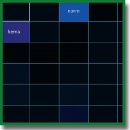
Radiomics and Quantitative MDA Criteria in Breast Cancer with Bone Metastases by MRI: Examples of Calculation Algorithms and Their Practical Use
In the practical work of a radiologist or oncologist, especially in the context of individualized treatment, there is a need for fast and in-depth diagnostics. Radiomics and artificial intelligence can be of great help here. Quantitative and qualitative assessments obtained in this way act as decision support for the physician.
The aim of the study is to enhance the ability of quantitative and qualitative assessment of metastatic spinal lesions in breast cancer, to better evaluate the nature of changes under the influence of therapy, in addition to MDA.
Materials and Methods. We used MRI data in sagittal projection for a patient diagnosed with T2N3M1 breast cancer when treated according to accepted clinical protocols. Metastases to the spine were assessed by a radiologist and by machine analysis using the described software: image internal structure extraction operators and recognition based on traditional neural networks. Fragments of the program codes used are also given.
Results. The structure of metastatically changed vertebrae in sagittal projection was analysed using machine operators of image analysis. Subtle changes in structure such as several types of “calderas” and the pattern of change in image complexity as treatment with CDK 4/6 inhibitors were detected. Measurements were supported by metastasis recognition using neural networks, to increase the reliability of the estimates. In addition to the ability to record response to therapy, a fundamental ability to assess the degree of action compared to previous therapy was identified.
Conclusion. The study showed high efficiency of using image structure analysis algorithms, good correlation of the results obtained with the radiologist’s opinion and with clinical and laboratory data, and allowed to approach the analysis of subtle effects to obtain not only quantitative characteristics in addition to MDA, but also to obtain new qualitative results.
- Steinhauer V., Sergeev N.I. Radiomics in breast cancer: in-depth machine analysis of MR images of metastatic spine lesion. Sovremennye tehnologii v medicine 2022; 14(2): 16, https://doi.org/10.17691/stm2022.14.2.02.
- SourceForge. dcm4che, a DICOM Implementation in JAVA Files. URL: https://sourceforge.net/projects/dcm4che/files/dcm4che3.
- Zwanenburg A., Vallières M., Abdalah M.A., Aerts H.J.W.L., Andrearczyk V., Apte A., Ashrafinia S., Bakas S., Beukinga R.J., Boellaard R., Bogowicz M., Boldrini L., Buvat I., Cook G.J.R., Davatzikos C., Depeursinge A., Desseroit M.C., Dinapoli N., Dinh C.V., Echegaray S., El Naqa I., Fedorov A.Y., Gatta R., Gillies R.J., Goh V., Götz M., Guckenberger M., Ha S.M., Hatt M., Isensee F., Lambin P., Leger S., Leijenaar R.T.H., Lenkowicz J., Lippert F., Losnegård A., Maier-Hein K.H., Morin O., Müller H., Napel S., Nioche C., Orlhac F., Pati S., Pfaehler E.A.G., Rahmim A., Rao A.U.K., Scherer J., Siddique M.M., Sijtsema N.M., Socarras Fernandez J., Spezi E., Steenbakkers R.J.H.M., Tanadini-Lang S., Thorwarth D., Troost E.G.C., Upadhaya T., Valentini V., van Dijk L.V., van Griethuysen J., van Velden F.H.P., Whybra P., Richter C., Löck S. The image biomarker standardization initiative: standardized quantitative radiomics for high-throughput image-based phenotyping. Radiology 2020; 295(2): 328–338, https://doi.org/10.1148/radiol.2020191145.
- Solovieva S.N., Matkin A.E. Development of a method of MRI image evaluation based on values estimated by the Hounsfield scale. Sovremennye naukoemkie tekhnologii 2018; 4: 188–126. URL: https://top-technologies.ru/en/article/view?id=36970.
- BOOFCV. Image processing. URL: https://boofcv.org/index.php?title=Main_Page.
- Kendrick J., Francis R., Hassan G.M., Rowshanfarzad P., Jeraj R., Kasisi C., Rusanov B., Ebert M. Radiomics for identification and prediction in metastatic prostate cancer: a review of studies. Front Oncol 2021; 11: 771787, https://doi.org/10.3389/fonc.2021.771787.
- Catalano D. Normalization. URL: https://stackoverflow.com/questions/ 18576538/image-normalization-in-java/ 18752509#18752509.
- Sudirman S., Al Kafri A., Natalia F., Meidia H., Afriliana N., Al-Rashdan W., Bashtawi M., Al-Jumaily M. Lumbar spine MRI dataset. Mendeley Data 2019; V. 2. URL: https://data.mendeley.com/datasets/k57fr854j2.
- An open source perceptual hash library. URL: https://www.phash.org/.
- Neuroph. Java Neural Network Framework. URL: https://neuroph.sourceforge.net/.
- Deeplearning4J. URL: https://deeplearning4j.konduit.ai/.
- Steinhauer V., Steinhauer L. Neuroph und DL4J. Einsatz in einer medizinischen Java-Anwendung. Java Magazin 2021; 06: 79–82.
- Sergeev N.I., Kotlyarov P.M., Solodkiy V.A. Differential diagnosis of focal changes in the spine using standard and radiologic analysis. N.N. Priorova Journal of Traumatology and Orthopedics 2023; 30(1): 77–86, https://doi.org/10.17816/vto322858.