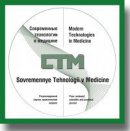
Brain–Computer Interfaces for Upper Limb Motor Recovery after Stroke: Current Status and Development Prospects (Review)
Brain–computer interfaces (BCIs) are a group of technologies that allow mental training with feedback for post-stroke motor recovery. Varieties of these technologies have been studied in numerous clinical trials for more than 10 years, and their construct and software are constantly being improved. Despite the positive treatment results and the availability of registered medical devices, there are currently a number of problems for the wide clinical application of BCI technologies. This review provides information on the most studied types of BCIs and its training protocols and describes the evidence base for the effectiveness of BCIs for upper limb motor recovery after stroke. The main problems of scaling this technology and ways to solve them are also described.
- Khan M.A., Das R., Iversen H.K., Puthusserypady S. Review on motor imagery based BCI systems for upper limb post-stroke neurorehabilitation: from designing to application. Comput Biol Med 2020; 123: 103843, https://doi.org/10.1016/j.compbiomed.2020.103843.
- Khrulev A.E., Kuryatnikova K.M., Belova A.N., Popova P.S., Khrulev S.E. Modern rehabilitation technologies of patients with motor disorders at an early rehabilitation of stroke (review). Sovremennye tehnologii v medicine 2022; 14(6): 64, https://doi.org/10.17691/stm2022.14.6.07.
- Molinari M., Masciullo M. Stroke and potential benefits of brain-computer interface. Handb Clin Neurol 2020; 168: 25–32, https://doi.org/10.1016/b978-0-444-63934-9.00003-2.
- Mokienko O.A., Chernikova L.A., Frolov A.A., Bobrov P.D. Motor imagery and its practical application. Neurosci Behav Physi 2014; 44: 483–489, https://doi.org/10.1007/s11055-014-9937-y.
- Mokienko O.A., Chervyakov A.V., Kulikova S.N., Bobrov P.D., Chernikova L.A., Frolov A.A., Piradov M.A. Increased motor cortex excitability during motor imagery in brain-computer interface trained subjects. Front Comput Neurosci 2013; 7: 168, https://doi.org/10.3389/fncom.2013.00168.
- Girges C., Vijiaratnam N., Zrinzo L., Ekanayake J., Foltynie T. Volitional control of brain motor activity and its therapeutic potential. Neuromodulation 2022; 25(8): 1187–1196, https://doi.org/10.1016/j.neurom.2022.01.007.
- Bhagat N.A., Yozbatiran N., Sullivan J.L., Paranjape R., Losey C., Hernandez Z., Keser Z., Grossman R., Francisco G.E., O’Malley M.K., Contreras-Vidal J.L. Neural activity modulations and motor recovery following brain-exoskeleton interface mediated stroke rehabilitation. Neuroimage Clin 2020; 28: 102502, https://doi.org/10.1016/j.nicl.2020.102502.
- Yuan K., Chen C., Wang X., Chu W.C.W., Tong R.K.Y. BCI training effects on chronic stroke correlate with functional reorganization in motor-related regions: a concurrent EEG and fMRI study. Brain Sci 2021; 11(1): 56, https://doi.org/10.3390/brainsci11010056.
- Liao W., Li J., Zhang X., Li C. Motor imagery brain-computer interface rehabilitation system enhances upper limb performance and improves brain activity in stroke patients: a clinical study. Front Hum Neurosci 2023; 17: 1117670, https://doi.org/10.3389/fnhum.2023.1117670.
- Liu M., Ushiba J. Brain-machine interface (BMI)-based neurorehabilitation for post-stroke upper limb paralysis. Keio J Med 2022; 71(4): 82–92, https://doi.org/10.2302/kjm.2022-0002-oa.
- Carvalho R., Dias N., Cerqueira J.J. Brain-machine interface of upper limb recovery in stroke patients rehabilitation: a systematic review. Physiother Res Int 2019; 24(2): e1764, https://doi.org/10.1002/pri.1764.
