocial functioning in schizophrenia. Schizophr Bull 2010; 36(5): 935–948, https://doi.org/10.1093/schbul/sbq043.
- Liang S., Vega R., Kong X., Deng W., Wang Q., Ma X., Li M., Hu X., Greenshaw A.J., Greiner R., Li T. Neurocognitive graphs of first-episode schizophrenia and major depression based on cognitive features. Neurosci Bull 2018; 34(2): 312–320, https://doi.org/10.1007/s12264-017-0190-6.
- Javanbakht A. A brief review of a brain model for schizophrenia and other psychotic disorders which might suggest novel treatment modalities. Clin Schizophr Relat Psychoses 2007; 1(3): 283–285.
- Padmanabhan J.L., Tandon N., Haller C.S., Mathew I.T., Eack S.M., Clementz B.A., Pearlson G.D., Sweeney J.A., Tamminga C.A., Keshavan M.S. Correlations between brain structure and symptom dimensions of psychosis in schizophrenia, schizoaffective, and psychotic bipolar I disorders. Schizophr Bull 2015; 41(1): 154–162, https://doi.org/10.1093/schbul/sbu075.
- McKnight R., Price J., Geddes J. Schizophrenia and related psychotic disorders. In: Psychiatry. Oxford University Press; 2019, https://doi.org/10.1093/oso/9780198754008.003.0030.
- Peralta V., Cuesta M.J. How many and which are the psychopathological dimensions in schizophrenia? Issues influencing their ascertainment. Schizophr Res 2001; 49(3): 269–285, https://doi.org/10.1016/s0920-9964(00)00071-2.
- Strik W., Stegmayer K., Walther S., Dierks T. Systems neuroscience of psychosis: mapping schizophrenia symptoms onto brain systems. Neuropsychobiology 2017; 75: 100–116, https://doi.org/10.1159/000485221.
- Liddle P.F. The symptoms of chronic schizophrenia: a re-examination of the positive-negative dichotomy. Br J Psychiatry 1987; 151(2): 145–151, https://doi.org/10.1192/bjp.151.2.145.
- Mortimer A.M., Lund C.E., McKenna P.J. The positive: negative dichotomy in schizophrenia. Br J Psychiatry 1990; 157(1): 41–49, https://doi.org/10.1192/bjp.157.1.41.
- Demjaha A., Morgan K., Morgan C., Landau S., Dean K., Reichenberg A., Sham P., Fearon P., Hutchinson G., Jones P.B., Murray R.M., Dazzan P. Combining dimensional and categorical representation of psychosis: the way forward for DSM-V and ICD-11? Psychol Med 2009; 39(12): 1943–1955, https://doi.org/10.1017/s0033291709990651.
- Crow T.J. The two-syndrome concept: origins and current status. Schizophr Bull 1985; 11(3): 471–488, https://doi.org/10.1093/schbul/11.3.471.
- Liddle P.F., Barnes T.R., Morris D., Haque S. Three syndromes in chronic schizophrenia. Br J Psychiatry Suppl 1989; 7: 119–122.
- Lindenmayer J.P., Bernstein-Hyman R., Grochowski S., Bark N. Psychopathology of schizophrenia: initial validation of a 5-factor model. Psychopathology 1995; 28(1): 22–31, https://doi.org/10.1159/000284896.
- Lehman A.F. Improving treatment for persons with schizophrenia. Psychiatr Q 1999; 70(4): 259–272, https://doi.org/10.1023/a:1022082031007.
- Mucci A., Galderisi S., Amodio A., Dierks T. Neuroimaging of schizophrenia and other primary psychotic disorders. In: Neuroimaging and psychopathological domains. Galderisi S., DeLisi L.E., Borgwardt S. (editors). Cham: Springer International Publishing; 2019; p. 57–155. URL: https://link.springer.com/chapter/10.1007/978-3-319-97307-4_2.
- Bopp M.H.A., Zöllner R., Jansen A., Dietsche B., Krug A., Kircher T.T.J. White matter integrity and symptom dimensions of schizophrenia: a diffusion tensor imaging study. Schizophr Res 2017; 184: 59–68, https://doi.org/10.1016/j.schres.2016.11.045.
- Cuthbert B.N., Kozak M.J. Constructing constructs for psychopathology: the NIMH research domain criteria. J Abnorm Psychol 2013; 122(3): 928–937, https://doi.org/10.1037/a0034028.
- Cohen A.S., Le T.P., Fedechko T.L., Elvevåg B. Can RDoC help find order in thought disorder? Schizophr Bull 2017; 43(3): 503–508, https://doi.org/10.1093/schbul/sbx030.
- Biomarkers Definitions Working Group. Biomarkers and surrogate endpoints: preferred definitions and conceptual framework. Clin Pharmacol Ther 2001; 69(3): 89–95, https://doi.org/10.1067/mcp.2001.113989.
- First M.B., Drevets W.C., Carter C., Dickstein D.P., Kasoff L., Kim K.L., McConathy J., Rauch S., Saad Z.S., Savitz J., Seymour K.E., Sheline Y.I., Zubieta J.K. Clinical applications of neuroimaging in psychiatric disorders. Am J Psychiatry 2018; 175(9): 915–916, https://doi.org/10.1176/appi.ajp.2018.1750701.
- Hill A.B. The environment and disease: association or causation? Proc R Soc Med 1965; 58(5): 295–300.
- Takeda M., Tanaka T., Cacabelos R. (editors). Molecular neurobiology of Alzheimer disease and related disorders. Karger AG; 2004.
