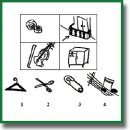
Individual Stable Patterns of Human Brain Rhythms as a Reflection of Mental Processes
Here, we attempt to summarize our research conducted for more than twenty years. Back in 1997, we were the first to publish the data indicating that the type of cognitive task (spatial or arithmetic) performed by a subject can be identified with a reliability of 70 to 98% (dependent on a subject) by analyzing the EEG spectra and using an artificial neural network. Further research led us to the understanding that any sustainable mental activity was accompanied by characteristic rhythmic EEG patterns. Individual EEG rhythms (that in totality form a pattern) differ in their frequency and topography. Cognitive patterns of EEG rhythms have a number of fundamental characteristics. They are highly specific and stable in each individual and persist for years (slowly changing); they are also highly specific for each type of cognitive activity.
Later it was found that the arising patterns of brain rhythms were not only different for different types of cognitive tasks but also interrelated with each other in the way similar to the inter-relations of psychological characteristics of the tasks. Based on this finding, we have developed a method for creating a map of a person’s cognitive space. It turned out that, by using this method, one can draw maps of a human sensory-emotional space.
In experiments with the presentation of equivalent audial and visual tasks, we found that the EEG rhythm patterns reflected the very nature of mental acts, and not processes of sensory perception.
The developed methods for distinguishing between different mental states and for creating mental space maps have found their practical use including that in medicine. In mental illnesses, the thinking ability is impaired, which is manifested in changes in the cognitive rhythmic patterns of the EEG. When consciousness is depressed, the emotional-sensory spaces reflect rather the physical properties (and not the emotional content) of the stimuli presented to patients.
The accumulated knowledge made it possible to develop a device prototype (called “cognovisor”), which allows for real-time tracking of one’s thinking process and displaying it on a map of the individual cognitive space.
- Kamitani Y., Tong F. Decoding the visual and subjective contents of the human brain. Nat Neurosci 2005; 8(5): 679–685, https://doi.org/10.1038/nn1444.
- Ivanitsky G.A. Recognition of the task type in the process of its mental solving by a few-second EEG record using the learned classifier. Zhurnal vysshei nervnoi deyatelnosti imeni I.P. Pavlova 1997; 47(4): 743–747.
- Ivanitsky G.A., Nikolaev A.R., Ivanitsky A.M. The use of artificial neural networks for recognition of types of thinking by EEG. Aviakosmicheskaya i ekologicheskaya meditsina 1997; 31(6): 23–28.
- Haxby J.V., Gobbini M.I., Furey M.L., Ishai A., Schouten J.L., Pietrini P. Distributed and overlapping representations of faces and objects in ventral temporal cortex. Science 2001; 293(5539): 2425–2430, https://doi.org/10.1126/science.1063736.
- Pietrini P., Furey M.L., Ricciardi E., Gobbini M.I., Wu W.H., Cohen L., Guazzelli M., Haxby J.V. Beyond sensory images: object-based representation in the human ventral pathway. Proc Natl Acad Sci U S A 2004; 101(15): 5658–5663, https://doi.org/10.1073/pnas.0400707101.
- Shinkareva S.V., Mason R.A., Malave V.L., Wang W., Mitchell T.M., Just M.A. Using fMRI brain activation to identify cognitive states associated with
perception of tools and dwellings. PLoS One 2008; 3(1): e1394, https://doi.org/10.1371/journal.pone.0001394. - Nishimoto S., Vu A.T., Naselaris T., Benjamini Y., Yu B., Gallant J.L. Reconstructing visual experiences from brain activity evoked by natural movies. Curr Biol 2011; 21(19): 1641–1646, https://doi.org/10.1016/j.cub.2011.08.031.
- Mitchell T.M., Hutchinson R., Niculescu R.S., Pereira F., Wang X., Just M., Newman S. Learning to decode cognitive states from brain images. Machine Learning 2004; 57(1/2): 145–175, https://doi.org/10.1023/b:mach.0000035475.85309.1b.
- Mitchell T.M., Shinkareva S.V., Carlson A., Chang K.M., Malave V.L., Mason R.A., Just M.A. Predicting human brain activity associated with the meanings of nouns. Science 2008; 320(5880): 1191–1195, https://doi.org/10.1126/science.1152876.
- Wolpaw J.R., McFarland D.J., Vaughan T.M. Brain-computer interface research at the Wadsworth Center. IEEE Trans Rehabil Eng 2000; 8(2): 222–226, https://doi.org/10.1109/86.847823.
- Peters B.O., Pfurtscheller G., Flyvbjerg H. Automatic differentiation of multichannel EEG signals. IEEE Trans Biomed Eng 2001; 48(1): 111–116, https://doi.org/10.1109/10.900270.