- Baniqued P.D.E., Stanyer E.C., Awais M., Alazmani A., Jackson A.E., Mon-Williams M.A., Mushtaq F., Holt R.J. Brain-computer interface robotics for hand rehabilitation after stroke: a systematic review. J Neuroeng Rehabil 2021; 18(1): 15, https://doi.org/10.1186/s12984-021-00820-8.
- Fu J., Chen S., Jia J. Sensorimotor rhythm-based brain-computer interfaces for motor tasks used in hand upper extremity rehabilitation after stroke: a systematic review. Brain Sci 2022; 13(1): 56, https://doi.org/10.3390/brainsci13010056.
- Bai Z., Fong K.N.K., Zhang J.J., Chan J., Ting K.H. Immediate and long-term effects of BCI-based rehabilitation of the upper extremity after stroke: a systematic review and meta-analysis. J Neuroeng Rehabil 2020; 17(1): 57, https://doi.org/10.1186/s12984-020-00686-2.
- Kruse A., Suica Z., Taeymans J., Schuster-Amft C. Effect of brain-computer interface training based on non-invasive electroencephalography using motor imagery on functional recovery after stroke — a systematic review and meta-analysis. BMC Neurol 2020; 20(1): 385, https://doi.org/10.1186/s12883-020-01960-5.
- Yang W., Zhang X., Li Z., Zhang Q., Xue C., Huai Y. The effect of brain-computer interface training on rehabilitation of upper limb dysfunction after stroke: a meta-analysis of randomized controlled trials. Front Neurosci 2021; 15: 766879, https://doi.org/10.3389/fnins.2021.766879.
- Mansour S., Ang K.K., Nair K.P.S., Phua K.S., Arvaneh M. Efficacy of brain-computer interface and the impact of its design characteristics on poststroke upper-limb rehabilitation: a systematic review and meta-analysis of randomized controlled trials. Clin EEG Neurosci 2022; 53(1): 79–90, https://doi.org/10.1177/15500594211009065.
- Peng Y., Wang J., Liu Z., Zhong L., Wen X., Wang P., Gong X., Liu H. The application of brain-computer interface in upper limb dysfunction after stroke: a systematic review and meta-analysis of randomized controlled trials. Front Hum Neurosci 2022; 16: 798883, https://doi.org/10.3389/fnhum.2022.798883.
- Nojima I., Sugata H., Takeuchi H., Mima T. Brain-computer interface training based on brain activity can induce motor recovery in patients with stroke: a meta-analysis. Neurorehabil Neural Repair 2022; 36(2): 83–96, https://doi.org/10.1177/15459683211062895.
- Xie Y.L., Yang Y.X., Jiang H., Duan X.Y., Gu L.J., Qing W., Zhang B., Wang Y.X. Brain-machine interface-based training for improving upper extremity function after stroke: a meta-analysis of randomized controlled trials. Front Neurosci 2022; 16: 949575, https://doi.org/10.3389/fnins.2022.949575.
- Shou Y.Z., Wang X.H., Yang G.F. Verum versus Sham brain-computer interface on upper limb function recovery after stroke: a systematic review and meta-analysis of randomized controlled trials. Medicine (Baltimore) 2023; 102(26): e34148, https://doi.org/10.1097/md.0000000000034148.
- IpsiHand rehab device for stroke survivors. Neurolutions; 2023. URL: https://www.neurolutions.com/ipsihand.
- Bundy D.T., Souders L., Baranyai K., Leonard L., Schalk G., Coker R., Moran D.W., Huskey T., Leuthardt E.C. Contralesional brain–computer interface control of a powered exoskeleton for motor recovery in chronic stroke survivors. Stroke 2017; 48(7): 1908–1915, https://doi.org/10.1161/strokeaha.116.016304.
- Ekzokist’-2 [Exo-hand-2]. Ekzoplast; 2023. URL: https://exoplast.ru/.
- Ortez-1. Androidnaya tekhnika; 2023. URL: https://npo-at.com/production/orthosis/.
- Mokienko O.A., Lyukmanov R.Kh., Chernikova L.A., Suponeva N.A., Piradov M.A., Frolov A.A. Brain-computer interface: the first clinical experience in Russia. Hum Physiol 2016; 42(1): 24–31, https://doi.org/10.1134/s0362119716010126.