- Ho B.C., Andreasen N.C., Ziebell S., Pierson R., Magnotta V. Long-term antipsychotic treatment and brain volumes: a longitudinal study of first-episode schizophrenia. Arch Gen Psychiatry 2011; 68(2): 128–137, https://doi.org/10.1001/archgenpsychiatry.2010.199.
- Meyer-Lindenberg A. Neuroimaging and the question of neurodegeneration in schizophrenia. Prog Neurobiol 2011; 95(4): 514–516, https://doi.org/10.1016/j.pneurobio.2011.07.007.
- Fusar-Poli P., Smieskova R., Kempton M.J., Ho B.C., Andreasen N.C., Borgwardt S. Progressive brain changes in schizophrenia related to antipsychotic treatment? A meta-analysis of longitudinal MRI studies. Neurosci Biobehav Rev 2013; 37(8): 1680–1691, https://doi.org/10.1016/j.neubiorev.2013.06.001.
- Sarpal D.K., Malhotra A.K. Structural and functional neuroimaging biomarkers of antipsychotic treatment response in early-course and chronic schizophrenia. In: Neuroimaging in schizophrenia. Kubicki M., Shenton M.E. (editors). Cham: Springer International Publishing; 2020; p. 355–366. URL: https://link.springer.com/10.1007/978-3-030-35206-6_18.
- Weinberger D.R. Implications of normal brain development for the pathogenesis of schizophrenia. Arch Gen Psychiatry 1987; 44(7): 660, https://doi.org/10.1001/archpsyc.1987.01800190080012.
- Sallet P.C., Elkis H., Alves T.M., Oliveira J.R., Sassi E., Campi de Castro C., Busatto G.F., Gattaz W.F. Reduced cortical folding in schizophrenia: an MRI morphometric study. Am J Psychiatry 2003; 160(9): 1606–1613, https://doi.org/10.1176/appi.ajp.160.9.1606.
- Owen M.J., O’Donovan M.C., Thapar A., Craddock N. Neurodevelopmental hypothesis of schizophrenia. Br J Psychiatry 2011; 198(3): 173–175, https://doi.org/10.1192/bjp.bp.110.084384.
- Done D.J., Johnstone E.C., Frith C.D., Golding J., Shepherd P.M., Crow T.J. Complications of pregnancy and delivery in relation to psychosis in adult life: data from the British perinatal mortality survey sample. BMJ 1991; 302(6792): 1576–1580, https://doi.org/10.1136/bmj.302.6792.1576.
- Fish B., Marcus J., Hans S.L., Auerbach J.G., Perdue S. Infants at risk for schizophrenia: sequelae of a genetic neurointegrative defect: a review and replication analysis of pandysmaturation in the Jerusalem Infant Development Study. Arch Gen Psychiatry 1992; 49(3): 221–235, https://doi.org/10.1001/archpsyc.1992.01820030053007.
- Marcus J., Hans S.L., Auerbach J.G., Auerbach A.G. Children at risk for schizophrenia: the Jerusalem Infant Development Study: II. Neurobehavioral deficits at school age. Arch Gen Psychiatry 1993; 50(10): 797–809, https://doi.org/10.1001/archpsyc.1993.01820220053006.
- Crow T.J., Done D.J., Sacker A. Chidhood precursors of psychiosis as clues to its evolutionary origins. Eur Arch Psychiatry Clin Nuerosci 1995; 245(2): 61–69, https://doi.org/10.1007/bf02190732.
- Hirjak D., Meyer-Lindenberg A., Kubera K.M., Thomann P.A., Wolf R.C. Motor dysfunction as research domain in the period preceding manifest schizophrenia: a systematic review. Neurosci Biobehav Rev 2018; 87: 87–105, https://doi.org/10.1016/j.neubiorev.2018.01.011.
- Owen M.J., O’Donovan M.C. Schizophrenia and the neurodevelopmental continuum: evidence from genomics. World Psychiatry 2017; 16(3): 227–235, https://doi.org/10.1002/wps.20440.
- The Alzheimer’s Disease Neuroimaging Initiative; EPIGEN Consortium; IMAGEN Consortium; Saguenay Youth Study (SYS) Group. The ENIGMA Consortium: large-scale collaborative analyses of neuroimaging and genetic data. Brain Imaging Behav 2014; 8(2): 153–182, https://doi.org/10.1007/s11682-013-9269-5.
- van Erp T.G., Hibar D.P., Rasmussen J.M., Glahn D.C., Pearlson G.D., Andreassen O.A., Agartz I., Westlye L.T., Haukvik U.K., Dale A.M., Melle I., Hartberg C.B., Gruber O., Kraemer B., Zilles D., Donohoe G., Kelly S., McDonald C., Morris D.W., Cannon D.M., Corvin A., Machielsen M.W., Koenders L., de Haan L., Veltman D.J., Satterthwaite T.D., Wolf D.H., Gur R.C., Gur R.E., Potkin S.G., Mathalon D.H., Mueller B.A., Preda A., Macciardi F., Ehrlich S., Walton E., Hass J., Calhoun V.D., Bockholt H.J., Sponheim S.R., Shoemaker J.M., van Haren N.E., Pol H.E., Ophoff R.A., Kahn R.S., Roiz-Santiañez R., Crespo-Facorro B., Wang L., Alpert K.I., Jönsson E.G., Dimitrova R., Bois C., Whalley H.C., McIntosh A.M., Lawrie S.M., Hashimoto R., Thompson P.M., Turner J.A. Subcortical brain volume abnormalities in 2028 individuals with schizophrenia and 2540 healthy controls via the ENIGMA consortium. Mol Psychiatry 2016; 21(4): 547–553, https://doi.org/10.1038/mp.2015.118.
