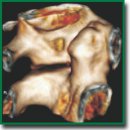
Experience of Using Neural Networks to Assess Age-Related Changes in Some Structures of the Skull and Cervical Vertebrae Based on СТ Scans (Pilot Project)
The aim of the investigation is to study the possibility of using artificial intelligence technologies for age prediction based on CT studies of some structures of the skull and cervical vertebrae.
Material and Methods. The study included 223 tomograms of the head and neck in sagittal projection from patients without any pathology of the studied structures. Morphometric analysis was carried out using PjaPro and Gradient programs, statistical analysis was performed by SPSS Statistics software. A fully convolutional EfficientNet-B2 neural network was used, which was trained in two stages: selection of the area of interest and solution of regression tasks.
Results. Morphometric assessment and subsequent statistical analysis of the selected group of features have shown presence of the strongest correlation with age in the indicator characterizing the involution of the median atlantoaxial joint. A deep learning method using the convolutional network, which automatically selects the desired area in the image (the area of the vertebral junction), classifies the sample, and makes an assumption about the age of the unknown individual with an accuracy of 7.5 to 10.5 years has been tested.
Conclusion. As a result of the study, a positive experience has been obtained indicating the possibility of using convolutional neural networks to determine the age of the unknown person, which expands the evidence base and provides new opportunities for determining group-wide personality traits in forensic medicine.
- Loganathan T., Chan Z.X., Hassan F., Ong Z.L., Majid H.A. Undocumented: an examination of legal identity and education provision for children in Malaysia. PLoS One 2022; 17(2): e0263404, https://doi.org/10.1371/journal.pone.0263404.
- Zolotenkova G.V., Rogachev A.I., Pigolkin Y.I., Edelev I.S., Borshchevskaya V.N., Cameriere R. Age сlassification in forensic medicine using machine learning techniques. Sovremennye tehnologii v medicine 2022; 14(1): 15, https://doi.org/10.17691/stm2022.14.1.02.
- Knudtzen F.C., Mørk L., Nielsen V.N., Astrup B.S. Accessing vulnerable undocumented migrants through a healthcare clinic including a community outreach programme: a 12-year retrospective cohort study in Denmark. J Travel Med 2022; 29(7): taab128, https://doi.org/10.1093/jtm/taab128.
- International Commission of Jurists. Migration and International Human Rights Law. A practitioners’ guide. Updated edition, 2014. URL: https://www.icj.org/wp-content/uploads/2014/10/Universal-MigrationHRlaw-PG-no-6-Publications-PractitionersGuide-2014-eng.pdf.
- Cummaudo M., De Angelis D., Magli F., Minà G., Merelli V., Cattaneo C. Age estimation in the living: a scoping review of population data for skeletal and dental methods. Forensic Sci Int 2021; 320: 110689, https://doi.org/10.1016/j.forsciint.2021.110689.
- Priya E. Methods of skeletal age estimation used by forensic anthropologists in adults: a review. Forensic Res Criminol Int J 2017; 4(2): 41–51, https://doi.org/10.15406/frcij.2017.04.00104.
- Bailey C., Vidoli G. Age-at-death estimation: accuracy and reliability of common age-reporting strategies in forensic anthropology. Forensic Sci 2023; 3(1): 179–191, https://doi.org/10.3390/forensicsci3010014.
- Zaborowicz M., Zaborowicz K., Biedziak B., Garbowski T. Deep learning neural modelling as a precise method in the assessment of the chronological age of children and adolescents using tooth and bone parameters. Sensors (Basel) 2022; 22(2): 637, https://doi.org/10.3390/s22020637.
- Gridina N.V., Zolotenkova G.V., Rogachev A.I., Pigolkin Yu.I. Building a 3D model of heart vessels with the removal of calcifications. Sistemy vysokoj dostupnosti 2020; 16(4): 64–70, https://doi.org/10.18127/j20729472-202004-06.