- Lyukmanov R.Kh., Aziatskaya G.A., Mokienko O.A., Varako N.A., Kovyazina M.S., Suponeva N.A., Chernikova L.A., Frolov A.A., Piradov M.A. Post-stroke rehabilitation training with a brain–computer interface: a clinical and neuropsychological study. Zhurnal nevrologii i psihiatrii im. S.S. Korsakova 2018; 118(8): 43–51, https://doi.org/10.17116/jnevro201811808143.
- Frolov A.A., Mokienko O., Lyukmanov R., Biryukova E., Kotov S., Turbina L., Nadareyshvily G., Bushkova Y. Post-stroke rehabilitation training with a motor-imagery-based brain-computer interface (BCI)-controlled hand exoskeleton: a randomized controlled multicenter trial. Front Neurosci 2017; 11: 400, https://doi.org/10.3389/fnins.2017.00400.
- Frolov A.A., Bobrov P.D., Biryukova E.V., Silchenko A.V., Kondur A.A., Dzhalagoniya I.Z., Massion J. Electrical, hemodynamic, and motor activity in BCI post-stroke rehabilitation: clinical case study. Front Neurol 2018; 9: 1135, https://doi.org/10.3389/fneur.2018.01135.
- Zhang R., Wang C., He S., Zhao C., Zhang K., Wang X., Li Y. An adaptive brain-computer interface to enhance motor recovery after stroke. IEEE Trans Neural Syst Rehabil Eng 2023; 31: 2268–2278, https://doi.org/10.1109/tnsre.2023.3272372.
- Fu J., Chen S., Shu X., Lin Y., Jiang Z., Wei D., Gao J., Jia J. Functional-oriented, portable brain-computer interface training for hand motor recovery after stroke: a randomized controlled study. Front Neurosci 2023; 17: 1146146, https://doi.org/10.3389/fnins.2023.1146146.
- Craik A., González-España J.J., Alamir A., Edquilang D., Wong S., Sánchez Rodríguez L., Feng J., Francisco G.E., Contreras-Vidal J.L. Design and validation of a low-cost mobile EEG-based brain-computer interface. Sensors (Basel) 2023; 23(13): 5930, https://doi.org/10.3390/s23135930.
- Gao Z., Pang Z., Chen Y., Lei G., Zhu S., Li G., Shen Y., Xu W. Restoring after central nervous system injuries: neural mechanisms and translational applications of motor recovery. Neurosci Bull 2022; 38(12): 1569–1587, https://doi.org/10.1007/s12264-022-00959-x.
- Behboodi A., Lee W.A., Hinchberger V.S., Damiano D.L. Determining optimal mobile neurofeedback methods for motor neurorehabilitation in children and adults with non-progressive neurological disorders: a scoping review. J Neuroeng Rehabil 2022; 19(1): 104, https://doi.org/10.1186/s12984-022-01081-9.
- Fu J., Jiang Z., Shu X., Chen S., Jia J. Correlation between the ERD in grasp/open tasks of BCIs and hand function of stroke patients: a cross-sectional study. Biomed Eng Online 2023; 22(1): 36, https://doi.org/10.1186/s12938-023-01091-1.
- Zanona A.F., Piscitelli D., Seixas V.M., Scipioni K.R.D.D.S., Bastos M.S.C., de Sá L.C.K., Monte-Silva K., Bolivar M., Solnik S., De Souza R.F. Brain-computer interface combined with mental practice and occupational therapy enhances upper limb motor recovery, activities of daily living, and participation in subacute stroke. Front Neurol 2022; 13: 1041978, https://doi.org/10.3389/fneur.2022.1041978.
- Gao W., Cui Z., Yu Y., Mao J., Xu J., Ji L., Kan X., Shen X., Li X., Zhu S., Hong Y. Application of a brain-computer interface system with visual and motor feedback in limb and brain functional rehabilitation after stroke: case report. Brain Sci 2022; 12(8): 1083, https://doi.org/10.3390/brainsci12081083.
