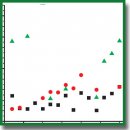
Компьютерная детекция респираторных шумов при бронхиальной астме на основе метода машинного обучения
Цель исследования — с помощью технологии машинного обучения разработать метод детекции патологических респираторных шумов, возникающих при бронхиальной астме.
Материалы и методы. Для построения и обучения нейронных сетей использовали записи респираторных шумов больных бронхиальной астмой в разной стадии заболевания (n=951) в возрасте от нескольких месяцев до 47 лет и здоровых добровольцев (n=167). Записи проводили при спокойном дыхании в четырех точках: в ротовой полости, над трахеей, на груди (второе межреберье с правой стороны) и в точке на спине.
Результаты. Разработанный метод компьютерной детекции респираторных шумов позволяет диагностировать характерные для бронхиальной астмы шумы в 89,4% случаев с чувствительностью 89,3% и специфичностью 86,0% независимо от пола и возраста пациента, стадии заболевания, а также точки записи шумов.
- Boulet L.P., Reddel H.K., Bateman E., Pedersen S., FitzGerald J.M., O’Byrne P.M. The global initiative for asthma (GINA): 25 years later. Eur Respir J 2019; 54(2): 1900598, https://doi.org/10.1183/13993003.00598-2019.
- Bahoura M., Lu X. Separation of crackles from vesicular sounds using wavelet packet transform. Proc IEEE Int Conf Acoust Speech Signal Process 2006; 2: 1076–1079.
- Brand P.L.P., Baraldi E., Bisgaard H., Boner A.L., Castro-Rodriguez J.A., Custovic A., de Blic J., de Jongste J.C., Eber E., Everard M.L., Frey U., Gappa M., Garcia-Marcos L., Grigg J., Lenney W., Le Souëf P., McKenzie S., Merkus P.J.F.M., Midulla F., Paton J.Y., Piacentini G., Pohunek P., Rossi G.A., Seddon P., Silverman M., Sly P.D., Stick S., Valiulis A., van Aalderen W.M.C., Wildhaber J.H., Wennergren G., Wilson N., Zivkovic Z., Bush A. Definition, assessment and treatment of wheezing disorders in preschool children: an evidence-based approach. Eur Respir J 2008; 32(4): 1096–1110, https://doi.org/10.1183/09031936.00002108.
- Furman Е.G., Yakovleva Е.V., Malinin S.V., Furman G., Sokolovsky V. Computer-assisted assay of respiratory sounds of children suffering from bronchial asthma. Sovremennye tehnologii v medicine 2014; 6(1): 83.
- Фурман Е.Г., Рочева Е.В., Малинин С.В., Фурман Г.Б., Соколовский В.Л. Сравнительная эффективность компьютерного анализа энергетической характеристики спектра респираторных шумов в трех точках для диагностики бронхобструктивного синдрома при бронхиальной астме у детей. Пермский медицинский журнал 2015; 32(5): 77–87.
- Mhetre R., Bagal U.R. Respiratory sound analysis for diagnostic information. IOSR J Electr Electron Eng 2014; 9(5): 42–46.
- Фурман Е.Г., Чарушин А., Эйрих Е., Фурман Г., Соколовский В., Малинин С., Шелудько В., Полянская Д., Калинина Н., Штивельман Д. Возможности компьютерного анализа дыхательных шумов у пациентов с заболеванием COVID-19. Пермский медицинский журнал 2021; 38(3): 97–109.
- Chekhovych M.G., Poreva A.S., Timofeyev V.I., Henaff P. Using of the machine learning methods to identify bronchopulmonary system diseases with the use of lung sounds. Visnyk NTUU KPI Seriia — Radioteknika Radioaparaturalobuduvannia 2018; 73: 55–62, https://doi.org/10.20535/radap.2018.73.55-62.
- Sovijarvi A.R.A., Malmberg L.P., Charbonneau G., Vanderschoot J., Dalmasso F., Sacco C., Rossi M., Earis J.R. Characteristics of breath sounds and adventitious respiratory sounds. Eur Respir Rev 2000; 10(77): 591–596.
- Sovijarvi A.R.A., Dalmasso F., Vanderschoot J., Malmberg L.P., Righini G., Stoneman S.A.T. Definition of terms for applications of respiratory sounds. Eur Respir Rev 2000; 10(77): 597–610.
