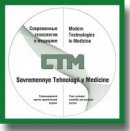
Artificial Intelligence Technologies in the Microsurgical Operating Room (Review)
Surgery performed by a novice neurosurgeon under constant supervision of a senior surgeon with the experience of thousands of operations, able to handle any intraoperative complications and predict them in advance, and never getting tired, is currently an elusive dream, but can become a reality with the development of artificial intelligence methods.
This paper has presented a review of the literature on the use of artificial intelligence technologies in the microsurgical operating room. Searching for sources was carried out in the PubMed text database of medical and biological publications. The key words used were “surgical procedures”, “dexterity”, “microsurgery” AND “artificial intelligence” OR “machine learning” OR “neural networks”. Articles in English and Russian were considered with no limitation to publication date. The main directions of research on the use of artificial intelligence technologies in the microsurgical operating room have been highlighted.
Despite the fact that in recent years machine learning has been increasingly introduced into the medical field, a small number of studies related to the problem of interest have been published, and their results have not proved to be of practical use yet. However, the social significance of this direction is an important argument for its development.
- Jian A., Jang K., Manuguerra M., Liu S., Magnussen J., Di Ieva A. Machine learning for the prediction of molecular markers in glioma on magnetic resonance imaging: a systematic review and meta-analysis. Neurosurgery 2021; 89(1): 31–44, https://doi.org/10.1093/neuros/nyab103.
- Litvin A.A., Burkin D.A., Kropinov A.A., Paramzin F.N. Radiomics and digital image texture analysis in oncology (review). Sovremennye tehnologii v medicine 2021; 13(2): 97, https://doi.org/10.17691/stm2021.13.2.11.
- Ning Z., Luo J., Xiao Q., Cai L., Chen Y., Yu X., Wang J., Zhang Y. Multi-modal magnetic resonance imaging-based grading analysis for gliomas by integrating radiomics and deep features. Ann Transl Med 2021; 9(4): 298, https://doi.org/10.21037/atm-20-4076.
- Lambin P., Rios-Velazquez E., Leijenaar R., Carvalho S., van Stiphout R.G., Granton P., Zegers C.M., Gillies R., Boellard R., Dekker A., Aerts H.J. Radiomics: extracting more information from medical images using advanced feature analysis. Eur J Cancer 2012; 48(4): 441–446, https://doi.org/10.1016/j.ejca.2011.11.036.
- Habib A., Jovanovich N., Hoppe M., Ak M., Mamindla P., Colen R.R., Zinn P.O. MRI-based radiomics and radiogenomics in the management of low-grade gliomas: evaluating the evidence for a paradigm shift. J Clin Med 2021; 10(7): 1411, https://doi.org/10.3390/jcm10071411.
- Cho H.H., Lee S.H., Kim J., Park H. Classification of the glioma grading using radiomics analysis. PeerJ 2018; 6: e5982, https://doi.org/10.7717/peerj.5982/supp-3.
- Su C., Jiang J., Zhang S., Shi J., Xu K., Shen N., Zhang J., Li L., Zhao L., Zhang J., Qin Y., Liu Y., Zhu W. Radiomics based on multicontrast MRI can precisely differentiate among glioma subtypes and predict tumour-proliferative behaviour. Eur Radiol 2019; 29(4): 1986–1996, https://doi.org/10.1007/s00330-018-5704-8.
- Cao X., Tan D., Liu Z., Liao M., Kan Y., Yao R., Zhang L., Nie L., Liao R., Chen S., Xie M. Differentiating solitary brain metastases from glioblastoma by radiomics features derived from MRI and 18F-FDG-PET and the combined application of multiple models. Sci Rep 2022; 12(1): 5722, https://doi.org/10.1038/s41598-022-09803-8.
- Qian J., Herman M.G., Brinkmann D.H., Laack N.N., Kemp B.J., Hunt C.H., Lowe V., Pafundi D.H. Prediction of MGMT status for glioblastoma patients using radiomics feature extraction from 18F-DOPA-PET imaging. Int J Radiat Oncol Biol Phys 2020; 108(5): 1339–1346, https://doi.org/10.1016/j.ijrobp.2020.06.073.