- Birnbaum R., Weinberger D.R. Functional neuroimaging and schizophrenia: a view towards effective connectivity modeling and polygenic risk. Dialogues Clin Neurosci 2013; 15(3): 279–289, https://doi.org/10.31887/dcns.2013.15.3/rbirnbaum.
- Abi-Dargham A., Horga G. The search for imaging biomarkers in psychiatric disorders. Nat Med 2016; 22(11): 1248–1255, https://doi.org/10.1038/nm.4190.
- Hugdahl K. Auditory hallucinations: a review of the ERC “VOICE” project. World J Psychiatry 2015; 5(2): 193–209, https://doi.org/10.5498/wjp.v5.i2.193.
- Galderisi S., DeLisi L.E., Borgwardt S. (editors). Neuroimaging of schizophrenia and other primary psychotic disorders: achievements and perspectives. Cham: Springer International Publishing; 2019. URL: https://link.springer.com/10.1007/978-3-319-97307-4.
- Allen P., Larøi F., McGuire P.K., Aleman A. The hallucinating brain: a review of structural and functional neuroimaging studies of hallucinations. Neurosci Biobehav Rev 2008; 32(1): 175–191, https://doi.org/10.1016/j.neubiorev.2007.07.012.
- Jardri R., Pouchet A., Pins D., Thomas P. Cortical activations during auditory verbal hallucinations in schizophrenia: a coordinate-based meta-analysis. Am J Psychiatry 2011; 168(1): 73–81, https://doi.org/10.1176/appi.ajp.2010.09101522.
- Modinos G., Costafreda S.G., van Tol M.J., McGuire P.K., Aleman A., Allen P. Neuroanatomy of auditory verbal hallucinations in schizophrenia: a quantitative meta-analysis of voxel-based morphometry studies. Cortex 2013; 49(4): 1046–1055, https://doi.org/10.1016/j.cortex.2012.01.009.
- Downar J., Crawley A.P., Mikulis D.J., Davis K.D. A multimodal cortical network for the detection of changes in the sensory environment. Nat Neurosci 2000; 3(3): 277–283, https://doi.org/10.1038/72991.
- Nenadic I., Smesny S., Schlösser R.G.M., Sauer H., Gaser C. Auditory hallucinations and brain structure in schizophrenia: voxel-based morphometric study. Br J Psychiatry 2010; 196(5): 412–413, https://doi.org/10.1192/bjp.bp.109.070441.
- Northoff G., Bermpohl F. Cortical midline structures and the self. Trends Cogn Sci 2004; 8(3): 102–107, https://doi.org/10.1016/j.tics.2004.01.004.
- Martí-Bonmatí L., Lull J.J., García-Martí G., Aguilar E.J., Moratal-Pérez D., Poyatos C., Robles M., Sanjuán J. Chronic auditory hallucinations in schizophrenic patients: MR analysis of the coincidence between functional and morphologic abnormalities. Radiology 2007; 244(2): 549–556, https://doi.org/10.1148/radiol.2442060727.
- García-Martí G., Aguilar E.J., Lull J.J., Martí-Bonmatí L., Escartí M.J., Manjón J.V., Moratal D., Robles M., Sanjuán J. Schizophrenia with auditory hallucinations: a voxel-based morphometry study. Prog Neuropsychopharmacol Biol Psychiatry 2008; 32(1): 72–80, https://doi.org/10.1016/j.pnpbp.2007.07.014.
- Zakharova N.V., Mamedova G.S., Bravve L.V., Kaydan M.A., Syunyakov T.S., Kostyuk G.P., Ushakov V.L. Brain gyrification index in schizophrenia (review, systematic review and meta-analysis). Procedia Comput Sci 2021; 190: 825–837, https://doi.org/10.1016/j.procs.2021.06.097.
- Crespo-Facorro B., Kim J., Andreasen N.C., O’Leary D.S., Bockholt H.J., Magnotta V. Insular cortex abnormalities in schizophrenia: a structural magnetic resonance imaging study of first-episode patients. Schizophr Res 2000; 46(1): 35–43, https://doi.org/10.1016/s0920-9964(00)00028-1.
- Crespo-Facorro B., Roiz-Santiáñez R., Quintero C., Pérez-Iglesias R., Tordesillas-Gutiérrez D., Mata I., Rodríguez-Sánchez J.M., Gutiérrez A., Vázquez-Barquero J.L. Insular cortex morphometry in first-episode schizophrenia-spectrum patients: diagnostic specificity and clinical correlations. J Psychiatr Res 2010; 44(5): 314–320, https://doi.org/10.1016/j.jpsychires.2009.08.014.
- Hubl D., Dougoud-Chauvin V., Zeller M., Federspiel A., Boesch C., Strik W., Dierks T., Koenig T. Structural analysis of Heschl’s gyrus in schizophrenia patients with auditory hallucinations. Neuropsychobiology 2010; 61(1): 1–9, https://doi.org/10.1159/000258637.
- Mangin J.F., Jouvent E., Cachia A. In-vivo measurement of cortical morphology: means and meanings. Curr Opin Neurol 2010; 23(4): 359–367, https://doi.org/10.1097/wco.0b013e32833a0afc.
- Cachia A., Paillère-Martinot M.L., Galinowski A., Januel D., de Beaurepaire R., Bellivier F., Artiges E., Andoh J., Bartrés-Faz D., Duchesnay E., Rivière D., Plaze M., Mangin J.F., Martinot J.L. Cortical folding abnormalities in schizophrenia patients with resistant auditory hallucinations. Neuroimage 2008; 39(3): 927–935, https://doi.org/10.1016/j.neuroimage.2007.08.049.