- Ang K.K., Chua K.S.G., Phua K.S., Wang C., Chin Z.Y., Kuah C.W., Low W., Guan C. A randomized controlled trial of EEG-based motor imagery brain-computer interface robotic rehabilitation for stroke. Clin EEG Neurosci 2015; 46(4): 310–320, https://doi.org/10.1177/1550059414522229.
- Ang K.K., Guan C., Phua K.S., Wang C., Zhou L., Tang K.Y., Ephraim Joseph G.J., Kuah C.W.K., Chua K.S.G. Brain-computer interface-based robotic end effector system for wrist and hand rehabilitation: results of a three-armed randomized controlled trial for chronic stroke. Front Neuroeng 2014; 7: 30, https://doi.org/10.3389/fneng.2014.00030.
- Ang K.K., Guan C., Phua K.S., Wang C., Zhao L., Teo W.P., Chen C., Ng Y.S., Chew E. Facilitating effects of transcranial direct current stimulation on motor imagery brain-computer interface with robotic feedback for stroke rehabilitation. Arch Phys Med Rehabil 2015; 96(3 Suppl): S79–S87, https://doi.org/10.1016/j.apmr.2014.08.008.
- Cheng N., Phua K.S., Lai H.S., Tam P.K., Tang K.Y., Cheng K.K., Yeow R.C., Ang K.K., Guan C., Lim J.H. Brain-computer interface-based soft robotic glove rehabilitation for stroke. IEEE Trans Biomed Eng 2020; 67(12): 3339–3351, https://doi.org/10.1109/tbme.2020.2984003.
- Li M., Liu Y., Wu Y., Liu S., Jia J., Zhang L. Neurophysiological substrates of stroke patients with motor imagery-based brain-computer interface training. Int J Neurosci 2014; 124(6): 403–415, https://doi.org/10.3109/00207454.2013.850082.
- Mihara M., Hattori N., Hatakenaka M., Yagura H., Kawano T., Hino T., Miyai I. Near-infrared spectroscopy-mediated neurofeedback enhances efficacy of motor imagery-based training in poststroke victims: a pilot study. Stroke 2013; 44(4): 1091–1098, https://doi.org/10.1161/strokeaha.111.674507.
- Pichiorri F., Morone G., Petti M., Toppi J., Pisotta I., Molinari M., Paolucci S., Inghilleri M., Astolfi L., Cincotti F., Mattia D. Brain-computer interface boosts motor imagery practice during stroke recovery. Ann Neurol 2015; 77(5): 851–865, https://doi.org/10.1002/ana.24390.
- Wu Q., Yue Z., Ge Y., Ma D., Yin H., Zhao H., Liu G., Wang J., Dou W., Pan Y. Brain functional networks study of subacute stroke patients with upper limb dysfunction after comprehensive rehabilitation including BCI training. Front Neurol 2019; 10: 1419, https://doi.org/10.3389/fneur.2019.01419.
- Biasiucci A., Leeb R., Iturrate I., Perdikis S., Al-Khodairy A., Corbet T., Schnider A., Schmidlin T., Zhang H., Bassolino M., Viceic D., Vuadens P., Guggisberg A.G., Millán J.D.R. Brain-actuated functional electrical stimulation elicits lasting arm motor recovery after stroke. Nat Commun 2018; 9(1): 2421, https://doi.org/10.1038/s41467-018-04673-z.
- Ramos-Murguialday A., Broetz D., Rea M., Läer L., Yilmaz O., Brasil F.L., Liberati G., Curado M.R., Garcia-Cossio E., Vyziotis A., Cho W., Agostini M., Soares E., Soekadar S., Caria A., Cohen L.G., Birbaumer N. Brain-machine interface in chronic stroke rehabilitation: a controlled study. Ann Neurol 2013; 74(1): 100–108, https://doi.org/10.1002/ana.23879.
- Chen S., Cao L., Shu X., Wang H., Ding L., Wang S.H., Jia J. Longitudinal electroencephalography analysis in subacute stroke patients during intervention of brain-computer interface with exoskeleton feedback. Front Neurosci 2020; 14: 809, https://doi.org/10.3389/fnins.2020.00809.
