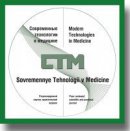
Тренды популяционных исследований: молекулярная и цифровая эпидемиология (обзор)
В последнее десятилетие развитие высокопроизводительных технологий привело к резкому увеличению возможностей изучения организма на молекулярном, клеточном и организменном уровнях. Быстрый прогресс биотехнологий стал причиной смены парадигмы в популяционных исследованиях. Достижения современных биомедицинских наук, включая геномные, полногеномные, постгеномные исследования и биоинформатику, способствовали появлению молекулярной эпидемиологии, принцип которой заключается в изучении персонализированного молекулярного механизма развития заболеваний и его экстраполяции на уровень популяции. Работа научных групп на стыке информационных технологий и медицины стала основанием для выделения цифровой эпидемиологии, важными инструментами которой являются машинное обучение, возможность работы с реальными данными (real world data, RWD) и накопленные большие данные.
Разрабатываемые подходы позволяют ускорить процесс сбора и обработки биомедицинских данных, проверку новых научных гипотез. Однако новые методы находятся пока на стадии становления, требуют проверки применения в различных условиях, а также стандартизации. Данный обзор освещает роль омиксных и цифровых технологий в проведении популяционных исследований.
- Diaz-Beltran L., Cano C., Wall D.P., Esteban F.J. Systems biology as a comparative approach to understand complex gene expression in neurological diseases. Behav Sci (Basel) 2013; 3(2): 253–272, https://doi.org/10.3390/bs3020253.
- Wang Q., Lu Q., Zhao H. A review of study designs and statistical methods for genomic epidemiology studies using next generation sequencing. Front Genet 2015; 6: 149, https://doi.org/10.3389/fgene.2015.00149.
- Pareek C.S., Smoczynski R., Tretyn A. Sequencing technologies and genome sequencing. J Appl Genet 2011; 52(4): 413–435, https://doi.org/10.1007/s13353-011-0057-x.
- Hill V., Ruis C., Bajaj S., Pybus O.G., Kraemer M.U.G. Progress and challenges in virus genomic epidemiology. Trends Parasitol 2021; 37(12): 1038–1049, https://doi.org/10.1016/j.pt.2021.08.007.
- Armstrong G.L., MacCannell D.R., Taylor J., Carleton H.A., Neuhaus E.B., Bradbury R.S., Posey J.E., Gwinn M. Pathogen genomics in public health. N Engl J Med 2019; 381(26): 2569–2580, https://doi.org/10.1056/nejmsr1813907.
- Ladner J.T., Grubaugh N.D., Pybus O.G., Andersen K.G. Precision epidemiology for infectious disease control. Nat Med 2019; 25(2): 206–211, https://doi.org/10.1038/s41591-019-0345-2.
- Holmes E.C., Dudas G., Rambaut A., Andersen K.G. The evolution of Ebola virus: insights from the 2013–2016 epidemic. Nature 2016; 538(7624): 193–200, https://doi.org/10.1038/nature19790.
- Kulikowski C.A. Pandemics: historically slow “learning curve” leading to biomedical informatics and vaccine breakthroughs. Yearb Med Inform 2021; 30(1): 290–301, https://doi.org/10.1055/s-0041-1726482.
- National Academies of Sciences, Engineering, and Medicine; Division on Earth and Life Studies; Board on Life Sciences; Health and Medicine Division; Board on Health Sciences Policy; Committee on Data Needs to Monitor the Evolution of SARS-CoV-2. Genomic epidemiology data infrastructure needs for SARS-CoV-2: modernizing pandemic response strategies. Washington (DC): National Academies Press (US); 2020. URL: https://www.ncbi.nlm.nih.gov/books/NBK562776/.
- Liu T., Chen Z., Chen W., Chen X., Hosseini M., Yang Z., Li J., Ho D., Turay D., Gheorghe C.P., Jones W., Wang C. A benchmarking study of SARS-CoV-2 whole-genome sequencing protocols using COVID-19 patient samples. iScience 2021; 24(8): 102892, https://doi.org/10.1016/j.isci.2021.102892.
- Richard M., Knauf S., Lawrence P., Mather A.E., Munster V.J., Müller M.A., Smith D., Kuiken T. Factors determining human-to-human transmissibility of zoonotic pathogens via contact. Curr Opin Virol 2017; 22: 7–12, https://doi.org/10.1016/j.coviro.2016.11.004.
- Gilchrist C.A., Turner S.D., Riley M.F., Petri W.A., Hewlett E.L. Whole-genome sequencing in outbreak analysis. Clin Microbiol Rev 2015; 28(3): 541–563, https://doi.org/10.1128/cmr.00075-13.