- Plaze M., Paillère-Martinot M.L., Penttilä J., Januel D., de Beaurepaire R., Bellivier F., Andoh J., Galinowski A., Gallarda T., Artiges E., Olié J.P., Mangin J.F., Martinot J.L., Cachia A. “Where do auditory hallucinations come from?” — a brain morphometry study of schizophrenia patients with inner or outer space hallucinations. Schizophr Bull 2011; 37(1): 212–221, https://doi.org/10.1093/schbul/sbp081.
- Federspiel A., Begré S., Kiefer C., Schroth G., Strik W.K., Dierks T. Alterations of white matter connectivity in first episode schizophrenia. Neurobiol Dis 2006; 22(3): 702–709, https://doi.org/10.1016/j.nbd.2006.01.015.
- Uranova N., Orlovskaya D., Vikhreva O., Zimina I., Kolomeets N., Vostrikov V., Rachmanova V. Electron microscopy of oligodendroglia in severe mental illness. Brain Res Bull 2001; 55(5): 597–610, https://doi.org/10.1016/s0361-9230(01)00528-7.
- Andreasen N.C., Nopoulos P., O’Leary D.S., Miller D.D., Wassink T., Flaum M. Defining the phenotype of schizophrenia: cognitive dysmetria and its neural mechanisms. Biol Psychiatry 1999; 46(7): 908–920, https://doi.org/10.1016/s0006-3223(99)00152-3.
- Davis K.L., Stewart D.G., Friedman J.I., Buchsbaum M., Harvey P.D., Hof P.R., Buxbaum J., Haroutunian V. White matter changes in schizophrenia: evidence for myelin-related dysfunction. Arch Gen Psychiatry 2003; 60(5): 443–456, https://doi.org/10.1001/archpsyc.60.5.443.
- Kubicki M., Park H., Westin C.F., Nestor P.G., Mulkern R.V., Maier S.E., Niznikiewicz M., Connor E.E., Levitt J.J., Frumin M., Kikinis R., Jolesz F.A., McCarley R.W., Shenton M.E. DTI and MTR abnormalities in schizophrenia: analysis of white matter integrity. Neuroimage 2005; 26(4): 1109–1118, https://doi.org/10.1016/j.neuroimage.2005.03.026.
- Okugawa G., Nobuhara K., Minami T., Takase K., Sugimoto T., Saito Y., Yoshimura M., Kinoshita T. Neural disorganization in the superior cerebellar peduncle and cognitive abnormality in patients with schizophrenia: a diffusion tensor imaging study. Prog Neuropsychopharmacol Biol Psychiatry 2006; 30(8): 1408–1412, https://doi.org/10.1016/j.pnpbp.2006.05.014.
- Oestreich L.K., Pasternak O., Shenton M.E., Kubicki M., Gong X.; Australian Schizophrenia Research Bank, McCarthy-Jones S., Whitford T.J. Abnormal white matter microstructure and increased extracellular free-water in the cingulum bundle associated with delusions in chronic schizophrenia. Neuroimage Clin 2016; 12: 405–414, https://doi.org/10.1016/j.nicl.2016.08.004.
- Zhou Y., Fan L., Qiu C., Jiang T. Prefrontal cortex and the dysconnectivity hypothesis of schizophrenia. Neurosci Bull 2015; 31(2): 207–219, https://doi.org/10.1007/s12264-014-1502-8.
- de Weijer A.D., Mandl R.C., Diederen K.M., Neggers S.F., Kahn R.S., Hulshoff Pol H.E., Sommer I.E. Microstructural alterations of the arcuate fasciculus in schizophrenia patients with frequent auditory verbal hallucinations. Schizophr Res 2011; 130(1–3): 68–77, https://doi.org/10.1016/j.schres.2011.05.010.
- de Weijer A.D., Neggers S.F., Diederen K.M., Mandl R.C., Kahn R.S., Hulshoff Pol H.E., Sommer I.E. Aberrations in the arcuate fasciculus are associated with auditory verbal hallucinations in psychotic and in non-psychotic individuals. Hum Brain Mapp 2013; 34(3): 626–634, https://doi.org/10.1002/hbm.21463.
- Vercammen A., Knegtering H., Bruggeman R., Aleman A. Subjective loudness and reality of auditory verbal hallucinations and activation of the inner speech processing network. Schizophr Bull 2011; 37(5): 1009–1016, https://doi.org/10.1093/schbul/sbq007.
- Raij T.T., Valkonen-Korhonen M., Holi M., Therman S., Lehtonen J., Hari R. Reality of auditory verbal hallucinations. Brain 2009; 132(Pt 11): 2994–3001, https://doi.org/10.1093/brain/awp186.
- Escartí M.J., de la Iglesia-Vayá M., Martí-Bonmatí L., Robles M., Carbonell J., Lull J.J., García-Martí G., Manjón J.V., Aguilar E.J., Aleman A., Sanjuán J. Increased amygdala and parahippocampal gyrus activation in schizophrenic patients with auditory hallucinations: an fMRI study using independent component analysis. Schizophr Res 2010; 117(1): 31–41, https://doi.org/10.1016/j.schres.2009.12.028.
- Kang J.I., Kim J.J., Seok J.H., Chun J.W., Lee S.K., Park H.J. Abnormal brain response during the auditory emotional processing in schizophrenic patients with chronic auditory hallucinations. Schizophr Res 2009; 107(1): 83–91, https://doi.org/10.1016/j.schres.2008.08.019.