- Bobrov P., Frolov A., Cantor C., Fedulova I., Bakhnyan M., Zhavoronkov A. Brain-computer interface based on
generation of visual images. PLoS One 2011; 6(6): e20674, https://doi.org/10.1371/journal.pone.0020674. - Bobrov P.D., Korshakov A.V., Roschin V.Yu., Frolov A.A. Bayesian classifier for brain–computer interface based on mental representation of movements. Zhurnal vysshei nervnoi deyatelnosti imeni I.P. Pavlova 2012; 62(1): 89–99.
- Del R. Millan J., Mourino J., Franze M., Cincotti F., Varsta M., Heikkonen J., Babiloni F. A local neural classifier for the recognition of EEG patterns associated with mental tasks. IEEE Trans Neural Netw 2002; 13(3): 678–686, https://doi.org/10.1109/tnn.2002.1000132.
- Wang Q.,
Sourina O. Real-time mental arithmetic task recognition from EEG signals. IEEE Trans Neural Syst Rehabil Eng 2013; 21(2): 225–232, https://doi.org/10.1109/tnsre.2012.2236576. - Bardin K.V. Problema porogov chuvstvitel’nosti i psikhofizicheskie metody [The problem of sensitivity thresholds and psychophysical methods]. Moscow: Nauka; 1976.
- Izmaylov Ch.A. Sfericheskaya model’ tsvetorazlicheniya [Spherical model of color discrimination]. Moscow: Izd-vo MGU, 1980.
- Izmaylov Ch.A., Sokolov E.N., Chernorizov A.M. Psikhofiziologiya tsvetovogo zreniya [Psychophysiology of color vision]. Moscow: Izd-vo MGU, 1989.
- Zabrodin Yu.M., Lebedev A.N. Psikhofiziologiya i psikhofizika [Psychophysiology and psychophysics]. Moscow: Nauka, 1977.
- Lomov B.F., Ivanitskii A.M. Connection between psychology and physiology in the investigation of perception. Human Physiology 1977; 3(6): 753–760.
- Terekhina A.Yu. Multidimensional scaling in psychology. Psikhologicheskiy zhurnal 1983; 4(1): 77–88.
- Huth A.G., Nishimoto S., Vu A.T., Gallant J.L. A continuous semantic space describes the representation of thousands of object and action categories across the human brain. Neuron 2012; 76(6): 1210–1224, https://doi.org/10.1016/j.neuron.2012.10.014.
- Ivanitskiy G.A. Raspoznavanie
tipa reshaemoy zadachi po neskol’kim sekundam EEG spomoshch’yu obuchaemogo klassifikatora . Dis. …dokt . biol. nauk [Recognizing the type of the task being solved by several seconds of EEG using a taught classifier. DSc Dissertation]. Moscow; 2007. - Rumelhart D.E., McClelland J.L., and the PDP Research Group. Parallel distributed processing. Cambridge, Mass.: MIT Press; 1986.
- Roik A.O., Ivanitsky G.A. Neurophysiological model of cognitive space. Zhurnal vysshei nervnoi deyatelnosti imeni I.P. Pavlova 2011; 61(6): 688–696.
- Roik A.O., Ivanitsky G.A., Ivanitsky A.M. Human cognitive space: coincidence of the models, built on base of EEG rhythms and psychometric measurements. Rossiiskii fiziologicheskii zhurnal imeni I.M. Sechenova 2012; 98(11): 1314–1328.
- Sammon J.W. A nonlinear mapping for data structure analysis. IEEE Trans Comput 1969; C-18(5): 401–409, https://doi.org/10.1109/t-c.1969.222678.
- Atanov M.S., Ivanitsky G.A., Ivanitsky A.M. Cognitive
brain–computer interface and probable aspects of its practical application. Human Physiology 2016; 42(3): 235–240, https://doi.org/10.1134/s0362119716030038. - Tarotin I.V., Atanov M.S., Ivanitsky G.A. A model for human cognitive activity monitoring in real time (“cognovisor”). Zhurnal vysshei nervnoi deyatelnosti imeni I.P. Pavlova 2017; 67(4): 493–503, https://doi.org/10.7868/s0044467717040116.
- Nikolaev A.R., Ivanitskiy G.A., Ivanitskiy A.М. Reproducible patterns of EEG alpha-rhythm in solving psychological tasks. Fiziologiia cheloveka 1998; 24(3): 1–8.
- Ivanitsky G.A., Naumov R.A., Ivanitsky A.M. The technology for the recognition of the mental thinking operations type using EEG patterns. Tekhnologii zhivykh sistem 2007; 4(5–6): 20–29.
- Atanov M.S., Ivanitsky G.A. Optimizatsiya algoritma raspoznavaniya tipa tekushchey myslitel’noy deyatel’nosti na osnove dannykh EEG. V kn.: XVII Vserossiyskaya nauchno-tekhnicheskaya konferentsiya “Neyroinformatika-2015”. Chast’ 1 [Optimization of current type of cognitive activity recognition based on EEG data. In: XVII All-Russian scientific and technical conference “Neuroinformatics-2015”. Part 1]. Moscow: NIYaU MIFI; 2015; p. 88–96.
