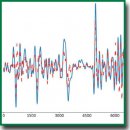
Memristive Neural Networks for Predicting Seizure Activity
The aim of the study is to assess the possibilities of predicting epileptiform activity using the neuronal activity data recorded from the hippocampus and medial entorhinal cortex of mice with chronic epileptiform activity. To reach this goal, a deep artificial neural network (ANN) has been developed and its implementation based on memristive devices has been demonstrated.
Materials and Methods
The biological part of the investigation. Young healthy outbred CD1 mice were used in our study. They were divided into two groups: control (n=6) and the group with induced chronic epileptiform activity (n=6). Local field potentials (LFP) were recorded from the hippocampus and medial entorhinal cortex of the mice of both groups to register neuronal activity. The LFP recordings were used for deep ANN training. Epileptiform activity in mice was modeled by intraperitoneal injection of pilocarpine (280 mg/kg). LFP were recorded in the awake mice a month after the induction of epileptiform activity.
Mathematical part of the investigation. A deep long short-term memory (LSTM) ANN capable of predicting biological signals of neuronal activity in mice has been developed. The ANN implementation is based on memristive devices, which are described by the equations of the redox processes running in the memristive thin metal–oxide–metal films, e.g., Au/ZrO2(Y)/TiN/Ti and Au/SiO2(Y)/TiN/Ti. In order to train the developed ANN to predict epileptiform activity, a supervised learning algorithm was used, which allowed us to adjust the network parameters and train LSTM on the described recordings of neuronal activity.
Results. After training on the LFP recordings from the hippocampus and medial entorhinal cortex of the mice with chronic epileptiform activity, the proposed deep ANN has demonstrated high values of evaluation metric (root-mean-square error, RMSE) and successfully predicted epileptiform activity shortly before its occurrence (40 ms). The results of the numerical experiments have shown that the RMSE value of 0.019 was reached, which indicates the efficacy of proposed approach. The accuracy of epileptiform activity prediction 40 ms before its occurrence is a significant result and shows the potential of the developed neural network architecture.
Conclusion. The proposed deep ANN can be used to predict pathological neuronal activity including epileptic seizure (focal) activity in mice before its actual occurrence. Besides, it can be applied for building a long-term prognosis of the disease course based on the LFP data. Thus, the proposed ANN based on memristive devices represents a novel approach to the prediction and analysis of pathological neuronal activity possessing a potential for improving the diagnosis and prognostication of epileptic seizures and other diseases associated with neuronal activity.
- Chang R.S., Leung C.Y.W., Ho C.C.A., Yung A. Classifications of seizures and epilepsies, where are we? — a brief historical review and update. J Formos Med Assoc 2017; 116(10): 736–741, https://doi.org/10.1016/j.jfma.2017.06.001.
- Pearce J.M.S. Bromide, the first effective antiepileptic agent. J Neurol Neurosurg Psychiatry 2002; 72(3): 412, https://doi.org/10.1136/jnnp.72.3.412.
- Mahaseth A., Lekhjung T. Child with intra cardiac masses and multiple seizure types. Rhabdomyoma, tuberous sclerosis and possible Lennox–Gastaut syndrome — a rare case report. Int J Cardiol Congenit Heart Dis 2023; 11: 100425, https://doi.org/10.1016/j.ijcchd.2022.100425.
- Knupp K.G., Scheffer I.E., Ceulemans B., Sullivan J., Nickels K.C., Lagae L., Guerrini R., Zuberi S.M., Nabbout R., Riney K., Agarwal A., Lock M., Dai D., Farfel G.M., Galer B.S., Gammaitoni A.R., Polega S., Davis R., Gil-Nagel A. Fenfluramine provides clinically meaningful reduction in frequency of drop seizures in patients with Lennox–Gastaut syndrome: interim analysis of an open-label extension study. Epilepsia 2023; 64(1): 139–151, https://doi.org/10.1111/epi.17431.
- Balfroid T., Warren A.E.L., Dalic L.J., Aeby A., Berlangieri S.U., Archer J.S. Frontoparietal 18F-FDG-PET hypo-metabolism in Lennox–Gastaut syndrome: further evidence highlighting the key network. Epilepsy Res 2023; 192: 107131, https://doi.org/10.1016/j.eplepsyres.2023.107131.