- Diederen K.M., Neggers S.F., Daalman K., Blom J.D., Goekoop R., Kahn R.S., Sommer I.E. Deactivation of the parahippocampal gyrus preceding auditory hallucinations in schizophrenia. Am J Psychiatry 2010; 167(4): 427–435, https://doi.org/10.1176/appi.ajp.2009.09040456.
- Shergill S.S., Bullmore E., Simmons A., Murray R., McGuire P. Functional anatomy of auditory verbal imagery in schizophrenic patients with auditory hallucinations. Am J Psychiatry 2000; 157(10): 1691–1693, https://doi.org/10.1176/appi.ajp.157.10.1691.
- Diederen K.M.J., Daalman K., de Weijer A.D., Neggers S.F.W., van Gastel W., Blom J.D., Kahn R.S., Sommer I.E. Auditory hallucinations elicit similar brain activation in psychotic and nonpsychotic individuals. Schizophr Bull 2012; 38(5): 1074–1082, https://doi.org/10.1093/schbul/sbr033.
- Linden D.E.J., Thornton K., Kuswanto C.N., Johnston S.J., van de Ven V., Jackson M.C. The brain’s voices: comparing nonclinical auditory hallucinations and imagery. Cereb Cortex 2011; 21(2): 330–337, https://doi.org/10.1093/cercor/bhq097.
- Vinogradov S., Luks T.L., Schulman B.J., Simpson G.V. Deficit in a neural correlate of reality monitoring in schizophrenia patients. Cereb Cortex 2008; 18(11): 2532–2539, https://doi.org/10.1093/cercor/bhn028.
- Lawrie S.M., Buechel C., Whalley H.C., Frith C.D., Friston K.J., Johnstone E.C. Reduced frontotemporal functional connectivity in schizophrenia associated with auditory hallucinations. Biol Psychiatry 2002; 51(12): 1008–1011, https://doi.org/10.1016/s0006-3223(02)01316-1.
- Mechelli A., Allen P., Amaro E. Jr., Fu C.H., Williams S.C., Brammer M.J., Johns L.C., McGuire P.K. Misattribution of speech and impaired connectivity in patients with auditory verbal hallucinations. Hum Brain Mapp 2007; 28(11): 1213–1222, https://doi.org/10.1002/hbm.20341.
- Wang L., Metzak P.D., Woodward T.S. Aberrant connectivity during self-other source monitoring in schizophrenia. Schizophr Res 2011; 125(2–3): 136–142, https://doi.org/10.1016/j.schres.2010.11.012.
- Vercammen A., Knegtering H., den Boer J.A., Liemburg E.J., Aleman A. Auditory hallucinations in schizophrenia are associated with reduced functional connectivity of the temporo-parietal area. Biol Psychiatry 2010; 67(10): 912–918, https://doi.org/10.1016/j.biopsych.2009.11.017.
- Gavrilescu M., Rossell S., Stuart G.W., Shea T.L., Innes-Brown H., Henshall K., McKay C., Sergejew A.A., Copolov D., Egan G.F. Reduced connectivity of the auditory cortex in patients with auditory hallucinations: a resting state functional magnetic resonance imaging study. Psychol Med 2010; 40(7): 1149–1158, https://doi.org/10.1017/s0033291709991632.
- Hoffman R.E., Fernandez T., Pittman B., Hampson M. Elevated functional connectivity along a corticostriatal loop and the mechanism of auditory/verbal hallucinations in patients with schizophrenia. Biol Psychiatry 2011; 69(5): 407–414, https://doi.org/10.1016/j.biopsych.2010.09.050.
- Galderisi S., Mucci A., Volpe U., Boutros N. Evidence-based medicine and electrophysiology in schizophrenia. Clin EEG Neurosci 2009; 40(2): 62–77, https://doi.org/10.1177/155005940904000206.
- Medkour T., Walden A.T., Burgess A.P., Strelets V.B. Brain connectivity in positive and negative syndrome schizophrenia. Neuroscience 2010; 169(4): 1779–1788, https://doi.org/10.1016/j.neuroscience.2010.05.060.
- Hare S.M., Ford J.M., Mathalon D.H., Damaraju E., Bustillo J., Belger A., Lee H.J., Mueller B.A., Lim K.O., Brown G.G., Preda A., van Erp T.G.M., Potkin S.G., Calhoun V.D., Turner J.A. Salience–default mode functional network connectivity linked to positive and negative symptoms of schizophrenia. Schizophr Bull 2019; 45(4): 892–901, https://doi.org/10.1093/schbul/sby112.
- Ford J.M., Mathalon D.H. Electrophysiological evidence of corollary discharge dysfunction in schizophrenia during talking and thinking. J Psychiatr Res 2004; 38(1): 37–46, https://doi.org/10.1016/s0022-3956(03)00095-5.
- Park S.M., Jeong B., Oh D.Y., Choi C.H., Jung H.Y., Lee J.Y., Lee D., Choi J.S. Identification of major psychiatric disorders from resting-state electroencephalography using a machine learning approach. Front Psychiatry 2021; 12: 707581, https://doi.org/10.3389/fpsyt.2021.707581.
- Kim J.Y., Lee H.S., Lee S.H. EEG source network for the diagnosis of schizophrenia and the identification of subtypes based on symptom severity — a machine learning approach. J Clin Med 2020; 9(12): 3934, https://doi.org/10.3390/jcm9123934.
- Ferri J., Ford J.M., Roach B.J., Turner J.A., van Erp T.G., Voyvodic J., Preda A., Belger A., Bustillo J., O’Leary D., Mueller B.A., Lim K.O., McEwen S.C., Calhoun V.D., Diaz M., Glover G., Greve D., Wible C.G., Vaidya J.G., Potkin S.G., Mathalon D.H. Resting-state thalamic dysconnectivity in schizophrenia and relationships with symptoms. Psychol Med 2018; 48(15): 2492–2499, https://doi.org/10.1017/s003329171800003x.