- Witten A.J., Patel N., Cohen-Gadol A. Image segmentation of operative neuroanatomy into tissue categories using a machine learning construct and its role in neurosurgical training. Oper Neurosurg (Hagerstown) 2022; 23(4): 279–286, https://doi.org/10.1227/ons.0000000000000322.
- Likhterman L.B. Healing: standards and art. Nejrohirurgia 2020; 22(2): 105–108, https://doi.org/10.17650/1683-3295-2020-22-2-105-108.
- Gusev E.I., Burd G.S., Konovalov A.N. Nevrologiya i neyrokhirurgiya [Neurology and neurosurgery]. Meditsina; 2000; URL: http://snsk.az/snsk/file/2013-05-9_11-31-06.pdf.
- Krylov V.V., Konovalov A.N., Dash’yan V.G., Kondakov E.N., Tanyashin S.V., Gorelyshev S.K., Dreval’ O.N., Grin’ A.A., Parfenov V.E., Kushniruk P.I., Gulyaev D.A., Kolotvinov V.S., Rzaev D.A., Poshataev K.E., Kravets L.Ya., Mozheiko R.A., Kas’yanov V.A., Kordonskii A.Yu., Trifonov I.S., Kalandari A.A., Shatokhin T.A., Airapetyan A.A., Dalibaldyan V.A., Grigor’ev I.V., Sytnik A.V. Neurosurgery in Russian Federation. Voprosy neirokhirurgii im. N.N. Burdenko 2017; 81(1): 5–12, https://doi.org/10.17116/neiro20178075-12.
- Bykanov A., Kiryushin M., Zagidullin T., Titov O., Rastvorova O. Effect of energy drinks on microsurgical hand tremor. Plast Reconstr Surg Glob Open 2021; 9(4): e3544, https://doi.org/10.1097/gox.0000000000003544.
- Coulson C.J., Slack P.S., Ma X. The effect of supporting a surgeon’s wrist on their hand tremor. Microsurgery 2010; 30(7): 565–568, https://doi.org/10.1002/micr.20776.
- Harada K., Morita A., Minakawa Y., Baek Y.M., Sora S., Sugita N., Kimura T., Tanikawa R., Ishikawa T., Mitsuishi M. Assessing microneurosurgical skill with medico-engineering technology. World Neurosurg 2015; 84(4): 964–971, https://doi.org/10.1016/j.wneu.2015.05.033.
- Applebaum M.A., Doren E.L., Ghanem A.M., Myers S.R., Harrington M., Smith D.J. Microsurgery competency during plastic surgery residency: an objective skills assessment of an integrated residency training program. Eplasty 2018;18: e25.
- Óvári A., Neményi D., Just T., Schuldt T., Buhr A., Mlynski R., Csókay A., Pau H.W., Valálik I. Positioning accuracy in otosurgery measured with optical tracking. PLoS One 2016; 11(3): e0152623, https://doi.org/10.1371/journal.pone.0152623.
- Satterwhite T., Son J., Carey J., Echo A., Spurling T., Paro J., Gurtner G., Chang J., Lee G.K. The Stanford Microsurgery and Resident Training (SMaRT) scale: validation of an on-line global rating scale for technical assessment. Ann Plast Surg 2014; 72(Suppl 1): S84–S88, https://doi.org/10.1097/sap.0000000000000139.
- Ward T.M., Mascagni P., Ban Y., Rosman G., Padoy N., Meireles O., Hashimoto D.A. Computer vision in surgery. Surgery 2021; 169(5): 1253–1256, https://doi.org/10.1016/j.surg.2020.10.039.
- Markarian N., Kugener G., Pangal D.J., Unadkat V., Sinha A., Zhu Y., Roshannai A., Chan J., Hung A.J., Wrobel B.B., Anandkumar A., Zada G., Donoho D.A. Validation of machine learning-based automated surgical instrument annotation using publicly available intraoperative video. Oper Neurosurg (Hagerstown) 2022; 23(3): 235–240, https://doi.org/10.1227/ons.0000000000000274.
- Jin A., Yeung S., Jopling J., Krause J., Azagury D., Milstein A., Fei-Fei L. Tool detection and operative skill assessment in surgical videos using region-based convolutional neural networks. In: Proc 2018 IEEE Winter Conf Appl Comput Vision WACV 2018; p. 691–699, https://doi.org/10.1109/wacv.2018.00081.