- Grad Y.H., Lipsitch M. Epidemiologic data and pathogen genome sequences: a powerful synergy for public health. Genome Biol 2014; 15(11): 538, https://doi.org/10.1186/s13059-014-0538-4.
- Wohl S., Schaffner S.F., Sabeti P.C. Genomic analysis of viral outbreaks. Annu Rev Virol 2016; 3(1): 173–195, https://doi.org/10.1146/annurev-virology-110615-035747.
- Maljkovic Berry I., Melendrez M.C., Bishop-Lilly K.A., Rutvisuttinunt W., Pollett S., Talundzic E., Morton L., Jarman R.G. Next generation sequencing and bioinformatics methodologies for infectious disease research and public health: approaches, applications, and considerations for development of laboratory capacity. J Infect Dis 2020; 221(Suppl 3): S292–S307, https://doi.org/10.1093/infdis/jiz286.
- Bandoy D.J.D.R., Weimer B.C. Analysis of SARS-CoV-2 genomic epidemiology reveals disease transmission coupled to variant emergence and allelic variation. Sci Rep 2021; 11(1): 7380, https://doi.org/10.1038/s41598-021-86265-4.
- Gonzaga-Jauregui C., Lupski J.R., Gibbs R.A. Human genome sequencing in health and disease. Annu Rev Med 2012; 63: 35–61, https://doi.org/10.1146/annurev-med-051010-162644.
- Cleeren E., Van der Heyden J., Brand A., Van Oyen H. Public health in the genomic era: will Public Health Genomics contribute to major changes in the prevention of common diseases? Arch Public Health 2011; 69(1): 8, https://doi.org/10.1186/0778-7367-69-8.
- McGuire A.L., Beskow L.M. Informed consent in genomics and genetic research. Annu Rev Genomics Hum Genet 2010; 11: 361–381, https://doi.org/10.1146/annurev-genom-082509-141711.
- Picó C., Serra F., Rodríguez A.M., Keijer J., Palou A. Biomarkers of nutrition and health: new tools for new approaches. Nutrients 2019; 11(5): 1092, https://doi.org/10.3390/nu11051092.
- Fearnley L.G., Inouye M. Metabolomics in epidemiology: from metabolite concentrations to integrative reaction networks. Int J Epidemiol 2016; 45(5): 1319–1328, https://doi.org/10.1093/ije/dyw046.
- Testa C., Pultrone C., Manners D.N., Schiavina R., Lodi R. Metabolic imaging in prostate cancer: where we are. Front Oncol 2016; 6: 225, https://doi.org/10.3389/fonc.2016.00225.
- Luptak I., Sverdlov A.L., Panagia M., Qin F., Pimentel D.R., Croteau D., Siwik D.A., Ingwall J.S., Bachschmid M.M., Balschi J.A., Colucci W.S. Decreased ATP production and myocardial contractile reserve in metabolic heart disease. J Mol Cell Cardiol 2018; 116: 106–114, https://doi.org/10.1016/j.yjmcc.2018.01.017.
- Metallo C.M., Heiden M.G.V. Understanding metabolic regulation and its influence on cell physiology. Mol Cell 2013; 49(3): 388–398, https://doi.org/10.1016/j.molcel.2013.01.018.
- Johnson C.H., Ivanisevic J., Siuzdak G. Metabolomics: beyond biomarkers and towards mechanisms. Nat Rev Mol Cell Biol 2016; 17(7): 451–459, https://doi.org/10.1038/nrm.2016.25.
- Bouatra S., Aziat F., Mandal R., Guo A.C., Wilson M.R., Knox C., Bjorndahl T.C., Krishnamurthy R., Saleem F., Liu P., Dame Z.T., Poelzer J., Huynh J., Yallou F.S., Psychogios N., Dong E., Bogumil R., Roehring C., Wishart D.S. The human urine metabolome. PLoS One 2013; 8(9): e73076, https://doi.org/10.1371/journal.pone.0073076.
- Psychogios N., Hau D.D., Peng J., Guo A.C., Mandal R., Bouatra S., Sinelnikov I., Krishnamurthy R., Eisner R., Gautam B., Young N., Xia J., Knox C., Dong E., Huang P., Hollander Z., Pedersen T.L., Smith S.R., Bamforth F., Greiner R., McManus B., Newman J.W., Goodfriend T., Wishart D.S. The human serum metabolome. PLoS One 2011; 6(2): e16957, https://doi.org/10.1371/journal.pone.0016957.
