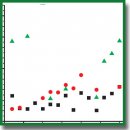
Computer-Aided Detection of Respiratory Sounds in Bronchial Asthma Patients Based on Machine Learning Method
The aim of the study is to develop a method for detection of pathological respiratory sound, caused by bronchial asthma, with the aid of machine learning techniques.
Materials and Methods. To build and train neural networks, we used the records of respiratory sounds of bronchial asthma patients at different stages of the disease (n=951) aged from several months to 47 years old and healthy volunteers (n=167). The sounds were recorded with calm breathing at four points: at the oral cavity, above the trachea, on the chest (second intercostal space on the right side), and at a point on the back.
Results. The method developed for computer-aided detection of respiratory sounds allows to diagnose sounds typical for bronchial asthma in 89.4% of cases with 89.3% sensitivity and 86.0% specificity regardless of sex and age of the patients, stage of the disease, and the point of sound recording.
- Boulet L.P., Reddel H.K., Bateman E., Pedersen S., FitzGerald J.M., O’Byrne P.M. The global initiative for asthma (GINA): 25 years later. Eur Respir J 2019; 54(2): 1900598, https://doi.org/10.1183/13993003.00598-2019.
- Bahoura M., Lu X. Separation of crackles from vesicular sounds using wavelet packet transform. Proc IEEE Int Conf Acoust Speech Signal Process 2006; 2: 1076–1079.
- Brand P.L.P., Baraldi E., Bisgaard H., Boner A.L., Castro-Rodriguez J.A., Custovic A., de Blic J., de Jongste J.C., Eber E., Everard M.L., Frey U., Gappa M., Garcia-Marcos L., Grigg J., Lenney W., Le Souëf P., McKenzie S., Merkus P.J.F.M., Midulla F., Paton J.Y., Piacentini G., Pohunek P., Rossi G.A., Seddon P., Silverman M., Sly P.D., Stick S., Valiulis A., van Aalderen W.M.C., Wildhaber J.H., Wennergren G., Wilson N., Zivkovic Z., Bush A. Definition, assessment and treatment of wheezing disorders in preschool children: an evidence-based approach. Eur Respir J 2008; 32(4): 1096–1110, https://doi.org/10.1183/09031936.00002108.
- Furman Е.G., Yakovleva Е.V., Malinin S.V., Furman G., Sokolovsky V. Computer-assisted assay of respiratory sounds of children suffering from bronchial asthma. Sovremennye tehnologii v medicine 2014; 6(1): 83.
- Furman E.G., Rocheva E.V., Malinin S.V., Furman G.B., Sokolovsky V.L. Comparative efficiency of computerized analysis of respiratory noise spectrum energetic characteristic in three points for diagnosis of bronchoobstructive syndrome in pediatric bronchial asthma. Permskij medicinskij zurnal 2015; 32(5): 77–87.
- Mhetre R., Bagal U.R. Respiratory sound analysis for diagnostic information. IOSR J Electr Electron Eng 2014; 9(5): 42–46.
- Furman E., Charushin A., Eirikh E., Furman G., Sokolovsky V., Malinin S., Sheludko V., Polyanskaya D., Kalinina N., Shtivelman D. Capabilities of computer analysis of breath sounds in patients with COVID-19. Permskij medicinskij zurnal 2021; 38(3): 97–109.
- Chekhovych M.G., Poreva A.S., Timofeyev V.I., Henaff P. Using of the machine learning methods to identify bronchopulmonary system diseases with the use of lung sounds. Visnyk NTUU KPI Seriia — Radioteknika Radioaparaturalobuduvannia 2018; 73: 55–62, https://doi.org/10.20535/radap.2018.73.55-62.
- Sovijarvi A.R.A., Malmberg L.P., Charbonneau G., Vanderschoot J., Dalmasso F., Sacco C., Rossi M., Earis J.R. Characteristics of breath sounds and adventitious respiratory sounds. Eur Respir Rev 2000; 10(77): 591–596.
- Sovijarvi A.R.A., Dalmasso F., Vanderschoot J., Malmberg L.P., Righini G., Stoneman S.A.T. Definition of terms for applications of respiratory sounds. Eur Respir Rev 2000; 10(77): 597–610.
- Furman Е.G., Malinin S.V., Furman G., Meerovich V., Sokolovsky V., Rocheva Е. Respiratory sound analysis for bronchial asthma diagnostics. IOSR J Eng 2020; 10(1): 53–59.