- Pangal D.J., Kugener G., Zhu Y., Sinha A., Unadkat V., Cote D.J., Strickland B., Rutkowski M., Hung A., Anandkumar A., Han X.Y., Papyan V., Wrobel B., Zada G., Donoho D.A. Expert surgeons and deep learning models can predict the outcome of surgical hemorrhage from 1 min of video. Sci Rep 2022; 12(1): 8137, https://doi.org/10.1038/s41598-022-11549-2.
- McGoldrick R.B., Davis C.R., Paro J., Hui K., Nguyen D., Lee G.K. Motion analysis for microsurgical training: objective measures of dexterity, economy of movement, and ability. Plast Reconstr Surg 2015; 136(2): 231e–240e, https://doi.org/10.1097/prs.0000000000001469.
- Franco-González I.T., Pérez-Escamirosa F., Minor-Martínez A., Rosas-Barrientos J.V., Hernández-Paredes T.J. Development of a 3D motion tracking system for the analysis of skills in microsurgery. J Med Syst 2021; 45(12): 106, https://doi.org/10.1007/s10916-021-01787-8.
- Oliveira M.M., Quittes L., Costa P.H.V., Ramos T.M., Rodrigues A.C.F., Nicolato A., Malheiros J.A., Machado C. Computer vision coaching microsurgical laboratory training: PRIME (Proficiency Index in Microsurgical Education) proof of concept. Neurosurg Rev 2022; 45(2): 1601–1606, https://doi.org/10.1007/s10143-021-01663-6.
- Wang J., Zhu H., Wang S.H., Zhang Y.D. A review of deep learning on medical image analysis. Mob Networks Appl 2020; 26: 351–380, https://doi.org/10.1007/s11036-020-01672-7.
- Du X., Kurmann T., Chang P.L., Allan M., Ourselin S., Sznitman R., Kelly J.D., Stoyanov D. Articulated multi-instrument 2-D pose estimation using fully convolutional networks. IEEE Trans Med Imaging 2018; 37(5): 1276–1287, https://doi.org/10.1109/tmi.2017.2787672.
- Twinanda A.P., Shehata S., Mutter D., Marescaux J., de Mathelin M., Padoy N. EndoNet: a deep architecture for recognition tasks on laparoscopic videos. IEEE Trans Med Imaging 2017; 36(1): 86–97, https://doi.org/10.1109/tmi.2016.2593957.
- Commowick O., Kain M., Casey R., Ameli R., Ferré J.C., Kerbrat A., Tourdias T., Cervenansky F., Camarasu-Pop S., Glatard T., Vukusic S., Edan G., Barillot C., Dojat M., Cotton F. Multiple sclerosis lesions segmentation from multiple experts: the MICCAI 2016 challenge dataset. Neuroimage 2021; 244: 118589, https://doi.org/10.1016/j.neuroimage.2021.118589.
- Gao Y., Vedula S.S., Reiley C.E., Ahmidi N., Varadarajan B., Lin H.C., Tao L., Zappella L., Béjar B., Yuh D.D., Chen C.C.G., Vidal R., Khudanpur S., Hager G.D. JHU-ISI Gesture And Skill Assessment Working Set (JIGSAWS): a surgical activity dataset for human motion modeling. 2022. URL: https://cirl.lcsr.jhu.edu/wp-content/uploads/2015/11/JIGSAWS.pdf.
- Sarikaya D., Corso J.J., Guru K.A. Detection and localization of robotic tools in robot-assisted surgery videos using deep neural networks for region proposal and detection. IEEE Trans Med Imaging 2017; 36(7): 1542–1549, https://doi.org/10.1109/tmi.2017.2665671.
- Yu T., Mutter D., Marescaux J., Padoy N. Learning from a tiny dataset of manual annotations: a teacher/student approach for surgical phase recognition. arXiv; 2018, https://doi.org/10.48550/arxiv.1812.00033.
- Ahmidi N., Poddar P., Jones J.D., Vedula S.S., Ishii L., Hager G.D., Ishii M. Automated objective surgical skill assessment in the operating room from unstructured tool motion in septoplasty. Int J Comput Assist Radiol Surg 2015; 10(6): 981–991, https://doi.org/10.1007/s11548-015-1194-1.
