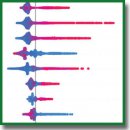
Performance of the Models Predicting In-Hospital Mortality in Patients with ST-Segment Elevation Myocardial Infarction with Predictors in Categorical and Continuous Forms
The aim of the study is to assess the performance of predictive models developed on the basis of predictors in the continuous and categorical forms to predict the probability of in-hospital mortality (IHM) in patients with ST-segment elevation myocardial infarction (STEMI) after percutaneous coronary intervention (PCI).
Materials and Methods. A single-center retrospective study has been conducted, within the framework of which data from 4674 medical records of patients with STEMI after PCI, treated at the Regional Vascular Center of Vladivostok (Russia), have been analyzed. Two groups of patients were identified: group 1 consisted of 318 (6.8%) individuals who died in the hospital, group 2 included 4356 (93.2%) patients with a favorable outcome of treatment. IHM prognostic models were developed using multivariate logistic regression (MLR), random forest (RF), and stochastic gradient boosting (SGB). 6-metric qualities were used to evaluate the accuracy of the models. Threshold values of IHM predictors were determined using a grid search to find the optimal cut-off points, calculating centroids, and Shapley additive explanations. The latter helped evaluate the degree to which the model predictors influence the endpoint.
Results. Based on the results of the multi-stage analysis of indicators of clinical and functional status of the STEMI patients, new predictors of IHM have been identified and validated, complementing the factors of the GRACE scoring system, their categorization has been carried out and prognostic models with continuous and categorical variables based on MLR, RF, and SGB have been developed. These models had a high (AUC — 0.88 to 0.90) and comparable predictive accuracy, but their predictors differed in various degrees of influence on the endpoint. The comparative analysis has shown that the Shapley additive explanation method has advantages in categorizing predictors compared to other methods and allows for detailing the structure of their relationships with IHM.
Conclusion. The use of modern data mining methods, including machine learning algorithms, categorization of predictors, and assessment of the degree of their effect on the endpoint, makes it possible to develop predictive models possessing high accuracy and the properties of explanation of the generated conclusions.
- Ibanez B., James S., Agewall S., Antunes M.J., Bucciarelli-Ducci C., Bueno H., Caforio A.L.P., Crea F., Goudevenos J.A., Halvorsen S., Hindricks G., Kastrati A., Lenzen M.J., Prescott E., Roffi M., Valgimigli M., Varenhorst C., Vranckx P., Widimský P.; ESC Scientific Document Group. 2017 ESC Guidelines for the management of acute myocardial infarction in patients presenting with ST-segment elevation: The Task Force for the management of acute myocardial infarction in patients presenting with ST-segment elevation of the European Society of Cardiology (ESC). Eur Heart J 2018; 39(2): 119–177, https://doi.org/10.1093/eurheartj/ehx393.
- Pfuntner A., Wier L.M., Stocks C. Most frequent procedures performed in U.S. hospitals, 2011. In: Healthcare Cost and Utilization Project (HCUP) Statistical Briefs. Rockville (MD): Agency for Healthcare Research and Quality (US); 2014.
- Wang J.J., Fan Y., Zhu Y., Zhang J.D., Zhang S.M., Wan Z.F., Su H.L., Jiang N. Biomarkers enhance the long-term predictive ability of the KAMIR risk score in Chinese patients with ST-elevation myocardial infarction. Chin Med J (Engl) 2019; 132(1): 30–41, https://doi.org/10.1097/cm9.0000000000000015.
- Liu X.J., Wan Z.F., Zhao N., Zhang Y.P., Mi L., Wang X.H., Zhou D., Wu Y., Yuan Z.Y. Adjustment of the GRACE score by HemoglobinA1c enables a more accurate prediction of long-term major adverse cardiac events in acute coronary syndrome without diabetes undergoing percutaneous coronary intervention. Cardiovasc Diabetol 2015; 14: 110, https://doi.org/10.1186/s12933-015-0274-4.
- Chen X., Shao M., Zhang T., Zhang W., Meng Y., Zhang H., Hai H., Li G. Prognostic value of the combination of GRACE risk score and mean platelet volume to lymphocyte count ratio in patients with ST-segment elevation myocardial infarction after percutaneous coronary intervention. Exp Ther Med 2020; 19(6): 3664–3674, https://doi.org/10.3892/etm.2020.8626.
- Wenzl F.A., Kraler S., Ambler G., Weston C., Herzog S.A., Räber L., Muller O., Camici G.G., Roffi M., Rickli H., Fox K.A.A., de Belder M., Radovanovic D., Deanfield J., Lüscher T.F. Sex-specific evaluation and redevelopment of the GRACE score in non-ST-segment elevation acute coronary syndromes in populations from the UK and Switzerland: a multinational analysis with external cohort validation. Lancet 2022; 400(10354): 744–756, https://doi.org/10.1016/s0140-6736(22)01483-0.
- Li R., Shen L., Ma W., Yan B., Chen W., Zhu J., Li L., Yuan J., Pan C. Use of machine learning models to predict in-hospital mortality in patients with acute coronary syndrome. Clin Cardiol 2023; 46(2): 184–194, https://doi.org/10.1002/clc.23957.
- Zack C.J., Senecal C., Kinar Y., Metzger Y., Bar-Sinai Y., Widmer R.J., Lennon R., Singh M., Bell M.R., Lerman A., Gulati R. Leveraging machine learning techniques to forecast patient prognosis after percutaneous coronary intervention. JACC Cardiovasc Interv 2019; 12(14): 1304–1311, https://doi.org/10.1016/j.jcin.2019.02.035.
