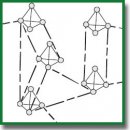
Architecture of a Wheelchair Control System for Disabled People: Towards Multifunctional Robotic Solution with Neurobiological Interfaces
The aim of the study was to develop a control system for a robotic wheelchair with an extensive user interface that is able to support users with different impairments.
Different concepts for a robotic wheelchair design for disabled people are discussed. The selected approach is based on a cognitive multimodal user interface to maximize autonomy of the wheelchair user and to allow him or her to communicate intentions by high-level instructions. Manual, voice, eye tracking, and BCI (brain–computer interface) signals can be used for strategic control whereas an intelligent autonomous system can perform low-level control. A semiotic model of the world processes sensory data and plans actions as a sequence of high-level tasks or behaviors for the control system.
A software and hardware architecture for the robotic wheelchair and its multimodal user interface was proposed. This architecture supports several feedback types for the user including voice messages, screen output, as well as various light indications and tactile signals.
The paper describes novel solutions that have been tested on real robotic devices. The prototype of the wheelchair uses a wide range of sensors such as a camera, range finders, and encoders to allow operator to move safely and provide object and scene recognition capabilities for the wheelchair. Dangerous behavior of the robot is interrupted by low-level reflexes. Additional high-level safety procedures can be implemented for the planning subsystem.
The developed architecture allows utilizing user interfaces with a considerable time lag that are usually not suitable for traditional automated wheelchair control. This is achieved by increasing time allocated for processing of the interface modules, which is known to increase the accuracy of such interfaces as voice, eye tracking, and BCI. The increased latency of commands is mitigated by the increased automation of the wheelchair since high-level tasks can be given less frequently than manual control. The prospective solutions use a number of technologies based on registration of parameters of human physiological systems, including brain neural networks, in relation to the task of indirect control and interaction with mobile technical systems.
- World Health Organization. The world report on disability. 2011.
- Report of a consensus conference on wheelchairs for developing countries. Edited by Sheldon S., Jacobs N.A. Bengaluru, India; 2006.
- Shin J., Kwon J., Choi J.,
Im C.-H. Performance enhancement of abrain–computer interface using high-density multi-distance NIRS. Sci Rep 2017; 7(1): 16545, https://doi.org/10.1038/s41598-017-16639-0. - Na’aman E., Shashua A., Wexler Y. User wearable visual assistance system. Patent US 2012/0212593 A1. 2012.
- Clark J.A., Roemer R.B. Voice controlled
wheelchair . Arch Phys Med Rehabil 1977; 58(4): 169–175. - Nishimori M., Saitoh T., Konishi R.
Voice controlled intelligent wheelchair. In: SICE annual conference. IEEE; 2007, p. 336–340, https://doi.org/10.1109/sice.2007.4421003. - Tamura H., Manabe T., Goto T., Yamashita Y., Tanno K. A study of the electric wheelchair hands-free safety control system using the surface-
electromygram of facial muscles. In: Liu H., Ding H., Xiong Z., Zhu X. (editors). Intelligent robotics and applications. ICIRA 2010. Lecture Notes in Computer Science. Springer, Berlin, Heidelberg; 2010; p. 97–104, https://doi.org/10.1007/978-3-642-16587-0_10. - Kim K.-H., Kim H.K., Kim J.-S., Son W., Lee S.-Y. A biosignal-based human interface controlling a power-wheelchair for people with motor disabilities. ETRI Journal 2006; 28(1): 111–114, https://doi.org/10.4218/etrij.06.0205.0069.
- Yulianto E.,
Indrato T.B., Suharyati. The design of electrical wheelchairs with electromyography signal controller for people with paralysis. Electrical and Electronic Engineering 2018; 8(1): 1–9. - Hardiansyah R., Ainurrohmah A., Aniroh Y., Tyas F.H. The electric wheelchair control using electromyography sensor of arm muscle. Int Conf Inf Commun Technol Syst 2016; 2016: 129–134, https://doi.org/10.1109/icts.2016.7910286.
- Jacob R.J.K. The use of eye movements in human-computer interaction techniques: what you look at is what you get. ACM Trans Inf Syst 1991; 9(2): 152–169, https://doi.org/10.1145/123078.128728.
- Fedorova A.A., Shishkin S.L., Nuzhdin Y.O., Velichkovsky B.M.
Gaze based robot control: the communicative approach. In: 7th International IEEE/EMBS conference on neural engineering (NER). IEEE; 2015; p. 751–754, https://doi.org/10.1109/ner.2015.7146732. - Ingram R. Eye-tracking wheelchair developed by DoC researchers featured in Reuters video. 2014. URL: http://www.imperial.ac.uk/news/152966/eye-tracking-wheelchair-developed-doc-researchers-featured.
- Velichkovsky B.M. Communicating attention: gaze position transfer in cooperative
problem solving . Pragmatics & Cognition 1995; 3(2): 199–223, https://doi.org/10.1075/pc.3.2.02vel. - Ben Taher F., Ben Amor N., Jallouli M. EEG control of an electric wheelchair for disabled persons. International Conference on Individual and Collective Behaviors in Robotics 2013; 2013: 27–32, https://doi.org/10.1109/icbr.2013.6729275.
- Swee S.K., Teck Kiang K.D., You L.Z. EEG controlled
wheelchair . MATEC Web of Conferences 2016; 51: 02011, https://doi.org/10.1051/matecconf/20165102011. - Rebsamen B., Guan C., Zhang H., Wang C., Teo C., Ang M.H. Jr., Burdet E. A
brain controlled wheelchair to navigate in familiar environments. IEEE Trans Neural Syst Rehabil Eng 2010; 18(6): 590–598, https://doi.org/10.1109/tnsre.2010.2049862. - Naseer N., Hong K.-S. fNIRS-based brain-computer interfaces: a review. Front Hum Neurosci 2015; 9: 3, https://doi.org/10.3389/fnhum.2015.00003.