- Tortella-Feliu M., Morillas-Romero A., Balle M., Llabrés J., Bornas X., Putman P. Spontaneous EEG activity and spontaneous emotion regulation. Int J Psychophysiol 2014; 94(3): 365–372, https://doi.org/10.1016/j.ijpsycho.2014.09.003.
- Jang K.I., Kim S., Kim S.Y., Lee C., Chae J.H. Machine learning-based electroencephalographic phenotypes of schizophrenia and major depressive disorder. Front Psychiatry 2021; 12: 745458, https://doi.org/10.3389/fpsyt.2021.745458.
- Vázquez M.A., Maghsoudi A., Mariño I.P. An interpretable machine learning method for the detection of schizophrenia using EEG signals. Front Syst Neurosci 2021; 15: 652662, https://doi.org/10.3389/fnsys.2021.652662.
- Ke P.F., Xiong D.S., Li J.H., Pan Z.L., Zhou J., Li S.J., Song J., Chen X.Y., Li G.X., Chen J., Li X.B., Ning Y.P., Wu F.C., Wu K. An integrated machine learning framework for a discriminative analysis of schizophrenia using multi-biological data. Sci Rep 2021; 11(1): 14636, https://doi.org/10.1038/s41598-021-94007-9.
- Tikka S., Singh B., Nizamie Sh., Garg S., Mandal S., Thakur K., Singh LK. Artificial intelligence-based classification of schizophrenia: a high density electroencephalographic and support vector machine study. Indian Journal of Psychiatry 2020; 62(3): 273, https://doi.org/10.4103/psychiatry.indianjpsychiatry_91_20.
- The Schizophrenia Psychiatric Genome-Wide Association Study (GWAS) Consortium. Genome-wide association study identifies five new schizophrenia loci. Nat Genet 2011; 43(10): 969–976, https://doi.org/10.1038/ng.940.
- de Witte L.D., van Mierlo H.C., Litjens M., Klein H.C., Bahn S., Osterhaus A.D.; GROUP Investigators. The association between antibodies to neurotropic pathogens and schizophrenia: a case-control study. NPJ Schizophr 2015; 1: 15041, https://doi.org/10.1038/npjschz.2015.41.
- Goldsmith D.R., Rapaport M.H., Miller B.J. A meta-analysis of blood cytokine network alterations in psychiatric patients: comparisons between schizophrenia, bipolar disorder and depression. Mol Psychiatry 2016; 21(12): 1696–1709, https://doi.org/10.1038/mp.2016.3.
- Johnsen E., Fathian F., Kroken R.A., Steen V.M., Jørgensen H.A., Gjestad R., Løberg E.M. The serum level of C-reactive protein (CRP) is associated with cognitive performance in acute phase psychosis. BMC Psychiatry 2016; 16: 60, https://doi.org/10.1186/s12888-016-0769-x.
- Malashenkova I.K., Krynskiy S.A., Ogurtsov D.P., Mamoshina M.V., Zakharova N.V., Ushakov V.L., Velichkovsky B.M., Didkovsky N.A. A role of the immune system in the pathogenesis of schizophrenia. Zhurnal nevrologii i psihiatrii imeni S.S. Korsakova 2018; 118(12): 72–80, https://doi.org/10.17116/jnevro201811812172.
- Malashenkova I.K., Ushakov V.L., Zakharova N.V., Krynskiy S.A., Ogurtsov D.P., Hailov N.A., Chekulaeva E.I., Ratushnyy A.Y., Kartashov S.I., Kostyuk G.P., Didkovsky N.A. Neuro-immune aspects of schizophrenia with severe negative symptoms: new diagnostic markers of disease phenotype. Sovremennye tehnologii v medicine 2021; 13(6): 24, https://doi.org/10.17691/stm2021.13.6.03.
- Tomasik J., Rahmoune H., Guest P.C., Bahn S. Neuroimmune biomarkers in schizophrenia. Schizophr Res 2016; 176(1): 3–13, https://doi.org/10.1016/j.schres.2014.07.025.
- Fond G., Lançon C., Korchia T., Auquier P., Boyer L. The role of inflammation in the treatment of schizophrenia. Front Psychiatry 2020; 11: 160, https://doi.org/10.3389/fpsyt.2020.00160.
- Ribeiro-Santos R., de Campos-Carli S.M., Ferretjans R., Teixeira-Carvalho A., Martins-Filho O.A., Teixeira A.L., Salgado J.V. The association of cognitive performance and IL-6 levels in schizophrenia is influenced by age and antipsychotic treatment. Nord J Psychiatry 2020; 74(3): 187–193, https://doi.org/10.1080/08039488.2019.1688389.
- Zhang Q., He H., Cao B., Gao R., Jiang L., Zhang X., Dai J. Analysis of cognitive impairment in schizophrenia based on machine learning: interaction between psychological stress and immune system. Neurosci Lett 2021; 760: 136084, https://doi.org/10.1016/j.neulet.2021.136084.
- Al-Hakeim H.K., Almulla A.F., Al-Dujaili A.H., Maes M. Construction of a neuro-immune-cognitive pathway-phenotype underpinning the phenome of deficit schizophrenia. Curr Top Med Chem 2020; 20(9): 747–758, https://doi.org/10.2174/1568026620666200128143948.