- Chetverikov S.F., Arzamasov K.M., Andreichenko A.E., Novik V.P., Bobrovskaya T.M., Vladzimirsky A.V. Approaches to sampling for quality control of artificial intelligence in biomedical research. Sovremennye tehnologii v medicine 2023; 15(2): 19, https://doi.org/10.17691/stm2023.15.2.02.
- LeCun Y., Bengio Y., Hinton G. Deep learning. Nature 2015; 521(7553): 436–444, https://doi.org/10.1038/nature14539.
- Dallora A.L., Anderberg P., Kvist O., Mendes E., Diaz Ruiz S., Sanmartin Berglund J. Bone age assessment with various machine learning techniques: a systematic literature review and meta-analysis. PLoS One 2019; 14(7): e0220242, https://doi.org/10.1371/journal.pone.0220242.
- Anthimopoulos M., Christodoulidis S., Ebner L., Christe A., Mougiakakou S. Lung pattern classification for interstitial lung diseases using a deep convolutional neural network. IEEE Trans Med Imaging 2016; 35(5): 1207–1216, https://doi.org/10.1109/tmi.2016.2535865.
- Gogoberidze Y.T., Klassen V.I., Natenzon M.Y., Prosvirkin I.A., Vladzimirsky A.V., Sharova D.E., Zinchenko V.V. PhthisisBioMed artificial medical intelligence: software for automated analysis of digital chest X-ray/fluorograms. Sovremennye tehnologii v medicine 2023; 15(4): 5, https://doi.org/10.17691/stm2023.15.4.01.
- Liskowski P., Krawiec K. Segmenting retinal blood vessels with deep neural networks. IEEE Trans Med Imaging 2016; 35(11): 2369–2380, https://doi.org/10.1109/tmi.2016.2546227.
- Frolov А.А., Pochinka I.G., Shakhov B.Е., Mukhin А.S., Frolov I.А., Barinova М.K., Sharabrin Е.G. Using an artificial neural network to predict coronary microvascular obstruction (no-reflow phenomenon) during percutaneous coronary interventions in patients with myocardial infarction. Sovremennye tehnologii v medicine 2021; 13(6): 6, https://doi.org/10.17691/stm2021.13.6.01.
- Li S., Liu B., Li S., Zhu X., Yan Y., Zhang D. A deep learning-based computer-aided diagnosis method of X-ray images for bone age assessment. Complex Intell Systems 2022; 8(3): 1929–1939, https://doi.org/10.1007/s40747-021-00376-z.
- Greenspan H., Hayit G., van Ginneken B., Summers R.M. Guest editorial deep learning in medical imaging: overview and future promise of an exciting new technique. IEEE Trans Med Imaging 2016; 35(5): 1153–1159, https://doi.org/10.1109/tmi.2016.2553401.
- Huang G., Liu Z., Van Der Maaten L., Weinberger K.Q. Densely connected convolutional networks. In: Proceedings of the IEEE Conference on Computer Vision and Pattern Recognition. Honolulu, HI; 2017; p. 4700–4708, https://doi.org/10.1109/cvpr.2017.243.
- Tan M., Le Q.V. EfficientNet: rethinking model scaling for convolutional neural networks. In: Proceedings of the 36th International Conference on Machine Learning. ICML; 2019; p. 6105–6114, http://proceedings.mlr.press/v97/tan19a.html.
- Spisok programm po analizu izobrazhenij s sajta 1mgmu.com [List of image analysis programs from the site 1mgmu.com]. URL: http://1mgmu.com/nau/spisokizo.html.
- Kovalev A.V., Ametrin M.D., Zolotenkova G.V., Gerasimov A.N., Gornostaev D.V., Poletaeva M.P. Forensic medical determination of the age based on the analysis of CT-scanograms of the skull and the craniovertebral region in the sagittal projection. Sudebno-meditsinskaya ekspertiza 2018; 61(1): 21–27, https://doi.org/10.17116/sudmed201861121-27.