- Manral M., Dwivedi R., Gulati S., Kaur K., Nehra A., Pandey R.M., Upadhyay A.D., Sapra S., Tripathi M. Safety, efficacy, and tolerability of modified Atkins diet in persons with drug-resistant epilepsy: a randomized controlled trial. Neurology 2023; 100(13): e1376–e1385, https://doi.org/10.1212/wnl.0000000000206776.
- Mutanana N., Tsvere M., Chiweshe M.K. General side effects and challenges associated with anti-epilepsy medication: a review of related literature. Afr J Prim Health Care Fam Med 2020; 12(1): e1–e5, https://doi.org/10.4102/phcfm.v12i1.2162.
- Braun E., Gualano F.M., Siddarth P., Segal E. Second-line cannabis therapy in patients with epilepsy. Clin Neurol Neurosurg 2023; 227: 107638, https://doi.org/10.1016/j.clineuro.2023.107638.
- Suluhan D., Kose K., Yildiz D., Unay B. Attitudes toward rational drug use and medication self-management among parents of children with epilepsy. Jundishapur J Chronic Dis Care 2023; 12(1): e134446, https://doi.org/10.5812/jjcdc-134446.
- Donnan A.M., Schneider A.L., Russ-Hall S., Churilov L., Scheffer I.E. Rates of status epilepticus and sudden unexplained death in epilepsy in people with genetic developmental and epileptic encephalopathies. Neurology 2023; 100(16): e1712–e1722, https://doi.org/10.1212/wnl.0000000000207080.
- Gracie L., Rostami-Hochaghan D., Taweel B., Mirza N.; SAGAS Scientists’ Collaborative. The Seizure-Associated Genes Across Species (SAGAS) database offers insights into epilepsy genes, pathways and treatments. Epilepsia 2022; 63(9): 2403–2412, https://doi.org/10.1111/epi.17352.
- Deivasigamani S., Senthilpari C., Yong W.H. Retracted article: machine learning method based detection and diagnosis for epilepsy in EEG signal. J Ambient Intell Humaniz Comput 2021; 12: 4215–4221.
- Mir W.A., Anjum M., Izharuddin M., Shahab S. Deep-EEG: an optimized and robust framework and method for EEG-based diagnosis of epileptic seizure. Diagnostics (Basel) 2023; 13(4): 773, https://doi.org/10.3390/diagnostics13040773.
- Azzony S., Moria K., Alghamdi J. Detecting cortical thickness changes in epileptogenic lesions using machine learning. Brain Sci 2023; 13(3): 487, https://doi.org/10.3390/brainsci13030487.
- Jehi L. Machine learning for precision epilepsy surgery. Epilepsy Curr 2023; 23(2): 78–83, https://doi.org/10.1177/15357597221150055.
- Yao L., Cai M., Chen Y., Shen C., Shi L., Guo Y. Prediction of antiepileptic drug treatment outcomes of patients with newly diagnosed epilepsy by machine learning. Epilepsy Behav 2019; 96: 92–97, https://doi.org/10.1016/j.yebeh.2019.04.006.
- Hakeem H., Feng W., Chen Z., Choong J., Brodie M.J., Fong S.L., Lim K.S., Wu J., Wang X., Lawn N., Ni G., Gao X., Luo M., Chen Z., Ge Z., Kwan P. Development and validation of a deep learning model for predicting treatment response in patients with newly diagnosed epilepsy. JAMA Neurol 2022; 79(10): 986–996, https://doi.org/10.1001/jamaneurol.2022.2514.
- Plata A., Lebedeva A., Denisov P., Nosova O., Postnikova T.Y., Pimashkin A., Brazhe A., Zaitsev A.V., Rusakov D.A., Semyanov A. Astrocytic atrophy following status epilepticus parallels reduced Ca2+ activity and impaired synaptic plasticity in the rat hippocampus. Front Mol Neurosci 2018; 11: 215, https://doi.org/10.3389/fnmol.2018.00215.