- Karu N., Deng L., Slae M., Guo A.C., Sajed T., Huynh H., Wine E., Wishart D.S. A review on human fecal metabolomics: methods, applications and the human fecal metabolome database. Anal Chim Acta 2018; 1030: 1–24, https://doi.org/10.1016/j.aca.2018.05.031.
- Wishart D.S., Lewis M.J., Morrissey J.A., Flegel M.D., Jeroncic K., Xiong Y., Cheng D., Eisner R., Gautam B., Tzur D., Sawhney S., Bamforth F., Greiner R., Li L. The human cerebrospinal fluid metabolome. J Chromatogr B 2008; 871(2): 164–173, https://doi.org/10.1016/j.jchromb.2008.05.001.
- Brown M.V., McDunn J.E., Gunst P.R., Smith E.M., Milburn M.V., Troyer D.A., Lawton K.A. Cancer detection and biopsy classification using concurrent histopathological and metabolomic analysis of core biopsies. Genome Med 2012; 4(4): 33, https://doi.org/10.1186/gm332.
- Robinson O., Keski-Rahkonen P., Chatzi L., Kogevinas M., Nawrot T., Pizzi C., Plusquin M., Richiardi L., Robinot N., Sunyer J., Vermeulen R., Vrijheid M., Vineis P., Scalbert A., Chadeau-Hyam M. Cord blood metabolic signatures of birth weight: a population-based study. J Proteome Res 2018; 17(3): 1235–1247, https://doi.org/10.1021/acs.jproteome.7b00846.
- Perng W., Rifas-Shiman S.L., McCulloch S., Chatzi L., Mantzoros C., Hivert M.F., Oken E. Associations of cord blood metabolites with perinatal characteristics, newborn anthropometry, and cord blood hormones in project viva. Metabolism 2017; 76: 11–22, https://doi.org/10.1016/j.metabol.2017.07.001.
- Lau C.E., Siskos A.P., Maitre L., Robinson O., Athersuch T.J., Want E.J., Urquiza J., Casas M., Vafeiadi M., Roumeliotaki T., McEachan R.R.C., Azad R., Haug L.S., Meltzer H.M., Andrusaityte S., Petraviciene I., Grazuleviciene R., Thomsen C., Wright J., Slama R., Chatzi L., Vrijheid M., Keun H.C., Coen M. Determinants of the urinary and serum metabolome in children from six European populations. BMC Med 2018; 16(1): 202, https://doi.org/10.1186/s12916-018-1190-8.
- Petrick L., Edmands W., Schiffman C., Grigoryan H., Perttula K., Yano Y., Dudoit S., Whitehead T., Metayer C., Rappaport S. An untargeted metabolomics method for archived newborn dried blood spots in epidemiologic studies. Metabolomics 2017; 13(3): 27, https://doi.org/10.1007/s11306-016-1153-z.
- Roede J.R., Uppal K., Park Y., Lee K., Tran V., Walker D., Strobel F.H., Rhodes S.L., Ritz B., Jones D.P. Serum metabolomics of slow vs. rapid motor progression Parkinson’s disease: a pilot study. PLoS One 2013; 8(10): e77629, https://doi.org/10.1371/journal.pone.0077629.
- Guasch-Ferré M., Hruby A., Toledo E., Clish C.B., Martínez-González M.A., Salas-Salvadó J., Hu F.B. Metabolomics in prediabetes and diabetes: a systematic review and meta-analysis. Diabetes Care 2016; 39(5): 833–846, https://doi.org/10.2337/dc15-2251.
- Wishart D.S., Mandal R., Stanislaus A., Ramirez-Gaona M. Cancer metabolomics and the human metabolome database. Metabolites 2016; 6(1): 10, https://doi.org/10.3390/metabo6010010.
- Frediani J.K., Jones D.P., Tukvadze N., Uppal K., Sanikidze E., Kipiani M., Tran V.T., Hebbar G., Walker D.I., Kempker R.R., Kurani S.S., Colas R.A., Dalli J., Tangpricha V., Serhan C.N., Blumberg H.M., Ziegler T.R. Plasma metabolomics in human pulmonary tuberculosis disease: a pilot study. PLoS One 2014; 9(10): e108854, https://doi.org/10.1371/journal.pone.0108854.