- Holmes M.S., D’arcy S., Costello R.W., Reilly R.B. Acoustic analysis of inhaler sounds community-dwellin asthmatic patients for automatic assessment of adherence. IEEE J Transl Eng Health Med 2014; 2: 2700210, https://doi.org/10.1109/jtehm.2014.2310480.
- Oud M., Dooijes E.H., van der Zee J.S. Asthmatic airways obstruction assessment based on detailed analysis of respiratory sound spectra. IEEE Trans Biomed Eng 2000; 47(11): 1450–1455, https://doi.org/10.1109/10.880096.
- Tomita K., Nagao R., Touge H., Ikeuchi T., Sano H., Yamasaki A., Tohda Y. Deep learning facilitates the diagnosis of adult asthma. Allergol Int 2019; 68(4): 456–461, https://doi.org/10.1016/j.alit.2019.04.010.
- Shakhgeldyan K.J., Geltser B.I., Kurpatov I.G. Machine learning methods for differential diagnosis of respiratory diseases. Trudy XX Mezhdunarodnogo kongressa “Informatsionnye tekhnologii v meditsine” [Proceedings of the XX International Congress “Information Technologies in Medicine”]. Moscow; 2019; p. 10–13.
- Robles-Rubio C.A., Bertolizio G., Brown K.A., Kearney R.E. Scoring tools for the analysis of infant respiratory inductive plethysmography signals. PLoS One 2015; 10(7): e0134182, https://doi.org/10.1371/journal.pone.0134182.
- Azar A.T. Fast neural network learning algorithms for medical applications. Neural Comput Appl 2013; 23: 1019–1034, https://doi.org/10.1007/s00521-012-1026-y.
- Dwivedi A.K. Artificial neural network model for effective cancer classification using microarray gene expression data. Neural Comput Appl 2018; 29: 1545–1554, https://doi.org/10.1007/s00521-016-2701-1.
- Gupta N., Gupta D., Khanna A., Filho P.P.R., de Albuquerque V.H.C. Evolutionary algorithms for automatic lung disease detection. Measurement 2019; 140: 590–608, https://doi.org/10.1016/j.measurement.2019.02.042.
- Swaminathan S., Qirko K., Smith T., Corcoran E., Wysham N.G., Bazaz G., Kappel G., Gerber A.N. A machine learning approach to triaging patients with chronic obstructive pulmonary disease. PLoS One 2017; 12(11): e0188532, https://doi.org/10.1371/journal.pone.0188532.
- Poreva A.S., Vaityshyn V.I., Karplyuk Ye.S. Machine learning methods for the study of the lungsounds signals. MicrosystElectronAcoust 2017; 22(6): 41–47, https://doi.org/10.20535/2523-4455.2017.22.6.108829.
- Yadav A., Dutta M.K., Prinosil J. Machine learning based automatic classification of respiratory signals using wavelet transform. In: 2020 43rd International Conference on Telecommunications and Signal Processing (TSP). Milan; 2020; p. 545–549, https://doi.org/10.1109/tsp49548.2020.9163565.
- Spathis D., Vlamos P. Diagnosing asthma and chronic obstructive pulmonary disease with machine learning. Health Informatics J 2019; 25(3): 811–827, https://doi.org/10.1177/1460458217723169.
- Messinger A.I., Bui N., Wagner B.D., Szefler S.J., Vu T., Deterding R.R. Novel pediatric-automated respiratory score using physiologic data and machine learning in asthma. Pediatr Pulmonol 2019; 54(8): 1149–1155, https://doi.org/10.1002/ppul.24342.
- Elmarakeby A.H., Hwang J., Arefeh R., Crowdis J., Gang S., Liu D., AlDubayan S.H., Salari K., Kregel S., Richter C., Arnoff T.E., Park J., Hahn W.C., Van Allen E.M. Biologically informed deep neural network for prostate cancer discovery. Nature 2021; 598: 348–352, https://doi.org/10.1038/s41586-021-03922-4.
- Shrikumar A., Greeside P., Kundaje A. Learning important features through propagating activation differences. Proc Mach Learn Res 2017; 70: 3145–3153, https://doi.org/10.48550/arxiv.1704.02685.
- Murdoch W.J., Singh C., Kumbier K., Abbasi-Asl R., Yu B. Definitions, methods, and applications in interpretable machine learning. Proc Natl Acad Sci USA; 2019; 116(44): 22071–22080, https://doi.org/10.1073/pnas.1900654116.