- Antelis J.M., Montesano L., Ramos-Murguialday A., Birbaumer N., Minguez J. Decoding upper limb movement attempt from EEG measurements of the contralesional motor cortex in chronic stroke patients. IEEE Trans Biomed Eng 2017; 64(1): 99–111, https://doi.org/10.1109/tbme.2016.2541084.
- Soekadar S.R., Kohl S.H., Mihara M., von Lühmann A. Optical brain imaging and its application to neurofeedback. Neuroimage Clin 2021; 30: 102577, https://doi.org/10.1016/j.nicl.2021.102577.
- Lee Friesen C., Lawrence M., Ingram T.G.J., Boe S.G. Home-based portable fNIRS-derived cortical laterality correlates with impairment and function in chronic stroke. Front Hum Neurosci 2022; 16: 1023246, https://doi.org/10.3389/fnhum.2022.1023246.
- Hramov A.E., Maksimenko V.A., Pisarchik A.N. Physical principles of brain–computer interfaces and their applications for rehabilitation, robotics and control of human brain states. Phys Rep 2021; 918: 1–133, https://doi.org/10.1016/j.physrep.2021.03.002.
- Lotte F., Bougrain L., Cichocki A., Clerc M., Congedo M., Rakotomamonjy A., Yger F. A review of classification algorithms for EEG-based brain-computer interfaces: a 10 year update. J Neural Eng 2018; 15(3): 031005, https://doi.org/10.1088/1741-2552/aab2f2.
- Wang X., Wong W.W., Sun R., Chu W.C.W., Tong K.Y. Differentiated effects of robot hand training with and without neural guidance on neuroplasticity patterns in chronic stroke. Front Neurol 2018; 9: 810, https://doi.org/10.3389/fneur.2018.00810.
- Reynolds C., Osuagwu B.A., Vuckovic A. Influence of motor imagination on cortical activation during functional electrical stimulation. Clin Neurophysiol 2015; 126(7): 1360–1369, https://doi.org/10.1016/j.clinph.2014.10.007.
- Bergquist A.J., Clair J.M., Lagerquist O., Mang C.S., Okuma Y., Collins D.F. Neuromuscular electrical stimulation: implications of the electrically evoked sensory volley. Eur J Appl Physiol 2011; 111(10): 2409–2426, https://doi.org/10.1007/s00421-011-2087-9.
- Chen L., Gu B., Wang Z., Zhang L., Xu M., Liu S., He F., Ming D. EEG-controlled functional electrical stimulation rehabilitation for chronic stroke: system design and clinical application. Front Med 2021; 15(5): 740–749, https://doi.org/10.1007/s11684-020-0794-5.
- Sinha A.M., Nair V.A., Prabhakaran V. Brain-computer interface training with functional electrical stimulation: facilitating changes in interhemispheric functional connectivity and motor outcomes post-stroke. Front Neurosci 2021; 15: 670953, https://doi.org/10.3389/fnins.2021.670953.
- Lee S.H., Kim S.S., Lee B.H. Action observation training and brain-computer interface controlled functional electrical stimulation enhance upper extremity performance and cortical activation in patients with stroke: a randomized controlled trial. Physiother Theory Pract 2022; 38(9): 1126–1134, https://doi.org/10.1080/09593985.2020.1831114.
- Kim T., Kim S., Lee B. Effects of action observational training plus brain-computer interface-based functional electrical stimulation on paretic arm motor recovery in patient with stroke: a randomized controlled trial. Occup Ther Int 2016; 23(1): 39–47, https://doi.org/10.1002/oti.1403.
- Miao Y., Chen S., Zhang X., Jin J., Xu R., Daly I., Jia J., Wang X., Cichocki A., Jung T.P. BCI-based rehabilitation on the stroke in sequela stage. Neural Plast 2020; 2020: 8882764, https://doi.org/10.1155/2020/8882764.