- Lundt A., Wormuth C., Siwek M.E., Müller R., Ehninger D., Henseler C., Broich K., Papazoglou A., Weiergräber M. EEG radiotelemetry in small laboratory rodents: a powerful state-of-the art approach in neuropsychiatric, neurodegenerative, and epilepsy research. Neural Plast 2016; 2016: 8213878, https://doi.org/10.1155/2016/8213878.
- Wei L., Boutouil H., Gerbatin R.R., Mamad O., Heiland M., Reschke C.R., Del Gallo F., Fabene P.F., Henshall D.C., Lowery M., Morris G., Mooney C. Detection of spontaneous seizures in EEGs in multiple experimental mouse models of epilepsy. J Neural Eng 2021; 18(5): 056060, https://doi.org/10.1088/1741-2552/ac2ca0.
- Vishwanath M., Jafarlou S., Shin I., Lim M.M., Dutt N., Rahmani A.M., Cao H. Investigation of machine learning approaches for traumatic brain injury classification via EEG assessment in mice. Sensors (Basel) 2020; 20(7): 2027, https://doi.org/10.3390/s20072027.
- Ahmad I., Wang X., Zhu M., Wang C., Pi Y., Khan J.A., Khan S., Samuel O.W., Chen S., Li G. EEG-based epileptic seizure detection via machine/deep learning approaches: a systematic review. Comput Intell Neurosci 2022; 2022: 6486570, https://doi.org/10.1155/2022/6486570.
- Rosas-Romero R., Guevara E., Peng K., Nguyen D.K., Lesage F., Pouliot P., Lima-Saad W.E. Prediction of epileptic seizures with convolutional neural networks and functional near-infrared spectroscopy signals. Comput Biol Med 2019; 111: 103355, https://doi.org/10.1016/j.compbiomed.2019.103355.
- Wu X., Zhang T., Zhang L., Qiao L. Epileptic seizure prediction using successive variational mode decomposition and transformers deep learning network. Front Neurosci 2022; 16: 982541, https://doi.org/10.3389/fnins.2022.982541.
- Li G., Lee C.H., Jung J.J., Youn Y.C., Camacho D. Deep learning for EEG data analytics: a survey. Concurr Comput Pract Exp 2020; 32(18): e5199, https://doi.org/10.1002/cpe.5199.
- Budde B., Maksimenko V., Sarink K., Seidenbecher T., van Luijtelaar G., Hahn T., Pape H.C., Lüttjohann A. Seizure prediction in genetic rat models of absence epilepsy: improved performance through multiple-site cortico-thalamic recordings combined with machine learning. eNeuro 2022; 9(1): ENEURO.0160-21.2021, https://doi.org/10.1523/eneuro.0160-21.2021.
- Camuñas-Mesa L.A., Linares-Barranco B., Serrano-Gotarredona T. Neuromorphic spiking neural networks and their memristor–CMOS hardware implementations. Materials (Basel) 2019; 12(17): 2745, https://doi.org/10.3390/ma12172745.
- Fouda M.E., Kurdahi F., Eltawil A., Neftci E. Spiking neural networks for inference and learning: a memristor-based design perspective. arXiv; 2019, https://doi.org/10.48550/arxiv.1909.01771.
- Bayat F.M., Prezioso M., Chakrabarti B., Nili H., Kataeva I., Strukov D. Implementation of multilayer perceptron network with highly uniform passive memristive crossbar circuits. Nat Commun 2018; 9(1): 2331, https://doi.org/10.1038/s41467-018-04482-4.
- Li C., Belkin D., Li Y., Yan P., Hu M., Ge N., Jiang H., Montgomery E., Lin P., Wang Z., Song W., Strachan J.P., Barnell M., Wu Q., Williams R.S., Yang J.J., Xia Q. Efficient and self-adaptive in-situ learning in multilayer memristor neural networks. Nat Commun 2018; 9(1): 2385, https://doi.org/10.1038/s41467-018-04484-2.
- Zhang Y., Wang X., Friedman E.G. Memristor-based circuit design for multilayer neural networks. IEEE Trans Circuits Syst I Reg Papers 2017; 65(2): 677–686, https://doi.org/10.1109/tcsi.2017.2729787.