- Würtz P., Havulinna A.S., Soininen P., Tynkkynen T., Prieto-Merino D., Tillin T., Ghorbani A., Artati A., Wang Q., Tiainen M., Kangas A.J., Kettunen J., Kaikkonen J., Mikkilä V., Jula A., Kähönen M., Lehtimäki T., Lawlor D.A., Gaunt T.R., Hughes A.D., Sattar N., Illig T., Adamski J., Wang T.J., Perola M., Ripatti S., Vasan R.S., Raitakari O.T., Gerszten R.E., Casas J.P., Chaturvedi N., Ala-Korpela M., Salomaa V. Metabolite profiling and cardiovascular event risk. Circulation 2015; 131(9): 774–785, https://doi.org/10.1161/circulationaha.114.013116.
- Ganna A., Salihovic S., Sundström J., Broeckling C.D., Hedman Å.K., Magnusson P.K.E., Pedersen N.L., Larsson A., Siegbahn A., Zilmer M., Prenni J., Arnlöv J., Lind L., Fall T., Ingelsson E. Large-scale metabolomic profiling identifies novel biomarkers for incident coronary heart disease. PLos Genet 2014; 10(12): e1004801, https://doi.org/10.1371/journal.pgen.1004801.
- Rogers A.J., McGeachie M., Baron R.M., Gazourian L., Haspel J.A., Nakahira K., Fredenburgh L.E., Hunninghake G.M., Raby B.A., Matthay M.A., Otero R.M., Fowler V.G., Rivers E.P., Woods C.W., Kingsmore S., Langley R.J., Choi A.M. Metabolomic derangements are associated with mortality in critically ill adult patients. PLos One 2014; 9(1): e87538, https://doi.org/10.1371/journal.pone.0087538.
- Playdon M.C., Ziegler R.G., Sampson J.N., Stolzenberg-Solomon R., Thompson H.J., Irwin M.L., Mayne S.T., Hoover R.N., Moore S.C. Nutritional metabolomics and breast cancer risk in a prospective study. Am J Clin Nutr 2017; 106(2): 637–649, https://doi.org/10.3945/ajcn.116.150912.
- Анфиногенова Я.Д., Трубачева И.А., Серебрякова В.Н., Попов С.В. Новые тренды и вызовы популяционной кардиологии. Сибирский журнал клинической и экспериментальной медицины 2020; 34(4): 24–38, https://doi.org/10.29001/2073-8552-2019-34-4-24-38.
- Garg S., Williams N.L., Ip A., Dicker A.P. Clinical integration of digital solutions in health care: an overview of the current landscape of digital technologies in cancer care. JCO Clin Cancer Inform 2018; 2: 1–9, https://doi.org/10.1200/cci.17.00159.
- Thyagarajan B., Nelson H.H., Poynter J.N., Prizment A.E., Roesler M.A., Cassidy E., Putnam S., Amos L., Hickle A., Reilly C., Spector L.G., Lazovich D. Field application of digital technologies for health assessment in the 10,000 families study. Cancer Epidemiol Biomarkers Prev 2020; 29(4): 744–751, https://doi.org/10.1158/1055-9965.epi-19-0858.
- Coathup V., Teare H.J., Minari J., Yoshizawa G., Kaye J., Takahashi M.P., Kato K. Using digital technologies to engage with medical research: views of myotonic dystrophy patients in Japan. BMC Med Ethics 2016; 17(1): 51, https://doi.org/10.1186/s12910-016-0132-2.
- Брико Н.И. Теоретические обобщения в эпидемиологии: от истории к современности. Эпидемиология и вакцинопрофилактика 2018; 17(5): 5–16, https://doi.org/10.31631/2073-3046-2018-17-5-5-16.
- Jagodzinski A., Johansen C., Koch-Gromus U., Aarabi G., Adam G., Anders S., Augustin M., der Kellen R.B., Beikler T., Behrendt C.A., Betz C.S., Bokemeyer C., Borof K., Briken P., Busch C.J., Büchel C., Brassen S., Debus E.S., Eggers L., Fiehler J., Gallinat J., Gellißen S., Gerloff C., Girdauskas E., Gosau M., Graefen M., Härter M., Harth V., Heidemann C., Heydecke G., Huber T.B., Hussein Y., Kampf M.O., von dem Knesebeck O., Konnopka A., König H.H., Kromer R., Kubisch C., Kühn S., Loges S., Löwe B., Lund G., Meyer C., Nagel L., Nienhaus A., Pantel K., Petersen E., Püschel K., Reichenspurner H., Sauter G., Scherer M., Scherschel K., Schiffner U., Schnabel R.B., Schulz H., Smeets R., Sokalskis V., Spitzer M.S., Terschüren C., Thederan I., Thoma T., Thomalla G., Waschki B., Wegscheider K., Wenzel J.P., Wiese S., Zyriax B.C., Zeller T., Blankenberg S. Rationale and design of the Hamburg city health study. Eur J Epidemiol 2020; 35(2): 169–181, https://doi.org/10.1007/s10654-019-00577-4.