- Simon C., Bolton D.A.E., Kennedy N.C., Soekadar S.R., Ruddy K.L. Challenges and opportunities for the future of brain-computer interface in neurorehabilitation. Front Neurosci 2021; 15: 699428, https://doi.org/10.3389/fnins.2021.699428.
- Colucci A., Vermehren M., Cavallo A., Angerhöfer C., Peekhaus N., Zollo L., Kim W.S., Paik N.J., Soekadar S.R. Brain-computer interface-controlled exoskeletons in clinical neurorehabilitation: ready or not? Neurorehabil Neural Repair 2022; 36(12): 747–756, https://doi.org/10.1177/15459683221138751.
- Mridha M.F., Das S.C., Kabir M.M., Lima A.A., Islam M.R., Watanobe Y. Brain-computer interface: advancement and challenges. Sensors (Basel) 2021; 21(17): 5746, https://doi.org/10.3390/s21175746.
- Jervis-Rademeyer H., Ong K., Djuric A., Munce S., Musselman K.E., Marquez-Chin C. Therapists’ perspectives on using brain-computer interface-triggered functional electrical stimulation therapy for individuals living with upper extremity paralysis: a qualitative case series study. J Neuroeng Rehabil 2022; 19(1): 127, https://doi.org/10.1186/s12984-022-01107-2.
- Padfield N., Zabalza J., Zhao H., Masero V., Ren J. EEG-based brain-computer interfaces using motor-imagery: techniques and challenges. Sensors (Basel) 2019; 19(6): 1423, https://doi.org/10.3390/s19061423.
- Saha S., Mamun K.A., Ahmed K., Mostafa R., Naik G.R., Darvishi S., Khandoker A.H., Baumert M. Progress in brain computer interface: challenges and opportunities. Front Syst Neurosci 2021; 15: 578875, https://doi.org/10.3389/fnsys.2021.578875.
- Angerhöfer C., Colucci A., Vermehren M., Hömberg V., Soekadar S.R. Post-stroke rehabilitation of severe upper limb paresis in Germany — toward long-term treatment with brain-computer interfaces. Front Neurol 2021; 12: 772199, https://doi.org/10.3389/fneur.2021.772199.
- Bobrova E.V., Reshetnikova V.V., Vershinina E.A., Grishin A.A., Bobrov P.D., Frolov A.A., Gerasimenko Y.P. Success of hand movement imagination depends on personality traits, brain asymmetry, and degree of handedness. Brain Sci 2021; 11(7): 853, https://doi.org/10.3390/brainsci11070853.
- Sebastián-Romagosa M., Cho W., Ortner R., Murovec N., Von Oertzen T., Kamada K., Allison B.Z., Guger C. Brain computer interface treatment for motor rehabilitation of upper extremity of stroke patients — a feasibility study. Front Neurosci 2020; 14: 591435, https://doi.org/10.3389/fnins.2020.591435.
- Thompson M.C. Critiquing the concept of BCI illiteracy. Sci Eng Ethics 2019; 25(4): 1217–1233, https://doi.org/10.1007/s11948-018-0061-1.
- Wang T., Du S., Dong E. A novel method to reduce the motor imagery BCI illiteracy. Med Biol Eng Comput 2021; 59(11–12): 2205–2217, https://doi.org/10.1007/s11517-021-02449-0.
- Mansour S., Giles J., Ang K.K., Nair K.P.S., Phua K.S., Arvaneh M. Exploring the ability of stroke survivors in using the contralesional hemisphere to control a brain-computer interface. Sci Rep 2022; 12(1): 16223, https://doi.org/10.1038/s41598-022-20345-x.
- Chen S., Shu X., Jia J., Wang H., Ding L., He Z., Brauer S., Zhu X. Relation between sensorimotor rhythm during motor attempt/imagery and upper-limb motor impairment in stroke. Clin EEG Neurosci 2022; 53(3): 238–247, https://doi.org/10.1177/15500594211019917.
- Leamy D.J., Collins R., Ward T.E. Combining fNIRS and EEG to improve motor cortex activity classification during an imagined movement-based task. In: Foundations of augmented cognition. Directing the future of adaptive systems. Schmorrow D.D., Fidopiastis C.M. (editors). Springer Berlin Heidelberg; 2011; p. 177–185.