- Hu S.G., Liu Y., Liu Z., Chen T.P., Wang J.J., Yu Q., Deng L.J., Yin Y., Hosaka S. Associative memory realized by a reconfigurable memristive Hopfield neural network. Nat Commun 2015; 6: 7522, https://doi.org/10.1038/ncomms8522.
- Zhang S., Zheng J., Wang X., Zeng Z., He S. Initial offset boosting coexisting attractors in memristive multi-double-scroll Hopfield neural network. Nonlinear Dyn 2020; 102(4): 2821–2841.
- Yakopcic C., Alom M.Z., Taha T.M. Memristor crossbar deep network implementation based on a convolutional neural network. In: 2016 International Joint Conference on Neural Networks (IJCNN). IEEE; 2016; p. 963–970, https://doi.org/10.1109/ijcnn.2016.7727302.
- Yakopcic C., Alom M.Z., Taha T.M. Extremely parallel memristor crossbar architecture for convolutional neural network implementation. In: 2017 International Joint Conference on Neural Networks (IJCNN). IEEE; 2017; р. 1696–1703, https://doi.org/10.1109/ijcnn.2017.7966055.
- Xu W., Wang J., Yan X. Advances in memristor-based neural networks. Front Nanotechnol 2021; 3: 645995, https://doi.org/10.3389/fnano.2021.645995.
- Gromov N., Gubina E., Levanova T. Loss functions in the prediction of extreme events and chaotic dynamics using machine learning approach. In: 2022 Fourth International Conference Neurotechnologies and Neurointerfaces (CNN). IEEE; 2022, https://doi.org/10.1109/cnn56452.2022.9912515.
- Gerasimova S.A., Mikhaylov A.N., Belov A.I., Korolev D.S., Guseinov D.V., Lebedeva A.V., Gorshkov O.N., Kazantsev V.B. Design of memristive interface between electronic neurons. AIP Conf Proc 2018; 1959(1): 090005, https://doi.org/10.1063/1.5034744.
- Kipelkin I., Gerasimova S., Guseinov D., Pavlov D., Vorontsov V., Mikhaylov A., Kazantsev V. Mathematical and experimental model of neuronal oscillator based on memristor-based nonlinearity. Mathematics 2023; 11(5): 1268, https://doi.org/10.3390/math11051268.
- Li C., Wang Z., Rao M., Belkin D., Song W., Jiang H., Yan P., Li Y., Lin P., Hu M., Ge N., Strachan J.P., Barnell M., Wu Q., Williams R.S., Yang J.J., Xia Q. Long short-term memory networks in memristor crossbar arrays. Nature Mach Intell 2019; 1: 49–57.
- Mikhaylov A.N., Belov A.I., Korolev D.S., Gerasimova S.A., Antonov I.N., Okulich E.V., Shuiskiy R.A., Tetelbaum D.I. Effect of ion irradiation on resistive switching in metal-oxide memristive nanostructures. J Phys Conf Ser 2019; 1410(1): 012245, https://doi.org/10.1088/1742-6596/1410/1/012245.
- van Luijtelaar G., Lüttjohann A., Makarov V.V., Maksimenko V.A., Koronovskii A.A., Hramov A.E. Methods of automated absence seizure detection, interference by stimulation, and possibilities for prediction in genetic absence models. J Neurosci Methods 2016; 260: 144–158, https://doi.org/10.1016/j.jneumeth.2015.07.010.
- Li X., Ouyang G., Richards D.A. Predictability analysis of absence seizures with permutation entropy. Epilepsy Res 2007; 77(1): 70–74, https://doi.org/10.1016/j.eplepsyres.2007.08.002.
- Pisarchik A.N., Grubov V.V., Maksimenko V.A., Lüttjohann A., Frolov N.S., Marqués-Pascual C., Gonzalez-Nieto D., Khramova M.V., Hramov A.E. Extreme events in epileptic EEG of rodents after ischemic stroke. Eur Phys J Spec Top 2018; 227: 921–932.
- Frolov N.S., Grubov V.V., Maksimenko V.A., Lüttjohann A., Makarov V.V., Pavlov A.N., Sitnikova E., Pisarchik A.N., Kurths J., Hramov A.E. Statistical properties and predictability of extreme epileptic events. Sci Rep 2019; 9(1): 7243, https://doi.org/10.1038/s41598-019-43619-3.