- Du X., Wang H., Wang S., He Y., Zheng J., Zhang H., Hao Z., Chen Y., Xu Z., Lu Z. Machine learning model for predicting risk of in-hospital mortality after surgery in congenital heart disease patients. Rev Cardiovasc Med 2022; 23(11): 376, https://doi.org/10.31083/j.rcm2311376.
- Zhao P., Liu C., Zhang C., Hou Y., Zhang X., Zhao J., Sun G., Zhou J. Using machine learning to predict the in-hospital mortality in women with ST-segment elevation myocardial infarction. Rev Cardiovasc Med 2023; 24(5): 126, https://doi.org/10.31083/j.rcm2405126.
- MacCallum R.C., Zhang S., Preacher K.J., Rucker D.D. On the practice of dichotomization of quantitative variables. Psychol Meth 2002; 7(1): 19–40, https://doi.org/10.1037/1082-989x.7.1.19.
- Gupta R., Day C.N., Tobin W.O., Crowson C.S. Understanding the effect of categorization of a continuous predictor with application to neuro-oncology. Neurooncol Pract 2021; 9(2): 87–90, https://doi.org/10.1093/nop/npab049.
- Geltser B.I., Shakhgeldyan K.I., Rublev V.Yu., Domzhalov I.G., Tsivanyuk M.M., Shekunova O.I. Phenotyping of risk factors and prediction of inhospital mortality in patients with coronary artery disease after coronary artery bypass grafting based on explainable artificial intelligence methods. Rossijskij kardiologiceskij zurnal 2023; 28(4): 5302, https://doi.org/10.15829/1560-4071-2023-5302.
- Dawson N.V., Weiss R. Dichotomizing continuous variables in statistical analysis: a practice to avoid. Med Decis Making 2012; 32(2): 225–226, https://doi.org/10.1177/0272989x12437605.
- Salis Z., Gallego B., Sainsbury A. Researchers in rheumatology should avoid categorization of continuous predictor variables. BMC Med Res Methodol 2023; 23(1): 104, https://doi.org/10.1186/s12874-023-01926-4.
- Altman D.G., Royston P. The cost of dichotomising continuous variables. BMJ 2006; 332(7549): 1080, https://doi.org/10.1136/bmj.332.7549.1080.
- Austin P.C., Brunner L.J. Inflation of the type I error rate when a continuous confounding variable is categorized in logistic regression analyses. Stat Med 2004; 23(7): 1159–1178, https://doi.org/10.1002/sim.1687.
- Streiner D.L. Breaking up is hard to do: the heartbreak of dichotomizing continuous data. Can J Psychiatr 2002; 47(3): 262–266, https://doi.org/10.1177/070674370204700307.
- von Elm E., Altman D.G., Egger M., Pocock S.J., Gøtzsche P.C., Vandenbroucke J.P.; STROBE Initiative. The Strengthening the Reporting of Observational Studies Epidemiology (STROBE) statement: guidelines for reporting observational studies. Lancet 2007; 370(9596): 1453–1457, https://doi.org/10.1016/s0140-6736(07)61602-x.
- Gel’tser B.I., Shakhgel’dyan K.I., Domzhalov I.G., Kuksin N.S., Kokarev E.A., Pak R.L. Prognosticheskaya otsenka kliniko-funktsional’nogo statusa patsientov s infarktom miokarda s pod,,emom segmenta ST posle chreskozhnogo koronarnogo vmeshatel’stva. Svidetel’stvo o registratsii bazy dannykh 2023622740, 10.08.2023. Zayavka No.2023622516 ot 28.07.2023 [Prognostic assessment of the clinical and functional status of patients with ST-segment elevation myocardial infarction after percutaneous coronary intervention. Database registration certificate 2023622740, October 8, 2023. Application No.2023622516 dated August 28, 2023].
- Valente F., Henriques J., Paredes S., Rocha T., de Carvalho P., Morais J. A new approach for interpretability and reliability in clinical risk prediction: acute coronary syndrome scenario. Artif Intell Med 2021; 117: 102113, https://doi.org/10.1016/j.artmed.2021.102113.
- Lundberg S.M., Lee S.I. A unified approach to interpreting model predictions. In: Advances in neural information processing systems. arXiv; 2017, https://doi.org/10.48550/arxiv.1705.07874.
- Geltser B.I., Shahgeldyan K.I., Domzhalov I.G., Kuksin N.S., Kokarev E.A., Kotelnikov V.N., Rublev V.Yu. Prediction of in-hospital mortality in patients with ST-segment elevation acute myocardial infarction after percutaneous coronary intervention. Rossijskij kardiologiceskij zurnal 2023; 28(6): 5414, https://doi.org/10.15829/1560-4071-2023-5414.
- Molnar C. Interpretable machine learning. A guide for making black box models explainable. 2023. URL: https://christophm.github.io/interpretable-ml-book/.
- Mabikwa O.V., Greenwood D.C., Baxter P.D., Fleming S.J. Assessing the reporting of categorised quantitative variables in observational epidemiological studies. BMC Health Serv Res 2017; 17(1): 201, https://doi.org/10.1186/s12913-017-2137-z.
- Turner E.L., Dobson J.E., Pocock S.J. Categorisation of continuous risk factors in epidemiological publications: a survey of current practice. Epidemiol Perspect Innov 2010; 7: 9, https://doi.org/10.1186/1742-5573-7-9.