- Shin Y.K., Koskinen V., Kouvonen A., Kemppainen T., Olakivi A., Wrede S., Kemppainen L. Digital information technology use and transnational healthcare: a population-based study on older Russian-speaking migrants in Finland. J Immigr Minor Health 2022; 24(1): 125–135, https://doi.org/10.1007/s10903-021-01301-9.
- Coppola L., Cianflone A., Grimaldi A.M., Incoronato M., Bevilacqua P., Messina F., Baselice S., Soricelli A., Mirabelli P., Salvatore M. Biobanking in health care: evolution and future directions. J Transl Med 2019; 17(1): 172, https://doi.org/10.1186/s12967-019-1922-3.
- Griffon N., Pereira H., Djadi-Prat J., García M.T., Testoni S., Cariou M., Hilbey J., N’Dja A., Navarro G., Gentili N., Nanni O., Raineri M., Chatellier G., Gómez De La Camara A., Lewi M., Sundgren M., Daniel C., Garvey A., Todorovic M., Ammour N. Performances of a solution to semi-automatically fill eCRF with data from the electronic health record: protocol for a prospective individual participant data meta-analysis. Stud Health Technol Inform 2020; 270: 367–371, https://doi.org/10.3233/shti200184.
- Rorie D.A., Flynn R.W.V., Grieve K., Doney A., Mackenzie I., MacDonald T.M., Rogers A. Electronic case report forms and electronic data capture within clinical trials and pharmacoepidemiology. Br J Clin Pharmacol 2017; 83(9): 1880–1895, https://doi.org/10.1111/bcp.13285.
- Mayerhoefer M.E., Materka A., Langs G., Häggström I., Szczypiński P., Gibbs P., Cook G. Introduction to radiomics. J Nucl Med 2020; 61(4): 488–495, https://doi.org/10.2967/jnumed.118.222893.
- Benelli M., Barucci A., Zoppetti N., Calusi S., Redapi L., Della Gala G., Piffer S., Bernardi L., Fusi F., Pallotta S. Comprehensive analysis of radiomic datasets by RadAR. Cancer Res 2020; 80(15): 3170–3174, https://doi.org/10.1158/0008-5472.can-20-0332.
- Scapicchio C., Gabelloni M., Barucci A., Cioni D., Saba L., Neri E. A deep look into radiomics. Radiol Med 2021; 126(10): 1296–1311, https://doi.org/10.1007/s11547-021-01389-x.
- Gagalova K.K., Leon Elizalde M.A., Portales-Casamar E., Görges M. What you need to know before implementing a clinical research data warehouse: comparative review of integrated data repositories in health care institutions. JMIR Form Res 2020; 4(8): e17687, https://doi.org/10.2196/17687.
- Huser V., Kahn M.G., Brown J.S., Gouripeddi R. Methods for examining data quality in healthcare integrated data repositories. Pac Symp Biocomput 2018; 23: 628–633.
- Ku W.Y., Nfor O.N., Liu W.H., Tantoh D.M., Hsu S.Y., Wang L., Chou T.Y., Liaw Y.P. Online community collaborative map: a geospatial and data visualization tool for cancer data. Medicine (Baltimore) 2019; 98(20): e15521, https://doi.org/10.1097/md.0000000000015521.
- Commons R.J., Thriemer K., Humphreys G., Suay I., Sibley C.H., Guerin P.J., Price R.N. The Vivax Surveyor: online mapping database for Plasmodium vivax clinical trials. Int J Parasitol Drugs Drug Resist 2017; 7(2): 181–190, https://doi.org/10.1016/j.ijpddr.2017.03.003.
- Dong E., Du H., Gardner L. An interactive web-based dashboard to track COVID-19 in real time. Lancet Infect Dis 2020; 20(5): 533–534, https://doi.org/10.1016/s1473-3099(20)30120-1.
- Kuzmenkov A.Y., Trushin I.V., Vinogradova A.G., Avramenko A.A., Sukhorukova M.V., Malhotra-Kumar S., Dekhnich A.V., Edelstein M.V., Kozlov R.S. AMRmap: an interactive web platform for analysis of antimicrobial resistance surveillance data in Russia. Front Microbiol 2021; 12: 620002, https://doi.org/10.3389/fmicb.2021.620002.