- Fazli S., Mehnert J., Steinbrink J., Curio G., Villringer A., Müller K.R., Blankertz B. Enhanced performance by a hybrid NIRS–EEG brain computer interface. Neuroimage 2012; 59(1): 519–529, https://doi.org/10.1016/j.neuroimage.2011.07.084.
- Liang W.D., Xu Y., Schmidt J., Zhang L.X., Ruddy K.L. Upregulating excitability of corticospinal pathways in stroke patients using TMS neurofeedback; a pilot study. Neuroimage Clin 2020; 28: 102465, https://doi.org/10.1016/j.nicl.2020.102465.
- de Seta V., Toppi J., Colamarino E., Molle R., Castellani F., Cincotti F., Mattia D., Pichiorri F. Cortico-muscular coupling to control a hybrid brain-computer interface for upper limb motor rehabilitation: a pseudo-online study on stroke patients. Front Hum Neurosci 2022; 16: 1016862, https://doi.org/10.3389/fnhum.2022.1016862.
- Al-Qazzaz N.K., Aldoori A.A., Ali S.H.B.M., Ahmad S.A., Mohammed A.K., Mohyee M.I. EEG signal complexity measurements to enhance BCI-based stroke patients’ rehabilitation. Sensors (Basel) 2023; 23(8): 3889, https://doi.org/10.3390/s23083889.
- Zhang R., Chen Y., Xu Z., Zhang L., Hu Y., Chen M. Recognition of single upper limb motor imagery tasks from EEG using multi-branch fusion convolutional neural network. Front Neurosci 2023; 17: 1129049, https://doi.org/10.3389/fnins.2023.1129049.
- Delisle-Rodriguez D., Silva L., Bastos-Filho T. EEG changes during passive movements improve the motor imagery feature extraction in BCIs-based sensory feedback calibration. J Neural Eng 2023; 20(1): 016047, https://doi.org/10.1088/1741-2552/acb73b.
- Wu D., Jiang X., Peng R. Transfer learning for motor imagery based brain-computer interfaces: a tutorial. Neural Netw 2022; 153: 235–253, https://doi.org/10.1016/j.neunet.2022.06.008.
- Varsehi H., Firoozabadi S.M.P. An EEG channel selection method for motor imagery based brain-computer interface and neurofeedback using Granger causality. Neural Netw 2021; 133: 193–206, https://doi.org/10.1016/j.neunet.2020.11.002.
- Al-Qazzaz N.K., Alyasseri Z.A.A., Abdulkareem K.H., Ali N.S., Al-Mhiqani M.N., Guger C. EEG feature fusion for motor imagery: a new robust framework towards stroke patients rehabilitation. Comput Biol Med 2021; 137: 104799, https://doi.org/10.1016/j.compbiomed.2021.104799.
- Zhang X., Ma Z., Zheng H., Li T., Chen K., Wang X., Liu C., Xu L., Wu X., Lin D., Lin H. The combination of brain-computer interfaces and artificial intelligence: applications and challenges. Ann Transl Med 2020; 8(11): 712, https://doi.org/10.21037/atm.2019.11.109.
- Cao L., Wu H., Chen S., Dong Y., Zhu C., Jia J., Fan C. A novel deep learning method based on an overlapping time window strategy for brain-computer interface-based stroke rehabilitation. Brain Sci 2022; 12(11): 1502, https://doi.org/10.3390/brainsci12111502.
- Hossain K.M., Islam M.A., Hossain S., Nijholt A., Ahad M.A.R. Status of deep learning for EEG-based brain-computer interface applications. Front Comput Neurosci 2022; 16: 1006763, https://doi.org/10.3389/fncom.2022.1006763.
- Sollfrank T., Ramsay A., Perdikis S., Williamson J., Murray-Smith R., Leeb R., Millán J.D.R., Kübler A. The effect of multimodal and enriched feedback on SMR-BCI performance. Clin Neurophysiol 2016; 127(1): 490–498, https://doi.org/10.1016/j.clinph.2015.06.004.