- Frisoli A., Loconsole C., Leonardis D., Banno F., Barsotti M., Chisari C., Bergamasco M. A new gaze-BCI-driven control of an upper limb exoskeleton for rehabilitation in real-world tasks. IEEE Transactions on Systems, Man, and Cybernetics, Part C 2012; 42(6): 1169–1179, https://doi.org/10.1109/tsmcc.2012.2226444.
- Osipov G.S., Panov A.I., Chudova N.V., Kuznetsova Yu.M. Znakovaya kartina mira sub”ekta povedeniya [Semiotic view of world for behavior subject]. Moscow: Fizmatlit; 2018.
- Saxena A., Jain A., Sener O., Jami A., Misra D.K., Koppula H.S. RoboBrain: large-scale knowledge engine for robots. 2014. URL: https://arxiv.org/abs/1412.0691v2.
- Skarzynski K., Stepniak M., Bartyna W., Ambroszkiewicz S. SO-MRS: a multi-robot system architecture based on the SOA paradigm and ontology. Lecture Notes in Computer Science 2018; 10965: 330–342, https://doi.org/10.1007/978-3-319-96728-8_28.
- Fikes R.E., Nilsson N.J. Strips: a new approach to the application of theorem proving to
problem solving . Artificial Intelligence 1971; 2(3–4): 189–208, https://doi.org/10.1016/0004-3702(71)90010-5. - Kiselev G.A., Panov A.I. STRIPS postanovka zadachi planirovaniya povedeniya v znakovoy kartine mira. V kn.: Informatika, upravlenie i sistemnyy analiz [STRIPS formulation of behavior planning problem in semiotic view of the world. In: Informatics, management, and system analysis]. Tver; 2016; p. 131–138.
- Karpov V. The parasitic manipulation of an
animat’s behavior. Biologically Inspired Cognitive Architectures 2017; 21: 67–74, https://doi.org/10.1016/j.bica.2017.05.002. - Sutton R.S., Barto A.G. Reinforcement learning: an introduction. IEEE Transactions on Neural Networks 1998; 9(5): 1054–1054, https://doi.org/10.1109/tnn.1998.712192.
- Narin’yani A.S., Borde S.B., Ivanov D.A. Subdefinite mathematics
and novel scheduling technology. Artificial Intelligence in Engineering 1997; 11(1): 5–14, https://doi.org/10.1016/0954-1810(96)00015-5. - Moscowsky A.D. Metod raspoznavaniya stsen na osnove nedoopredelennykh modeley. V kn.: Shestnadtsataya natsional’naya konferentsiya po iskusstvennomu intellektu s mezhdunarodnym uchastiem KII-2018 (24–27 sentyabrya 2018 g., Moskva, Rossiya). Tom 2 [Scene recognition method using non fully defined models. In: 16th Russian conference on artificial intelligence, RCAI 2018 (September 24–27, 2018, Moscow, Russia). Vol. 2]. Moscow: NIU VShE; 2018; p. 27–34.
- Moscowsky A.D. Ob odnom metode raspoznavaniya ob”ektov s ne polnost’yu opredelennymi priznakami. V kn.: Tretiy Vserossiyskiy nauchno-prakticheskiy seminar “Bespilotnye transportnye sredstva s elementami iskusstvennogo intellekta” (BTS-II-2016, 22–23 sentyabrya 2016 g., Innopolis, Respublika Tatarstan, Rossiya) [On a recognition method for objects with non fully defined feature set. In: III All-Russian scientific-practical seminar “Remotely piloted aircraft systems with the elements of artifitial intelligence” (RPAS-AI 2016, September 22–23, 2016, Innopolis, Republic of Tatarstan, Russia)]. Moscow: Iz-vo “Pero”; 2016; p. 137–146.
- Karpov V., Migalev A., Moscowsky A.,
Rovbo M., Vorobiev V. Multi-robot exploration and mapping based on thesubdefinite models. In: Ronzhin A., Rigol G., Meshcheryakov R. (editors). Interactive collaborative robotics. Springer International Publishing; 2016; p. 143–152, https://doi.org/10.1007/978-3-319-43955-6_18. - Davison A.J., Reid I.D., Molton N.D., Stasse O. MonoSLAM: real-time single camera SLAM. IEEE Trans Pattern Anal Mach Intell 2007; 29(6): 1052–1067, https://doi.org/10.1109/tpami.2007.1049.
- Engel J., Schöps T., Cremers D. LSD-SLAM: large-scale direct monocular SLAM. In: Fleet D., Pajdla T., Schiele B., Tuytelaars T. (editors). Computer vision — ECCV 2014. ECCV 2014. Lecture Notes in Computer Science. Springer, Cham; 2014; p. 834–849, https://doi.org/10.1007/978-3-319-10605-2_54.
- Mur-Artal R., Montiel J.M.M., Tardos J.D. ORB-SLAM: a versatile and accurate monocular SLAM system. EEE Trans Robot 2015; 31(5): 1147–1163, https://doi.org/10.1109/tro.2015.2463671.
- Milford M.J., Wyeth G.F., Prasser D. RatSLAM: a hippocampal model for simultaneous localization and mapping. IEEE Int Conf Robot Autom 2004; 1: 403–408, https://doi.org/10.1109/robot.2004.1307183.