- Liu Y., Elsworth B., Erola P., Haberland V., Hemani G., Lyon M., Zheng J., Lloyd O., Vabistsevits M., Gaunt T.R. EpiGraphDB: a database and data mining platform for health data science. Bioinformatics 2021; 37(9): 1304–1311, https://doi.org/10.1093/bioinformatics/btaa961.
- Hemani G., Zheng J., Elsworth B., Wade K.H., Haberland V., Baird D., Laurin C., Burgess S., Bowden J., Langdon R., Tan V.Y., Yarmolinsky J., Shihab H.A., Timpson N.J., Evans D.M., Relton C., Martin R.M., Davey Smith G., Gaunt T.R., Haycock P.C. The MR-Base platform supports systematic causal inference across the human phenome. eLife 2018; 7: e34408, https://doi.org/10.7554/eLife.34408.
- Slenter D.N., Kutmon M., Hanspers K., Riutta A., Windsor J., Nunes N., Mélius J., Cirillo E., Coort S.L., Digles D., Ehrhart F., Giesbertz P., Kalafati M., Martens M., Miller R., Nishida K., Rieswijk L., Waagmeester A., Eijssen L.M.T., Evelo C.T., Pico A.R., Willighagen E.L. WikiPathways: a multifaceted pathway database bridging metabolomics to other omics research. Nucleic Acids Res 2018; 46(D1): D661–D667, https://doi.org/10.1093/nar/gkx1064.
- Szklarczyk D., Morris J.H., Cook H., Kuhn M., Wyder S., Simonovic M., Santos A., Doncheva N.T., Roth A., Bork P., Jensen L.J., von Mering C. The STRING database in 2017: quality-controlled protein-protein association networks, made broadly accessible. Nucleic Acids Res 2017; 45(D1): D362–D368, https://doi.org/10.1093/nar/gkw937.
- Toom K., Raidvee A., Braschinsky M. The applicability of web-based solutions in headache epidemiology research. J Headache Pain 2020; 21(1): 60, https://doi.org/10.1186/s10194-020-01141-2.
- Egger M.J., Lukacz E.S., Newhouse M., Wang J., Nygaard I. Web versus paper-based completion of the epidemiology of prolapse and incontinence questionnaire. Female Pelvic Med Reconstr Surg 2013; 19(1): 17–22, https://doi.org/10.1097/spv.0b013e31827bfd93.
- Tassiopoulos K., Roberts-Toler C., Fichtenbaum C.J., Koletar S.L. Web-based data collection for older adults living with HIV in a clinical research setting: pilot observational study. J Med Internet Res 2020; 22(11): e18588, https://doi.org/10.2196/18588.
- Braekman E., Charafeddine R., Demarest S., Drieskens S., Berete F., Gisle L., Van der Heyden J., Van Hal G. Comparing web-based versus face-to-face and paper-and-pencil questionnaire data collected through two Belgian health surveys. Int J Public Health 2020; 65(1): 5–16, https://doi.org/10.1007/s00038-019-01327-9.
- Jeon Y.H., Shin M., Smith A., Beattie E., Brodaty H., Frost D., Hobbs A., Kotting P., Petrie G., Rossor M., Thompson J., Vickers J., Waters D. Early implementation and evaluation of StepUp for Dementia Research: an Australia-wide dementia research participation and public engagement platform. Int J Environ Res Public Health 2021; 18(21): 11353, https://doi.org/10.3390/ijerph182111353.
- Langbaum J.B., Karlawish J., Roberts J.S., Wood E.M., Bradbury A., High N., Walsh T.L., Gordon D., Aggarwal R., Davis P., Stowell C., Trisko L., Langlois C.M., Reiman E.M., Tariot P.N. GeneMatch: a novel recruitment registry using at-home APOE genotyping to enhance referrals to Alzheimer’s prevention studies. Alzheimers Dement J Alzheimers Assoc 2019; 15(4): 515–524, https://doi.org/10.1016/j.jalz.2018.12.007.
- Weiner M.W., Nosheny R., Camacho M., Truran-Sacrey D., Mackin R.S., Flenniken D., Ulbricht A., Insel P., Finley S., Fockler J., Veitch D. The Brain Health Registry: an internet-based platform for recruitment, assessment, and longitudinal monitoring of participants for neuroscience studies. Alzheimers Dement J Alzheimers Assoc 2018; 14(8): 1063–1076, https://doi.org/10.1016/j.jalz.2018.02.021.