- Furman Е.G., Malinin S.V., Furman G., Meerovich V., Sokolovsky V., Rocheva Е. Respiratory sound analysis for bronchial asthma diagnostics. IOSR J Eng 2020; 10(1): 53–59.
- Holmes M.S., D’arcy S., Costello R.W., Reilly R.B. Acoustic analysis of inhaler sounds community-dwellin asthmatic patients for automatic assessment of adherence. IEEE J Transl Eng Health Med 2014; 2: 2700210, https://doi.org/10.1109/jtehm.2014.2310480.
- Oud M., Dooijes E.H., van der Zee J.S. Asthmatic airways obstruction assessment based on detailed analysis of respiratory sound spectra. IEEE Trans Biomed Eng 2000; 47(11): 1450–1455, https://doi.org/10.1109/10.880096.
- Tomita K., Nagao R., Touge H., Ikeuchi T., Sano H., Yamasaki A., Tohda Y. Deep learning facilitates the diagnosis of adult asthma. Allergol Int 2019; 68(4): 456–461, https://doi.org/10.1016/j.alit.2019.04.010.
- Шахгельдян К.И., Гельцер Б.И., Курпатов И.Г. Методы машинного обучения для дифференциальной диагностики болезней органов дыхания. Цифровое здравоохранение. Труды XX Международного конгресса «Информационные технологии в медицине». М; 2019; с. 10–13.
- Robles-Rubio C.A., Bertolizio G., Brown K.A., Kearney R.E. Scoring tools for the analysis of infant respiratory inductive plethysmography signals. PLoS One 2015; 10(7): e0134182, https://doi.org/10.1371/journal.pone.0134182.
- Azar A.T. Fast neural network learning algorithms for medical applications. Neural Comput Appl 2013; 23: 1019–1034, https://doi.org/10.1007/s00521-012-1026-y.
- Dwivedi A.K. Artificial neural network model for effective cancer classification using microarray gene expression data. Neural Comput Appl 2018; 29: 1545–1554, https://doi.org/10.1007/s00521-016-2701-1.
- Gupta N., Gupta D., Khanna A., Filho P.P.R., de Albuquerque V.H.C. Evolutionary algorithms for automatic lung disease detection. Measurement 2019; 140: 590–608, https://doi.org/10.1016/j.measurement.2019.02.042.
- Swaminathan S., Qirko K., Smith T., Corcoran E., Wysham N.G., Bazaz G., Kappel G., Gerber A.N. A machine learning approach to triaging patients with chronic obstructive pulmonary disease. PLoS One 2017; 12(11): e0188532, https://doi.org/10.1371/journal.pone.0188532.
- Порева А.С., Вайтышин В.И., Карплюк Е.С. Методы машинного обучения для исследования звуков легких. MicrosystElectronAcoust 2017; 22(6): 41–47, https://doi.org/10.20535/2523-4455.2017.22.6.108829.
- Yadav A., Dutta M.K., Prinosil J. Machine learning based automatic classification of respiratory signals using wavelet transform. In: 2020 43rd International Conference on Telecommunications and Signal Processing (TSP). Milan; 2020; p. 545–549, https://doi.org/10.1109/tsp49548.2020.9163565.
- Spathis D., Vlamos P. Diagnosing asthma and chronic obstructive pulmonary disease with machine learning. Health Informatics J 2019; 25(3): 811–827, https://doi.org/10.1177/1460458217723169.
- Messinger A.I., Bui N., Wagner B.D., Szefler S.J., Vu T., Deterding R.R. Novel pediatric-automated respiratory score using physiologic data and machine learning in asthma. Pediatr Pulmonol 2019; 54(8): 1149–1155, https://doi.org/10.1002/ppul.24342.
- Elmarakeby A.H., Hwang J., Arefeh R., Crowdis J., Gang S., Liu D., AlDubayan S.H., Salari K., Kregel S., Richter C., Arnoff T.E., Park J., Hahn W.C., Van Allen E.M. Biologically informed deep neural network for prostate cancer discovery. Nature 2021; 598: 348–352, https://doi.org/10.1038/s41586-021-03922-4.
- Shrikumar A., Greeside P., Kundaje A. Learning important features through propagating activation differences. Proc Mach Learn Res 2017; 70: 3145–3153, https://doi.org/10.48550/arxiv.1704.02685.