- Roik A.O. Kodirovanie osobennostey kognitivnoy deyatel’nosti v ritmicheskom risunke EEG. Avtoref. dis. … kand. biol. nauk [Encoding of cognitive activity specifics in the EEG rhythmic pattern. PhD Thesis]. Moscow; 2012.
- Ilyuchenok I.R. EEG frequency differences during perception of positive, negative, and neutral words. Zhurnal vysshei nervnoi deyatelnosti imeni I.P. Pavlova 1996; 46(3): 457–468.
- Ilyutchenok I.R., Savostyanov A.N., Valeev R.G. EEG spectral dynamics in the theta and alpha bands during a negative emotional reaction. Zhurnal vysshei nervnoi deyatelnosti imeni I.P. Pavlova 2001; 51(5): 563–571.
- Kostyunina M.B. Human EEG during mental reproduction of emotionally significant events. Zhurnal vysshei nervnoi deyatelnosti imeni I.P. Pavlova 1998; 48(2): 213–221.
- Kolbeneva M.G., Aleksandrov Yu.I. Organy chuvstv, emotsii i prilagatel’nye russkogo yazyka. V kn.: Lingvo-psikhologicheskiy slovar’. Yazyki slavyanskikh kul’tur [The sense organs, emotions and adjectives of the Russian language. In: Lingvo-psychological dictionary. Languages of Slavic cultures]. Moscow; 2010.
- Kolbeneva M.G., Alexandrov Y.I. Mental reactivation and pleasantness judgment of experience related to vision, hearing, skin sensations, taste
and olfaction. PLoS One 2016; 11(7): e0159036, https://doi.org/10.1371/journal.pone.0159036. - Portnova G.V., Ivanitsky G.A., Sharova E.V., Ivanitsky A.M. The re-arrangement of brain rhythms in response to emotionally significant stimuli in healthy adults, children and patients in coma. Tekhnologii zhivykh sistem 2012; 9(5): 3–13.
- Portnova G.V., Gladun K.V., Sharova E.V., Ivanitsky A.M. Changes of EEG power spectrum in response to the emotional auditory stimuli in patients in acute and recovery stages of TBI (traumatic brain injury). Zhurnal vysshei nervnoi deyatelnosti imeni I.P. Pavlova 2013; 63(6): 753, https://doi.org/10.7868/s0044467713060142.
- Portnova G., Stebakova D., Ivanitsky G. The EEG-based emotion classification in tactile, olfactory, acoustic and visual modalities. In: Proceedings of the 2nd International conference on computer-human interaction research and applications. Vol. 1: CHIRA. SCITEPRESS — Science and Technology Publications; 2018; p. 93–99, https://doi.org/10.5220/0006892100930099.
- Baklushev M.E., Ivanitsky G.A., Atanov M.S., Ivanitsky A.M. High variability of rhythmic EEG patterns, intrinsic for different type of thinking in schizophrenia patients. Zhurnal vysshei nervnoi deyatelnosti imeni I.P. Pavlova 2016; 66(5): 579–58, https://doi.org/10.7868/s0044467716050038.
- Baklushev M.E. Nestabil’nost’ ritmicheskikh kharakteristik EEG pri myshlenii u bol’nykh shizofreniey. Dis. … kand. med. nauk [Instability of EEG rhythmic characteristics during thinking in patients with schizophrenia. PhD Dissertation]. Moscow; 2018.
- Baklushev M.E., Ivanitsky G.A., Ivanitsky A.M. Violation of assessing the salience of information in schizophrenia. Uspekhi fiziologicheskikh nauk 2016; 47(1): 34–47.
- Portnova G.V., Atanov M.S. EEG of patients in coma after traumatic brain injury reflects physical parameters of auditory stimulation but not its emotional content. Brain Injury 2018; 33(3): 370–376, https://doi.org/10.1080/02699052.2018.1553310.
- Fink A., Grabner R.H., Neuper C., Neubauer A.C. EEG alpha band dissociation with increasing task demands. Brain Res Cogn Brain Res 2005; 24(2): 252–259, https://doi.org/10.1016/j.cogbrainres.2005.02.002.
- Tarotin I.V., Ivanitsky G.A. Central EEG rhythm associated with movement and EEG rhythm associated with spatial reasoning: are they homologous? Zhurnal vysshei nervnoi deyatelnosti imeni I.P. Pavlova 2014; 64(6): 615–626.
- Lega B.C., Jacobs J., Kahana M. Human hippocampal theta oscillations and the formation of episodic memories. Hippocampus 2011; 22(4): 748–761, https://doi.org/10.1002/hipo.20937.
- Ekstrom A.D., Caplan J.B., Ho E., Shattuck K., Fried I., Kahana M.J. Human hippocampal theta activity during virtual navigation. Hippocampus 2005; 15(7): 881–889, https://doi.org/10.1002/hipo.20109.