- Harris P.A., Taylor R., Minor B.L., Elliott V., Fernandez M., O’Neal L., McLeod L., Delacqua G., Delacqua F., Kirby J., Duda S.N.; REDCap Consortium. The REDCap consortium: building an international community of software platform partners. J Biomed Inform 2019; 95: 103208, https://doi.org/10.1016/j.jbi.2019.103208.
- Ranaweera T., Makalic E., Hopper J.L., Bickerstaffe A. An open-source, integrated pedigree data management and visualization tool for genetic epidemiology. Int J Epidemiol 2018; 47(4): 1034–1039, https://doi.org/10.1093/ije/dyy049.
- Djenno M., Insua G.M., Pho A. From paper to pixels: using Google Forms for collaboration and assessment. Libr Hi Tech News 2015; 32(4): 9–13, https://doi.org/10.1108/lhtn-12-2014-0105.
- Wang X. Establishment of an internet-based epidemiological survey data collection customized system model. Front Public Health 2021; 9: 761031, https://doi.org/10.3389/fpubh.2021.761031.
- Golinelli D., Boetto E., Carullo G., Nuzzolese A.G., Landini M.P., Fantini M.P. Adoption of digital technologies in health care during the COVID-19 pandemic: systematic review of early scientific literature. J Med Internet Res 2020; 22(11): e22280, https://doi.org/10.2196/22280.
- Budd J., Miller B.S., Manning E.M., Lampos V., Zhuang M., Edelstein M., Rees G., Emery V.C., Stevens M.M., Keegan N., Short M.J., Pillay D., Manley E., Cox I.J., Heymann D., Johnson A.M., McKendry R.A. Digital technologies in the public-health response to COVID-19. Nat Med 2020; 26(8): 1183–1192, https://doi.org/10.1038/s41591-020-1011-4.
- He Z., Zhang C.J.P., Huang J., Zhai J., Zhou S., Chiu J.W., Sheng J., Tsang W., Akinwunmi B.O., Ming W.K. A new era of epidemiology: digital epidemiology for investigating the COVID-19 outbreak in China. J Med Internet Res 2020; 22(9): e21685, https://doi.org/10.2196/21685.
- Horst B.R., Sixsmith A., Simeonov D., Mihailidis A. Demographic and psychographic factors of social isolation during the COVID-19 pandemic: the importance of technology confidence. Front Public Health 2021; 9: 749515, https://doi.org/10.3389/fpubh.2021.749515.
- Wilkinson M.D., Dumontier M., Jan Aalbersberg I., Appleton G., Axton M., Baak A., Blomberg N., Boiten J.W., da Silva Santos L.B., Bourne P.E., Bouwman J., Brookes A.J., Clark T., Crosas M., Dillo I., Dumon O., Edmunds S., Evelo C.T., Finkers R., Gonzalez-Beltran A., Gray A.J.G., Groth P., Goble C., Grethe J.S., Heringa J., ‘t Hoen P.A.C., Hooft R., Kuhn T., Kok R., Kok J., Lusher S.J., Martone M.E., Mons A., Packer A.L., Persson B., Rocca-Serra P., Roos M., van Schaik R., Sansone S.A., Schultes E., Sengstag T., Slater T., Strawn G., Swertz M.A., Thompson M., van der Lei J., van Mulligen E., Velterop J., Waagmeester A., Wittenburg P., Wolstencroft K., Zhao J., Mons B. Addendum: the FAIR Guiding Principles for scientific data management and stewardship. Sci Data 2019; 6(1): 6, https://doi.org/10.1038/s41597-019-0009-6.
- Munafò M.R., Hollands G.J., Marteau T.M. Open science prevents mindless science. BMJ 2018; 363: k4309, https://doi.org/10.1136/bmj.k4309.
- Sanjana N.E. Voices of the new generation: open science is good for science (and for you). Nat Rev Mol Cell Biol 2021; 22(11): 709, https://doi.org/10.1038/s41580-021-00414-1.
- Suhre K., McCarthy M.I., Schwenk J.M. Genetics meets proteomics: perspectives for large population-based studies. Nat Rev Genet 2021; 22(1): 19–37, https://doi.org/10.1038/s41576-020-0268-2.
- Bian J., Lyu T., Loiacono A., Viramontes T.M., Lipori G., Guo Y., Wu Y., Prosperi M., George T.J., Harle C.A., Shenkman E.A., Hogan W. Assessing the practice of data quality evaluation in a national clinical data research network through a systematic scoping review in the era of real-world data. J Am Med Inform Assoc 2020; 27(12): 1999–2010, https://doi.org/10.1093/jamia/ocaa245.