- Wu D., Lv P., Li F., Zhang W., Fu G., Dai J., Hu N., Liu J., Xiao Y., Li S., Shah C., Tao B., Zhao Y., Gong Q., Lui S. Association of peripheral cytokine levels with cerebral structural abnormalities in schizophrenia. Brain Res 2019; 1724: 146463, https://doi.org/10.1016/j.brainres.2019.146463.
- O’Connell K.S., Sønderby I.E., Frei O., van der Meer D., Athanasiu L., Smeland O.B., Alnæs D., Kaufmann T., Westlye L.T., Steen V.M., Andreassen O.A., Hughes T., Djurovic S. Association between complement component 4A expression, cognitive performance and brain imaging measures in UK Biobank. Psychol Med 2021, https://doi.org/10.1017/s0033291721000179. Online ahead of print.
- Lizano P., Lutz O., Xu Y., Rubin L.H., Paskowitz L., Lee A.M., Eum S., Keedy S.K., Hill S.K., Reilly J.L., Wu B., Tamminga C.A., Clementz B.A., Pearlson G.D., Gershon E.S., Keshavan M.S., Sweeney J.A., Bishop J.R. Multivariate relationships between peripheral inflammatory marker subtypes and cognitive and brain structural measures in psychosis. Mol Psychiatry 2021; 26(7): 3430–3443, https://doi.org/10.1038/s41380-020-00914-0.
- Sharaev M., Artemov A., Kondrateva E., Sushchinskaya S., Burnaev E., Bernstein A., Akzhigitov R., Andreev A. MRI-based diagnostics of depression concomitant with epilepsy: in search of the potential biomarkers. In: 2018 IEEE 5th international conference on data science and advanced analytics (DSAA). Turin: IEEE; 2018; p. 555–564. URL: https://ieeexplore.ieee.org/document/8631407.
- Sharaev M., Andreev A., Artemov A., Burnaev E., Kondratyeva E., Sushchinskaya S., Samotaeva I., Gaskin V., Bernstein A. Pattern recognition pipeline for neuroimaging data. In: Artificial neural networks in pattern recognition. Pancioni L., Schwenker F., Trentin E. (editors). Cham: Springer International Publishing; 2018; p. 306–319. URL: https://link.springer.com/10.1007/978-3-319-99978-4_24.
- Varoquaux G., Gramfort A., Poline J.B., Thirion B. Brain covariance selection: better individual functional connectivity models using population prior. Advances in Neural Information Processing Systems 2010. URL: https://arxiv.org/abs/1008.5071.
- Wang X., Ren Y., Zhang W. Depression disorder classification of fMRI data using sparse low-rank functional brain network and graph-based features. Comput Math Methods Med 2017; 2017: 3609821, https://doi.org/10.1155/2017/3609821.
- Sharaev M., Artemov A., Kondrateva E., Ivanov S., Sushchinskaya S., Bernstein A., Cichocki A., Burnaev E. Learning connectivity patterns via graph kernels for fMRI-based depression diagnostics. In: 2018 IEEE International Conference on data Mining Workshops (ICDMW). Singapore: IEEE; 2018; p. 308–314. URL: https://ieeexplore.ieee.org/document/8637372.
- Pominova M., Artemov A., Sharaev M., Kondrateva E., Bernstein A., Burnaev E. Voxelwise 3D convolutional and recurrent neural networks for epilepsy and depression diagnostics from structural and functional MRI data. In: 2018 IEEE International Conference on data Mining Workshops (ICDMW). Singapore: IEEE; 2018; p. 299–307. URL: https://ieeexplore.ieee.org/document/8637478.
- Dvornek N.C., Ventola P., Pelphrey K.A., Duncan J.S. Identifying autism from resting-state fMRI using long short-term memory networks. Mach Learn Med Imaging 2017; 10541: 362–370, https://doi.org/10.1007/978-3-319-67389-9_42.
- Dakka J., Bashivan P., Gheiratmand M., Rish I., Jha S., Greiner R. Learning neural markers of schizophrenia disorder using recurrent neural networks. arXiv 2017. URL: http://arxiv.org/abs/1712.00512.
- Gabeur V., Sun C., Alahari K., Schmid C. Multi-modal transformer for video retrieval. Lecture Notes in Computer Science 2020, https://doi.org/10.1007/978-3-030-58548-8_13.
- Xu Y., Xu Y., Lv T., Cui L., Wei F., Wang G., Lu Y., Florencio D., Zhang C., Che W., Zhang M., Zhou L. LayoutLMv2: multi-modal pre-training for visually-rich document understanding. Proceedings of the 59th Annual Meeting of the Association for Computational Linguistics and the 11th International Joint Conference on Natural Language Processing (Volume 1: Long Papers) 2021, https://doi.org/10.18653/v1/2021.acl-long.201.
- Ramesh A., Pavlov M., Goh G., Gray S., Voss C., Radford A., Chen M., Sutskever I. Zero-shot text-to-image generation. International Conference on Machine Learning. PMLR 2021. URL: https://arxiv.org/abs/2102.12092.
- Jaegle A., Gimeno F., Brock A., Zisserman A., Vinyals O., Carreira J. Perceiver: general perception with iterative attention. International Conference on Machine Learning. PMLR 2021. URL: https://arxiv.org/abs/2103.03206.
- Jaegle A., Borgeaud S., Alayrac J.B., Doersch C., Ionescu C., Ding D., Koppula S., Zoran D., Brock A., Shelhamer E., Hénaff O., Botvinick M.M., Zisserman A., Vinyals O., Carreira J. Perceiver IO: a general architecture for structured inputs & outputs. arXiv 2021. URL: https://arxiv.org/abs/2107.14795.