- Murdoch W.J., Singh C., Kumbier K., Abbasi-Asl R., Yu B. Definitions, methods, and applications in interpretable machine learning. Proc Natl Acad Sci USA; 2019; 116(44): 22071–22080, https://doi.org/10.1073/pnas.1900654116.
- Российское Респираторное Общество. Педиатрическое Респираторное Общество. Федерация педиатров стран СНГ. Национальная программа «Бронхиальная астма у детей. Стратегия лечения и профилактика». М; 2012.
- Бронхиальная астма. Клинические рекомендации. Под редакцией Чучалина А.Г. М; 2021; 118 с.
- Hastie T., Tibshirani R., Friedman J.H. The elements of statistical learning: data mining, inference, and prediction. Second edition. New York: Springer; 2009.
- Kiang M.Y. A comparative assessment of classification methods. Decis Support Syst 2003; 35(4): 441–454, https://doi.org/10.1016/s0167-9236(02)00110-0.
- Sharma G., Umapathy K., Krishnan S. Trends in audio signal feature extraction methods. Appl Acoust 2020; 158: 107020, https://doi.org/10.1016/j.apacoust.2019.107020.
- Boulesteix A.L., Janitza S., Kruppa J., König I.R. Overview of random forest methodology and practical guidance with emphasis on computational biology and bioinformatics. WIREs Data Mining Knowl Discov 2012; 2(6): 493–507, https://doi.org/10.1002/widm.1072.
- Rokach L., Maimon O. Decision trees. In: Data mining and knowledge discovery handbook. Boston, MA: Springer; 2005; p. 165–192, https://doi.org/10.1007/0-387-25465-x_9.
- Du K.L. Clustering: a neural network approach. Neural Netw 2010; 23(1): 89–107, https://doi.org/10.1016/j.neunet.2009.08.007.
- Nitze I., Schulthess U., Asche H. Comparison of machine learning algorithms random forest, artificial neural network and support vector machine to maximum likelihood for supervised crop type classification. In: Proceedings of the 4th GEOBIA. Rio de Janeiro; 2012; p. 35.
- Buccio E.D., Li Q., Melucci M., Tiwari P. Binary classification model inspired from quantum detection theory. In: Proceedings of the ACM SIGIR International Conference on Theory of Information Retrieval (ICTIR). New York; 2018; p. 187–190.
- Doshi К. Audio deep learning made simple: sound classification, step-by-step. Mar 18, 2021. URL: https://towardsdatascience.com/audio-deep- learning-made-simple-sound-classification- step-by-step-cebc936bbe5.
- van de Berge M., ten Hacken N.H.T., van der Wiel E., Postma D.S. Treatment of the bronchial tree from beginning to end: targeting small airway inflammation in asthma. Allergy 2013; 68(1): 16–26, https://doi.org/10.1111/all.12062.
- Фурман Е.Г., Корюкина И.П. Бронхиальная астма у детей: маркеры воспаления и состояние функции внешнего дыхания. Пермь; 2010; 183 с.
- Овсянников Д.Ю., Фурман Е.Г., Елисеева Т.И. Бронхиальная астма у детей. М: Российский университет дружбы народов; 2019; 211 с.
- Schneider A., Gindner L., Tilemann L., Schermer T., Dinant G.J., Joachim Meyer F., Szecsenyi J. Diagnostic accuracy of spirometry in primary care. BMC Pulm Med 2009; 9: 31, https://doi.org/10.1186/1471-2466-9-31.
- Valadares M.A., Santos I.N., Melo E.V., da Silva Â.M., Archanjo P.T., Nepomusceno E.C., Porto R.L., Gurgel R.Q., Brito L.S., Almeida M.L.D. Spirometry with FEV0.75 increases the sensitivity for the diagnosis of obstructive disorder in children of asthmatic mothers. J Aller Ther 2013; S2: 6, https://doi.org/10.4172/2155-6121.s2-006.
- Künzli N., Stutz E.Z., Perruchoud A.P., Brändli O., Tschopp J.M., Bolognini G., Karrer W., Schindler C., Ackermann-Liebrich U., Leuenberger P. Peak flow variability in the SAPALDIA study and its validity in screening for asthma-related conditions. The SPALDIA Team. Am J Respir Crit Care Med 1999; 160(2): 427–434, https://doi.org/10.1164/ajrccm.160.2.9807008.