- Brownstein J.S., Freifeld C.C., Reis B.Y., Mandl K.D. Surveillance Sans Frontières: internet-based emerging infectious disease intelligence and the HealthMap project. PLoS Med 2008; 5(7): e151, https://doi.org/10.1371/journal.pmed.0050151.
- Land-Zandstra A.M., van Beusekom M., Koppeschaar C., van den Broek J. Motivation and learning impact of Dutch flu-trackers. J Sci Commun 2016; 15(1): A04, https://doi.org/10.22323/2.15010204.
- Paolotti D., Carnahan A., Colizza V., Eames K., Edmunds J., Gomes G., Koppeschaar C., Rehn M., Smallenburg R., Turbelin C., Van Noort S., Vespignani A. Web-based participatory surveillance of infectious diseases: the Influenzanet participatory surveillance experience. Clin Microbiol Infect 2014; 20(1): 17–21, https://doi.org/10.1111/1469-0691.12477.
- Chatzidimitriou A., Minga E., Chatzikonstantinou T., Moreno C., Stamatopoulos K., Ghia P. Challenges and solutions for collecting and analyzing real world data: the Eric CLL database as an illustrative example. Hemasphere 2020; 4(5): e425, https://doi.org/10.1097/hs9.0000000000000425.
- Salathé M. Digital epidemiology: what is it, and where is it going? Life Sci Soc Policy 2018; 14(1): 1, https://doi.org/10.1186/s40504-017-0065-7.
- Ginsberg J., Mohebbi M.H., Patel R.S., Brammer L., Smolinski M.S., Brilliant L. Detecting influenza epidemics using search engine query data. Nature 2009; 457(7232): 1012–1014, https://doi.org/10.1038/nature07634.
- Lippi G., Mattiuzzi C., Cervellin G. Is digital epidemiology the future of clinical epidemiology? J Epidemiol Glob Health 2019; 9(2): 146, https://doi.org/10.2991/jegh.k.190314.003.
- Cervellin G., Comelli I., Lippi G. Is Google Trends a reliable tool for digital epidemiology? Insights from different clinical settings. J Epidemiol Glob Health 2017; 7(3): 185–189, https://doi.org/10.1016/j.jegh.2017.06.001.
- Roth J.A., Battegay M., Juchler F., Vogt J.E., Widmer A.F. Introduction to machine learning in digital healthcare epidemiology. Infect Control Hosp Epidemiol 2018; 39(12): 1457–1462, https://doi.org/10.1017/ice.2018.265.
- Frérot M., Lefebvre A., Aho S., Callier P., Astruc K., Aho Glélé L.S. What is epidemiology? Changing definitions of epidemiology 1978–2017. PLoS One 2018; 13(12): e0208442, https://doi.org/10.1371/journal.pone.0208442.
- Werler M.M., Stuver S.O., Healey M.A., LaMorte W.W. The future of teaching epidemiology. Am J Epidemiol 2019; 188(5): 825–829, https://doi.org/10.1093/aje/kwz039.
- Vallury K.D., Baird B., Miller E., Ward P. Going viral: researching safely on social media. J Med Internet Res 2021; 23(12): e29737, https://doi.org/10.2196/29737.
- Juengst E.T., Van Rie A. Transparency, trust, and community welfare: towards a precision public health ethics framework for the genomics era. Genome Med 2020; 12(1): 98, https://doi.org/10.1186/s13073-020-00800-y.
- Solomon D.H., Rudin R.S. Digital health technologies: opportunities and challenges in rheumatology. Nat Rev Rheumatol 2020; 16(9): 525–535, https://doi.org/10.1038/s41584-020-0461-x.
- Sharma A., Nilsen T.B., Czerwinska K.P., Onitiu D., Brenna L., Johansen D., Johansen H.D. Up-to-the-minute privacy policies via gossips in participatory epidemiological studies. Front Big Data 2021; 4: 624424, https://doi.org/10.3389/fdata.2021.624424.
- Holub P., Kohlmayer F., Prasser F., Mayrhofer M.T., Schlünder I., Martin G.M., Casati S., Koumakis L., Wutte A., Kozera Ł., Strapagiel D., Anton G., Zanetti G., Sezerman O.U., Mendy M., Valík D., Lavitrano M., Dagher G., Zatloukal K., van Ommen G.B., Litton J.E. Enhancing reuse of data and biological material in medical research: from FAIR to FAIR-Health. Biopreserv Biobank 2018; 16(2): 97–105, https://doi.org/10.1089/bio.2017.0110.