- Tay Y., Dehghani M., Aribandi V., Gupta J., Pham P., Qin Z., Bahri D., Juan D.C., Metzler D. OmniNet: omnidirectional representations from transformers. International Conference on Machine Learning. PMLR 2021. URL: https://arxiv.org/abs/2103.01075.
- Suk H.I., Lee S.W., Shen D.; Alzheimer’s Disease Neuroimaging Initiative. Hierarchical feature representation and multimodal fusion with deep learning for AD/MCI diagnosis. Neuroimage 2014; 101: 569–582, https://doi.org/10.1016/j.neuroimage.2014.06.077.
- Venugopalan J., Tong L., Hassanzadeh H.R., Wang M.D. Multimodal deep learning models for early detection of Alzheimer’s disease stage. Sci Rep 2021; 11(1): 3254, https://doi.org/10.1038/s41598-020-74399-w.
- Calhoun V.D., Sui J. Multimodal fusion of brain imaging data: a key to finding the missing link(s) in complex mental illness. Biol Psychiatry Cogn Neurosci Neuroimaging 2016; 1(3): 230–244, https://doi.org/10.1016/j.bpsc.2015.12.005.
- Skudlarski P., Jagannathan K., Anderson K., Stevens M.C., Calhoun V.D., Skudlarska B.A., Pearlson G. Brain connectivity is not only lower but different in schizophrenia: a combined anatomical and functional approach. Biol Psychiatry 2010; 68(1): 61–69, https://doi.org/10.1016/j.biopsych.2010.03.035.
- Isobe M., Miyata J., Hazama M., Fukuyama H., Murai T., Takahashi H. Multimodal neuroimaging as a window into the pathological physiology of schizophrenia: current trends and issues. Neurosci Res 2016; 102: 29–38, https://doi.org/10.1016/j.neures.2015.07.009.
- Salgado-Pineda P., Junqué C., Vendrell P., Baeza I., Bargalló N., Falcón C., Bernardo M. Decreased cerebral activation during CPT performance. Neuroimage 2004; 21(3): 840–847, https://doi.org/10.1016/j.neuroimage.2003.10.027.
- Plis S.M., Amin M.F., Chekroud A., Hjelm D., Damaraju E., Lee H.J., Bustillo J.R., Cho K., Pearlson G.D., Calhoun V.D. Reading the (functional) writing on the (structural) wall: multimodal fusion of brain structure and function via a deep neural network based translation approach reveals novel impairments in schizophrenia. Neuroimage 2018; 181: 734–747, https://doi.org/10.1016/j.neuroimage.2018.07.047.
- Rahaman M.A., Chen J., Fu Z., Lewis N., Iraji A., Calhoun V.D. Multi-modal deep learning of functional and structural neuroimaging and genomic data to predict mental illness. In: 2021 43rd Annual International Conference of the IEEE Engineering in Medicine & Biology Society (EMBC) 2021; p. 3267–3272, https://doi.org/10.1109/embc46164.2021.9630693.
- Fong R., Vedaldi A. Interpretable explanations of black boxes by meaningful perturbation. IEEE International Conference on Computer Vision (ICCV) 2017, https://doi.org/10.1109/iccv.2017.371.
- Zhou B., Khosla A., Lapedriza A., Oliva A., Torralba A. Learning deep features for discriminative localization. IEEE Conference on Computer Vision and Pattern Recognition (CVPR) 2016, https://doi.org/10.1109/cvpr.2016.319.
- Selvaraju R.R., Cogswell M., Das A., Vedantam R., Parikh D., Batra D. Grad-CAM: visual explanations from deep networks via gradient-based localization. Int J Comput Vis 2020; 128(2): 336–359, https://doi.org/10.1007/s11263-019-01228-7.
- Montavon G., Bach S., Binder A., Samek W., Müller K.R. Explaining nonlinear classification decisions with deep taylor decomposition. Pattern Recognit 2017; 65: 211–222, https://doi.org/10.1016/j.patcog.2016.11.008.
- Shrikumar A., Greenside P., Kundaje A. Learning important features through propagating activation differences. arXiv 2019. URL: https://arxiv.org/abs/1704.02685.
- Bach S., Binder A., Montavon G., Klauschen F., Müller K.R., Samek W. On pixel-wise explanations for non-linear classifier decisions by layer-wise relevance propagation. PLoS One 2015; 10(7): e0130140, https://doi.org/10.1371/journal.pone.0130140.
- Ribeiro M.T., Singh S., Guestrin C. “Why should i trust you?”: explaining the predictions of any classifier. Proceedings of the 22nd ACM SIGKDD International Conference on Knowledge Discovery and Data Mining 2016. URL: https://arxiv.org/abs/1602.04938.
- Petsiuk V., Das A., Saenko K. RISE: randomized input sampling for explanation of black-box models. British Machine Vision Conference 2018. URL: https://arxiv.org/abs/1806.07421.
- Kapishnikov A., Bolukbasi T., Viégas F., Terry M. XRAI: better attributions through regions. IEEE/CVF International Conference on Computer Vision 2019, https://doi.org/10.1109/iccv.2019.00505.
- Baumgartner C.F., Koch L.M., Tezcan K.C., Ang J.X., Konukoglu E. Visual feature attribution using Wasserstein GANs. IEEE/CVF Conference on Computer Vision and Pattern Recognition 2018, https://doi.org/10.1109/cvpr.2018.00867.
- Bass C., da Silva M., Sudre C., Tudosiu P.D., Smith S.M., Robinson E.C. ICAM: interpretable classification via disentangled representations and feature attribution mapping. Advances in Neural Information Processing Systems 2020. URL: https://arxiv.org/abs/2006.08287.