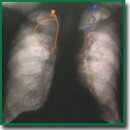
PhthisisBioMed Artificial Medical Intelligence: Software for Automated Analysis of Digital Chest X-ray/Fluorograms
The scope of diagnostic medical examinations increases from year to year causing a reasonable desire to develop and implement new technologies to diagnostics and medical data analysis. Artificial intelligence (AI) algorithms became one of the most promising solutions to this problem and proved themselves in the course of mass practical application. During the three-year Moscow experiment started in 2020, the possibility was achieved to develop methodologies of AI use and to successfully implement it into the regional level healthcare system.
In this article, the authors share their experience in developing a medical AI service using the example of PhthisisBioMed AI service and the results of its application in real clinical activities environment. This AI service has shown its quality and reliability confirmed by technological monitoring.
Clinical trials of PhthisisBioMed AI service were conducted on a specially prepared verified data set (n=1536) considering epidemiological indicators of the thoracic organs major diseases prevalence. The mean sensitivity of the service was 0.975 (95% CI: 0.966–0.984).
PhthisisBioMed medical AI service is registered as a medical device (medical device registration certificate No.RZN 2022/17406 dated May 31, 2022) and is actively used in the Russian Federation as a diagnostic tool to reduce the burden on radiologists and to accelerate the process of medical report obtaining.
- Federal State Statistics Service (Rosstat). Zdravookhranenie [Healthcare]. URL: https://rosstat.gov.ru/folder/13721.
- Gogoberidze Yu.T., Klassen V.I., Natenzon M.Ya., Prosvirkin I.A., Safin A.A. Osobennosti implementatsii sistem iskusstvennogo intellekta v zadache analiza dvukhmernykh radiologicheskikh izobrazheniy. V kn.: Matematicheskie metody raspoznavaniya obrazov [Features of the implementation of artificial intelligence systems in the problem of analyzing two-dimensional radiological images. In: Mathematical methods of pattern recognition]. Moscow: Rossiyskaya akademiya nauk; 2019; p. 307–308.
- Huang G., Liu., van der Maaten L., Weinberger K.Q. Densely connected convolutional networks. arXiv; 2018, https://doi.org/10.48550/arXiv.1608.06993.
- Hwang E.J., Park S., Jin K.N., Kim J.I., Choi S.Y., Lee J.H., Goo J.M., Aum J., Yim J.J., Cohen J.G., Ferretti G.R., Park C.M.; DLAD Development and Evaluation Group. Development and validation of a deep learning-based automated detection algorithm for major thoracic diseases on chest radiographs. JAMA Netw Open 2019; 2(3): e191095, https://doi.org/10.1001/jamanetworkopen.2019.1095.
- Morozov S.P., Vladzimirskiy A.V., Klyashtornyy V.G., Andreychenko A.E., Kul’berg N.S., Gombolevskiy V.A., Sergunova K.A. Klinicheskie ispytaniya programmnogo obespecheniya na osnove intellektual’nykh tekhnologiy (luchevaya diagnostika). Seriya “Luchshie praktiki luchevoy i instrumental’noy diagnostiki”. Vyp. 57 [Clinical trials of software based on intelligent technologies (radiology). Series “Best practices of radiological and instrumental diagnostics”. Issue 57]. Moscow; 2019.
- Rebrova O.Yu., Gusev A.V. Sample size calculation for clinical trials of medical decision support systems with binary outcome. Sovremennye tehnologii v medicine 2022; 14(3): 6, https://doi.org/10.17691/stm2022.14.3.01.
- Sun X., Xu W. Fast implementation of DeLong’s algorithm for comparing the areas under correlated receiver operating characteristic curves. IEEE Signal Process Lett 2014; 21(11): 1389–1393, https://doi.org/10.1109/lsp.2014.2337313.
- Scientific and Practical Clinical Center for Diagnostics and Telemedicine Technologies of the Moscow Department of Health. Eksperiment po ispol’zovaniy innovatsionnykh tekhnologiy v oblasti komp’yuternogo zreniya dlya analiza meditsinskikh izobrazheniy i dal’neyshego primeneniya v sisteme zdravookhraneniya Moskvy [An experiment on the use of innovative computer vision technologies for medical image analysis and subsequent applicability in the healthcare system of Moscow]. URL: https://mosmed.ai.
- Prikaz Departamenta zdravookhraneniya goroda Moskvy ot 19.02.2020 No.142 “Ob utverzhdenii Poryadka i usloviy provedeniya eksperimenta po ispol’zovaniyu innovatsionnykh tekhnologiy v oblasti komp’yuternogo zreniya dlya analiza meditsinskikh izobrazheniy i dal’neyshego primeneniya v sisteme zdravookhraneniya goroda Moskvy” [Order of the Moscow Department of Health dated February 19, 2020 No.142 “On approval of the Procedure and conditions for conducting an experiment on the use of innovative computer vision technologies for medical image analysis and subsequent applicability in the healthcare system of Moscow”].
- LAVAL medical systems. Edinyy radiologicheskiy informatsionnyy servis [Unified Radiological Information Service]. URL: http://lvlmed.ru/eris/.
- DICOM® — Digital Imaging and Communications in Medicine. Current Edition. URL: https://www.dicomstandard.org/current/.
- Apache Software Foundation. Apache Kafka. URL: https://kafka.apache.org/.
- Korevaar D.A., Gopalakrishna G., Cohen J.F., Bossuyt P.M. Targeted test evaluation: a framework for designing diagnostic accuracy studies with clear study hypotheses. Diagn Progn Res 2019; 3: 22, https://doi.org/10.1186/s41512-019-0069-2.
- Bossuyt P.M., Reitsma J.B., Bruns D.E., Gatsonis C.A., Glasziou P.P., Irwig L., Lijmer J.G., Moher D., Rennie D., de Vet H.C., Kressel H.Y., Rifai N., Golub R.M., Altman D.G., Hooft L., Korevaar D.A., Cohen J.F.; STARD Group. STARD 2015: an updated list of essential items for Reporting Diagnostic Accuracy Studies. Radiology 2015; 277(3): 826–832, https://doi.org/10.1148/radiol.2015151516.
- Zinchenko V.V., Akhmad E.S., Zayunchkovsky S.Yu., Sharova D.E., Vladzimirsky A.V., Morozov S.P. Methodology for conducting clinical trials of medical software with artificial intelligence. Meditsinskaya fizika 2022; 1: 32.
- Morozov S.P., Vladzimirskiy A.V., Andreychenko A.E., Akhmad E.S., Blokhin I.A., Gombolevskiy V.A., Zinchenko V.V., Kul’berg N.S., Novik V.P., Pavlov N.A. Reglament podgotovki naborov dannykh s opisaniem podkhodov k formirovaniyu reprezentativnoy vyborki dannykh. Chast’ 1: metodicheskie rekomendatsii. Seriya “Luchshie praktiki luchevoy i instrumental’noy diagnostiki”. Vyp. 103 [Regulations for the preparation of data sets with a description of approaches to the formation of a representative sample of data. Part 1: guidelines. Series “Best practices of radiological and instrumental diagnostics”. Issue 103]. Moscow: GBUZ “NPKTs DiT DZM”; 2022.
- Narkevich A.N., Vinogradov K.A. Methods for determining the minimum required sample size in medical research. Social’nye aspekty zdorov’a naselenia 2019; 65(6): 10.