- Russian Respiratory Society. Pediatric Respiratory Society. Federation of pediatricians of the CIS countries. Natsional’naya programma “Bronkhial’naya astma u detey. Strategiya lecheniya i profilaktika” [National program “Bronchial asthma in children. Treatment strategy and prevention”]. Moscow; 2012.
- Bronkhial’naya astma. Klinicheskie rekomendatsii [Bronchial asthma. Clinical recommendations]. Chuchalin A.G. (editor). Moscow; 2021; 118 p.
- Hastie T., Tibshirani R., Friedman J.H. The elements of statistical learning: data mining, inference, and prediction. Second edition. New York: Springer; 2009.
- Kiang M.Y. A comparative assessment of classification methods. Decis Support Syst 2003; 35(4): 441–454, https://doi.org/10.1016/s0167-9236(02)00110-0.
- Sharma G., Umapathy K., Krishnan S. Trends in audio signal feature extraction methods. Appl Acoust 2020; 158: 107020, https://doi.org/10.1016/j.apacoust.2019.107020.
- Boulesteix A.L., Janitza S., Kruppa J., König I.R. Overview of random forest methodology and practical guidance with emphasis on computational biology and bioinformatics. WIREs Data Mining Knowl Discov 2012; 2(6): 493–507, https://doi.org/10.1002/widm.1072.
- Rokach L., Maimon O. Decision trees. In: Data mining and knowledge discovery handbook. Boston, MA: Springer; 2005; p. 165–192, https://doi.org/10.1007/0-387-25465-x_9.
- Du K.L. Clustering: a neural network approach. Neural Netw 2010; 23(1): 89–107, https://doi.org/10.1016/j.neunet.2009.08.007.
- Nitze I., Schulthess U., Asche H. Comparison of machine learning algorithms random forest, artificial neural network and support vector machine to maximum likelihood for supervised crop type classification. In: Proceedings of the 4th GEOBIA. Rio de Janeiro; 2012; p. 35.
- Buccio E.D., Li Q., Melucci M., Tiwari P. Binary classification model inspired from quantum detection theory. In: Proceedings of the ACM SIGIR International Conference on Theory of Information Retrieval (ICTIR). New York; 2018; p. 187–190.
- Doshi К. Audio deep learning made simple: sound classification, step-by-step. Mar 18, 2021. URL: https://towardsdatascience.com/audio-deep- learning-made-simple-sound-classification- step-by-step-cebc936bbe5.
- van de Berge M., ten Hacken N.H.T., van der Wiel E., Postma D.S. Treatment of the bronchial tree from beginning to end: targeting small airway inflammation in asthma. Allergy 2013; 68(1): 16–26, https://doi.org/10.1111/all.12062.
- Furman E.G., Koryukina I.P. Bronkhial’naya astma u detey: markery vospaleniya i sostoyanie funktsii vneshnego dykhaniya [Bronchial asthma in children: markers of inflammation and the state of respiratory function]. Perm; 2010; 183 p.
- Ovsyannikov D.Yu., Furman E.G., Eliseeva T.I. Bronkhial’naya astma u detey [Bronchial asthma in children]. Moscow: Rossiyskiy universitet druzhby narodov; 2019; 211 p.
- Schneider A., Gindner L., Tilemann L., Schermer T., Dinant G.J., Joachim Meyer F., Szecsenyi J. Diagnostic accuracy of spirometry in primary care. BMC Pulm Med 2009; 9: 31, https://doi.org/10.1186/1471-2466-9-31.
- Valadares M.A., Santos I.N., Melo E.V., da Silva Â.M., Archanjo P.T., Nepomusceno E.C., Porto R.L., Gurgel R.Q., Brito L.S., Almeida M.L.D. Spirometry with FEV0.75 increases the sensitivity for the diagnosis of obstructive disorder in children of asthmatic mothers. J Aller Ther 2013; S2: 6, https://doi.org/10.4172/2155-6121.s2-006.
- Künzli N., Stutz E.Z., Perruchoud A.P., Brändli O., Tschopp J.M., Bolognini G., Karrer W., Schindler C., Ackermann-Liebrich U., Leuenberger P. Peak flow variability in the SAPALDIA study and its validity in screening for asthma-related conditions. The SPALDIA Team. Am J Respir Crit Care Med 1999; 160(2): 427–434, https://doi.org/10.1164/ajrccm.160.2.9807008.