- Ma Z.Z., Wu J.J., Hua X.Y., Zheng M.X., Xing X.X., Ma J., Shan C.L., Xu J.G. Evidence of neuroplasticity with brain-computer interface in a randomized trial for post-stroke rehabilitation: a graph-theoretic study of subnetwork analysis. Front Neurol 2023; 14: 1135466, https://doi.org/10.3389/fneur.2023.1135466.
- Chen S., Shu X., Wang H., Ding L., Fu J., Jia J. The differences between motor attempt and motor imagery in brain-computer interface accuracy and event-related desynchronization of patients with hemiplegia. Front Neurorobot 2021; 15: 706630, https://doi.org/10.3389/fnbot.2021.706630.
- Alghamdi I., Ariti C., Williams A., Wood E., Hewitt J. Prevalence of fatigue after stroke: a systematic review and meta-analysis. Eur Stroke J 2021; 6(4): 319–332, https://doi.org/10.1177/23969873211047681.
- de Castro-Cros M., Sebastian-Romagosa M., Rodríguez-Serrano J., Opisso E., Ochoa M., Ortner R., Guger C., Tost D. Effects of gamification in BCI functional rehabilitation. Front Neurosci 2020; 14: 882, https://doi.org/10.3389/fnins.2020.00882.
- Ziadeh H., Gulyas D., Nielsen LD., Lehmann S., Nielsen T.B., Kjeldsen T.K.K., Hougaard B.I., Jochumsen M., Knoche H. “Mine works better”: examining the influence of embodiment in virtual reality on the sense of agency during a binary motor imagery task with a brain-computer interface. Front Psychol 2021; 12: 806424, https://doi.org/10.3389/fpsyg.2021.806424.
- Zulauf-Czaja A., Al-Taleb M.K.H., Purcell M., Petric-Gray N., Cloughley J., Vuckovic A. On the way home: a BCI-FES hand therapy self-managed by sub-acute SCI participants and their caregivers: a usability study. J Neuroeng Rehabil 2021; 18(1): 44, https://doi.org/10.1186/s12984-021-00838-y.
- McWilliams E.C., Barbey F.M., Dyer J.F., Islam M.N., McGuinness B., Murphy B., Nolan H., Passmore P., Rueda-Delgado L.M., Buick A.R. Feasibility of repeated assessment of cognitive function in older adults using a wireless, mobile, dry-EEG headset and tablet-based games. Front Psychiatry 2021; 12: 574482, https://doi.org/10.3389/fpsyt.2021.574482.
- Jamil N., Belkacem A.N., Ouhbi S., Lakas A. Noninvasive electroencephalography equipment for assistive, adaptive, and rehabilitative brain-computer interfaces: a systematic literature review. Sensors (Basel) 2021; 21(14): 4754, https://doi.org/10.3390/s21144754.
- Yang S., Li R., Li H., Xu K., Shi Y., Wang Q., Yang T., Sun X. Exploring the use of brain-computer interfaces in stroke neurorehabilitation. BioMed Res Int 2021; 2021: 9967348, https://doi.org/10.1155/2021/9967348.
- Hashimoto Y., Kakui T., Ushiba J., Liu M., Kamada K., Ota T. Portable rehabilitation system with brain-computer interface for inpatients with acute and subacute stroke: a feasibility study. Assist Technol 2022; 34(4): 402–410, https://doi.org/10.1080/10400435.2020.1836067.
- Borisova V.A., Isakova E.V., Kotov S.V. Possibilities of the brain–computer interface in the correction of post-stroke cognitive impairments. Zhurnal nevrologii i psihiatrii im. S.S. Korsakova 2022; 122(12-2): 60–66, https://doi.org/10.17116/jnevro202212212260.
- Liu X., Zhang W., Li W., Zhang S., Lv P., Yin Y. Effects of motor imagery based brain-computer interface on upper limb function and attention in stroke patients with hemiplegia: a randomized controlled trial. BMC Neurol 2023; 23(1): 136, https://doi.org/10.1186/s12883-023-03150-5.