- Rosen J., Hannaford B., Richards C.G., Sinanan M.N. Markov modeling of minimally invasive surgery based on tool/tissue interaction and force/torque signatures for evaluating surgical skills. IEEE Trans Biomed Eng 2001; 48(5): 579–591, https://doi.org/10.1109/10.918597.
- Jiang J., Xing Y., Wang S., Liang K. Evaluation of robotic surgery skills using dynamic time warping. Comput Methods Programs Biomed 2017; 152: 71–83, https://doi.org/10.1016/j.cmpb.2017.09.007.
- Peng W., Xing Y., Liu R., Li J., Zhang Z. An automatic skill evaluation framework for robotic surgery training. Int J Med Robot 2019; 15(1): e1964, https://doi.org/10.1002/rcs.1964.
- Poursartip B., LeBel M.E., McCracken L.C., Escoto A., Patel R.V., Naish M.D., Trejos A.L. Energy-based metrics for arthroscopic skills assessment. Sensors (Basel) 2017; 17(8): 1808, https://doi.org/10.3390/s17081808.
- Gorantla K.R., Esfahani E.T. Surgical skill assessment using motor control features and hidden Markov model. Annu Int Conf IEEE Eng Med Biol Soc 2019; 2019: 5842–5845, https://doi.org/10.1109/embc.2019.8857629.
- Bissonnette V., Mirchi N., Ledwos N., Alsidieri G., Winkler-Schwartz A., Del Maestro R.F.; Neurosurgical Simulation & Artificial Intelligence Learning Centre. Artificial intelligence distinguishes surgical training levels in a virtual reality spinal task. J Bone Joint Surg Am 2019; 101(23): e127, https://doi.org/10.2106/jbjs.18.01197.
- Winkler-Schwartz A., Yilmaz R., Mirchi N., Bissonnette V., Ledwos N., Siyar S., Azarnoush H., Karlik B., Del Maestro R. Machine learning identification of surgical and operative factors associated with surgical expertise in virtual reality simulation. JAMA Netw Open 2019; 2(8): e198363, https://doi.org/10.1001/jamanetworkopen.2019.8363.
- Hung A.J., Chen J., Che Z., Nilanon T., Jarc A., Titus M., Oh P.J., Gill I.S., Liu Y. Utilizing machine learning and automated performance metrics to evaluate robot-assisted radical prostatectomy performance and predict outcomes. J Endourol 2018; 32(5): 438–444, https://doi.org/10.1089/end.2018.0035.
- Baghdadi A., Hussein A.A., Ahmed Y., Cavuoto L.A., Guru K.A. A computer vision technique for automated assessment of surgical performance using surgeons console-feed videos. Int J Comput Assist Radiol Surg 2019; 14(4): 697–707, https://doi.org/10.1007/s11548-018-1881-9.
- Yamaguchi T., Suzuki K., Nakamura R. Development of a visualization and quantitative assessment system of laparoscopic surgery skill based on trajectory analysis from USB camera image. Int J Comput Assist Radiol Surg 2016; 11(suppl): S254–S256.
- Weede O., Möhrle F., Wörn H., Falkinger M., Feussner H. Movement analysis for surgical skill assessment and measurement of ergonomic conditions. In: 2014 2nd International Conference on Artificial Intelligence, Modelling and Simulation. IEEE; 2014; p. 97–102, https://doi.org/10.1109/aims.2014.69.
- Kelly J.D., Petersen A., Lendvay T.S., Kowalewski T.M. Bidirectional long short-term memory for surgical skill classification of temporally segmented tasks. Int J Comput Assist Radiol Surg 2020; 15(12): 2079–2088, https://doi.org/10.1007/s11548-020-02269-x.
- Gahan J., Steinberg R., Garbens A., Qu X., Larson E. MP34-06 machine learning using a multi-task convolutional neural networks can accurately assess robotic skills. J Urol 2020; 203(Suppl 4): e505, https://doi.org/10.1097/ju.0000000000000878.06.
- Liu Y., Zhao Z., Shi P., Li F. Towards surgical tools detection and operative skill assessment based on deep learning. IEEE Trans Med. Robot Bionics 2022; 4(1): 62–71, https://doi.org/10.1109/tmrb.2022.3145672.